By Fern Halper, VP Research, Advanced Analytics
There was a time when choosing a programming language for data analysis had essentially no choice at all. The tools were few and they were usually developed and maintained by individual corporations that, though they ensured a reliable level of quality, could sometimes be quite difficult to work with and slow to fix bugs or innovate with new features. The landscape has changed, though.
Thanks to the Web, the open source software development model has shown that it can produce robust, stable, mature products that enterprises can rely upon. Two such products are of special interest to data analysts: Python and R. Python is an interpreted, interactive, object-oriented scripting language created in 1991 and now available through the Python Foundation. R, which first appeared at roughly the same time, is a language and software environment for statistical computing and graphics that is supported by the R Foundation for Statistical Computing.
Each comes with a large and active community of innovative developers, and has enormous resources readily available through libraries for analytics and processing—libraries such as NumPy, SciPy, and Scikit-learn for data manipulation and analytics in Python, and purr, ggplot2, and rga in R. These features can go a long way to speeding time-to-value for today’s businesses.
Python pro Paul Boal and long-time TDWI instructor Deanne Larson (who will both be bringing their Quick Camps to Accelerate Seattle) both see the value in learning these tools.
“R is very popular with data scientists,” Larson says. “They’re using it for data analysis, extracting and transforming data, fitting models, drawing inferences, even plotting and reporting the results.”
Scaling to the Enterprise Level
Debraj GuhaThakurta, senior data and applied scientist at Microsoft, warns that “Although R has a large number of packages and functions for statistics and machine learning,” he says, “many data scientists and developers today do not have the familiarity or expertise to scale their R-based analytics or create predictive solutions in R within databases.” Work is needed, he says, to create and deploy predictive analytics solutions in distributed computing and database environments.
And although there are adjustments to be made at the enterprise level to integrate and scale the use of these technologies, the use cases continue to grow. According to Natasha Balac of Data Insight Discovery, Inc., the number of tools available for advanced analytics techniques such as machine learning has exploded to the level where help is needed to navigate the field. She chalks it up to increasingly affordable and scalable hardware. “It’s not limited to any one vertical either,” Balac says. “Users need help integrating their experience, goals, time lines, and budgets to sort out the optimal use cases for these tools—both for individuals and teams.”
There are significant resources available to companies and data professionals to close the gap between skilled users and implementation. TDWI has integrated new courses on machine learning, AI, and advanced analytics into events like TDWI Accelerate. The next event will be held in Seattle on October 16-18, check out the full agenda here.
Build your expertise. Drive your organization’s success. Advance your career. Join us at TDWI Accelerate, October 16-18 in Seattle, WA.
Posted on July 26, 20170 comments
By Fern Halper, TDWI Research Director for Advanced Analytics
Philip Russom, Dave Stodder, and I are in the process of putting together our most recent Best Practices Report: Emerging Technologies for Business Intelligence, Analytics, and Data Warehousing. TDWI refers to new and exciting technologies, vendor tools, team structures, development methods, user best practices, and new sources of big data as emerging technologies and methods (ETMs). For example, tools for data visualization are the most hotly adopted ETM in BI in recent years. In addition to visualization, most of these tools also support other emerging techniques, namely data exploration and discovery, data preparation, analytics, and storytelling. ETMs for analytics involve advanced techniques, including predictive analytics, stream mining, and text analytics, that are progressively applied to emerging data sources, namely social media data, machine data, cloud-generated data, and the Internet of things. A number of emerging data platforms have entered data warehouse (DW) environments, including Hadoop, MapReduce, columnar database management systems (DBMSs), and real-time platforms for event and stream data. The most influential emerging methods are based on agile development or collaborative team structures (e.g., competency centers).
ETMs assist with competitiveness, decisions, business change, and innovation. According to this report’s survey, the leading general benefits of ETMs (in survey order) are improvements in competitiveness, decision making, responses to business change, business performance, and innovation. These benefits are being realized today, because two-thirds of organizations surveyed are already using ETMs and 79 percent consider ETMs an opportunity.
Despite the benefits, a number of barriers stand in the way of adopting ETMs. Many people feel held back by their IT team’s lack of skills, staffing, infrastructure, and buy-in. Others have trouble seeing the business value of leading-edge technologies. Some work in risk-averse organizations that lack a culture of innovation for either IT or the business. Nonetheless, both business and technical respondents report working through these issues to adopt ETMs.
