By Fern Halper, TDWI Research Director for Advanced Analytics
Philip Russom, Dave Stodder, and I are in the process of putting together our most recent Best Practices Report: Emerging Technologies for Business Intelligence, Analytics, and Data Warehousing. TDWI refers to new and exciting technologies, vendor tools, team structures, development methods, user best practices, and new sources of big data as emerging technologies and methods (ETMs). For example, tools for data visualization are the most hotly adopted ETM in BI in recent years. In addition to visualization, most of these tools also support other emerging techniques, namely data exploration and discovery, data preparation, analytics, and storytelling. ETMs for analytics involve advanced techniques, including predictive analytics, stream mining, and text analytics, that are progressively applied to emerging data sources, namely social media data, machine data, cloud-generated data, and the Internet of things. A number of emerging data platforms have entered data warehouse (DW) environments, including Hadoop, MapReduce, columnar database management systems (DBMSs), and real-time platforms for event and stream data. The most influential emerging methods are based on agile development or collaborative team structures (e.g