Some ETMs are more like tool features that are emerging in a variety of tool types. The most pervasive is self-service functionality, which is found in tools for reporting, analytics, data prep, and so on. The point is to give certain classes of users tools that are simple, intuitive, and integrated with common data sources, requiring little-to-no setup or assistance from IT. Fifty-four percent of users surveyed consider themselves successful with IT-free self-service.
Open source software (OSS) has become an important wellspring for innovation. Hadoop (whether from Apache or a software vendor), tools associated with it (MapReduce, Spark, Hive, HBase), and other similar data platforms (NoSQL databases) have emerged from their Internet-company roots and are now being adopted by mainstream enterprises. These ETMs are examples of how influential OSS has become for innovative products. Interfaces to these platforms’ data are also common emerging features in vendor-supplied tools for data integration, data prep, data exploration, reporting, and analytics.
DW environments presently include multiple ETMs, many based on open source. All these OSS-based or OSS-inspired ETMs are now entering DW environments, along with slightly older ETMs like DW appliances, analytics DBMSs, and columnar DBMSs. This emergence has driven a trend toward multi-platform DW environments, where the core relational warehouse is joined by a long list of standalone data platforms, most of them ETMs.
Posted by Fern Halper, Ph.D. on July 30, 20150 comments
By Fern Halper, TDWI Research Director for Advanced Analytics
What does it take to achieve analytics maturity? Earlier this week, Dave Stodder and I hosted a webcast with a panel of vendor experts from Cloudera, Microstrategy, and Tableau. These three companies are all sponsors of the Analytics Maturity Model, an analytics assessment tool that measures where your organization stands relative to its peers in terms of analytics maturity.
There were many good points made during the discussion. A few particularly caught my attention, because they focused on the organizational aspects of analytics maturity, which are often the most daunting.
Crawl, walk, run: TJ Laher, from Cloudera, pointed out that their customers often crawl, walk, and then run to analytics. I’ve said before that there is no silver bullet for analytics. TJ stressed the need for organizations to have a clear vision of strategic objectives and to start off with some early projects that might take place over a six-month time frame. He spoke about going deep with the use cases that you have and then becoming more advanced, such as in bringing in new data types. Cloudera has observed that success in these early projects often helps to facilitate the walking and then ultimately the running (i.e., becoming more sophisticated) with analytics.
Short-term victories have long-term implications: Vijay Anand from MicroStrategy also touched upon the idea of early wins and pointed out that these can have long-term implications. He pointed out that it is important to think about these early victories in terms of what is down the road. For instance, say the business implements a quick BI solution. That’s great. However, business and IT need to work together to build a certified environment to avoid conflicting and non-standardized information. It is important to think it through.
IT builds the car and business drives it. Ian Coe, from Tableau, also talked about IT and the business working together. He said that organizations achieve success and become mature when teams work together collaboratively on a number of prototypes using an agile approach. Tableau believes that the ideal model for empowering users involves a self-service BI approach. Business people are responsible for doing analysis. IT is responsible for managing and securing data. This elevates IT from the role of dashboard factory to architect and steward of the company’s assets. IT can work in quick cycles to give business what they need and check in with business regularly.
Of course, each expert came to the discussion table with their own point of view. And, these are just some of the insights that the panel provided. The Webinar is available on demand at tdwi.org. I encourage you to listen to it and, of course, take the assessment!
Posted by Fern Halper, Ph.D. on February 6, 20150 comments
I recently completed TDWI’s latest Best Practices Report: Next Generation Analytics and Platforms for Business Success. Although the phrase "next-generation analytics and platforms" can evoke images of machine learning, big data, Hadoop, and the Internet of things (IoT), most organizations are somewhere in between the technology vision and today’s reality of BI and dashboards. For some organizations, next generation can simply mean pushing past reports and dashboards to more advanced forms, such as predictive analytics. Next-generation analytics might move your organization from visualization to big data visualization; from slicing and dicing data to predictive analytics; or to using more than just structured data for analysis. The market is on the cusp of moving forward.
What are some of the newer next-generation steps that companies are taking to move ahead?
- Moving to predictive analytics. Predictive analytics is a statistical or data mining technique that can be used on both structured and unstructured data to determine outcomes such as whether a customer will "leave or stay" or "buy or not buy." Predictive analytics models provide probabilities of certain outcomes. Popular use cases include churn analysis, fraud analysis, and predictive maintenance. Predictive analytics is gaining momentum and the market is primed for growth, if users stick to their plans and if they can be successful with the technology. In this case, 39% of respondents stated they are using predictive analytics today, and an additional 46% are planning to use it in the next few years . Often organizations move in fits and starts when it comes to more advanced analytics, but predictive analytics along with other techniques such as geospatial analytics, text analytics, social media analytics, and stream mining are gaining interest in the market.
- Adding disparate data to the mix. Currently, 94% of respondents stated they are using structured data for analytics, and 68% are enriching this structured data with demographic data for analysis. However, companies are also getting interested in other kinds of data. Sources such as internal text data (today 27%), external Web data (today 29%), and external social media data (today 19%) are set to double or even triple in use for analysis over the next three years. Likewise, while IoT data is used by fewer than 20% of respondents today, another 34% are expecting to use it in the next three years. Real-time streaming data, which goes hand in hand with IoT data, is also set to grow in use (today 18%).
- Operationalizing and embedding analytics. Operationalizing refers to making analytics part of a business process; i.e., deploying analytics into production. In this way, the output of analytics can be acted upon. Operationalizing occurs in different ways. It may be as simple as manually routing all claims that seem to have a high probability of fraud to a special investigation unit, or it might be as complex as embedding analytics in a system that automatically takes action based on the analytics. The market is still relatively new to this concept. Twenty-five percent have not operationalized their analytics, and another 15% stated they operationalize using manual approaches. Less than 10% embed analytics in system processes to operationalize it.
- Investing in skills. Respondents cited the lack of skilled personnel as a top challenge for next-generation analytics. To overcome this challenge, some respondents talked about hiring fewer but more skilled personnel such as data analysts and data scientists. Others talked about training from within because current employees understand the business. Our survey revealed that many organizations are doing both. Additionally, some organizations are building competency centers where they can train from within. Where funding is limited, organizations are engaging in self-study.
These are only a few of the findings in this Best Practices Report.
Download the Report
Posted by Fern Halper, Ph.D. on December 18, 20140 comments
Analytics is hot—many organizations realize that it can provide an important competitive advantage. If your company wants to build an “analytics culture” where data analysis plays an essential role, your first step is to determine the maturity of your organization's analytics. To help your organizations measure their progress in their analytics efforts, we recently developed the TDWI Analytics Maturity Model and Assessment, which provides a quick way for you to compare your progress to other companies.
Take the assessment and you’ll get the big picture of your current analytics program, where it needs to go, and where to concentrate your efforts to achieve your goals and gain value from your analytics. Download the guide to help analyze your scores.
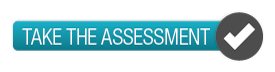
So, why a maturity model for analytics?
- It provides a framework.
Numerous studies indicate acting on analytics has a top- and bottom-line impact. Some companies don’t know where to start. Others don’t know what to do next.
Our analytics model provides a framework across five dimensions that are critical for analytics deployments: organization, infrastructure, data management, analytics, and governance.
The model helps create structure around an analytics program and determine where to start. It also helps identify and define the organization’s goals around the program and creates a process to communicate that vision across the entire organization.
- It provides guidance.
The model can provide guidance for companies at any stage of their analytics journey by helping them understand best practices used by companies more mature in their deployments.
A maturity model provides a methodology to measure and monitor the state of the program and the effort needed to complete the current stage, as well as steps to move to the next stage of maturity. It serves as a kind of odometer to measure and manage the speed of your progress and adoption within the company for an analytics program.
- It provides a benchmark.
Organizations want to understand how their analytics deployments compare to those of their peers so they can provide best-in-class insight and support.
We have created an online assessment that consists of 35 questions across the dimensions mentioned above. At the end of the assessment, you will get a score that lets you know how mature your current analytics deployment is. You will also receive your score in each of the dimensions, along with the average scores of others in your industry or company size. This is a great way to benchmark your analytics progress!
We invite you to read the benchmark guide and take the assessment!
Posted by Fern Halper, Ph.D. on November 6, 20140 comments
Almost a year has passed since the launch of the TDWI Big Data Maturity Model and assessment tool, which I co-authored with Krish Krishnan. To date, more than 600 respondents have participated in the assessment.
We asked questions in five categories relevant to big data:
- Organization: To what extent does your organizational strategy, culture, leadership, and funding support a successful big data program? What value does your company place in analytics?
- Infrastructure: How advanced and coherent is your architecture in support of a big data initiative? To what extent does your infrastructure support all parts of the company and potential users? How effective is your big data development approach? What technologies are in place to support a big data initiative, and how are they integrated into your existing environment?
- Data Management: How extensive is the variety, volume, and velocity of data used for big data analytics, and how does your company manage its big data in support of analytics? (This includes data quality and processing as well as data integration and storage issues.)
- Analytics: How advanced is your company in its use of big data analytics? (This includes the kinds of analytics utilized, how the analytics are delivered in the organization, and the skills to make analytics happen.)
- Governance: How coherent is your company’s data governance strategy in support of its big data analytics program?
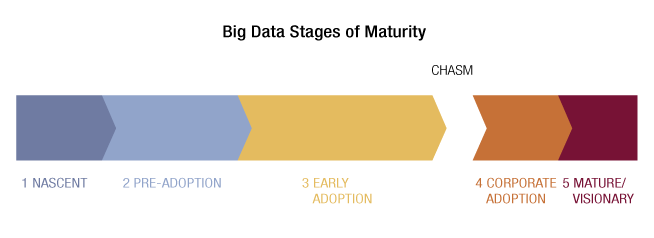
Respondents answered 75 questions across these categories and were given a score for each category. Scores correlated with stages of maturity, including nascent, pre-adoption, early adoption, corporate adoption, and mature/visionary. (Get more information about the Big Data Maturity Model by downloading the guide.)
So what are we seeing? Where are organizations in terms of big data maturity?
In a word: early—at least for organizations that took this assessment. The majority self-reported that they had nothing in place for big data or were just in the experimentation phase.
Only a small percentage of respondents are organized to execute on big data initiatives.
When we averaged scores across all five dimensions, the mean scores put respondents between the pre-adoption and early adoption stages—when organizations are thinking about big data and may have some proof of concepts going.
However, here are three results worth noting:
- Respondents are not organized to execute. Only a small percentage of respondents are organized to execute on big data initiatives. About 25% of respondents had a road map or a strategy in place for big data. In addition, about one-quarter had some sort of funding process in place for dealing with big data projects. Although organizations scored higher on "soft" questions such as whether they thought they had an analytics culture in place, scores still put many in the pre-adoption phase.
- The data warehouse is cited most often as the infrastructure for big data. We asked respondents what kind of infrastructure they had in place for big data. A sign of maturity for big data is to take a hybrid ecosystem approach. In other words, organizations often have a data warehouse (or marts) in place, but supplement it with other tools for other jobs. Hadoop or an analytics platform might work in conjunction with the warehouse, for instance. Some organizations might use some infrastructure in the cloud. About one-third of respondents stated that their data warehouse was their core technology for big data. Another one-third stated they didn’t have a big data infrastructure. The rest had some combination of technologies in place, but they were often siloed.
- More advanced analytics are happening on big data, but in pockets. On the analytics front, organizations were often collecting data they weren’t analyzing. About half of the respondents stated that they were performing advanced analytics (i.e., predictive analytics or other advanced techniques), but it was happening in pockets. It was also a bit unclear whether they were analyzing big data as part of their advanced analytics efforts. Many respondents were still trying to put their big data teams together. Few had a center of excellence (COE), where ideas are shared, governance exists, and training takes place.
I’ll continue to share interesting and notable results from the Big Data Maturity Model Assessment. In the meantime, if you haven’t taken the assessment, I encourage you check it out here.

What’s next? TDWI set to launch new analytics maturity model in late 2014
TDWI is excited to announce our plans to launch a new assessment, which we're calling the Analytics Maturity Model. Like the Big Data Maturity Model, this model has an assessment associated with it. Unlike the Big Data Maturity Model, this model focuses on analytics maturity across the spectrum of BI and analytics techniques and infrastructures. I’ll be writing more about this maturity model in the coming weeks. Stay tuned!
Posted by Fern Halper, Ph.D. on October 16, 20140 comments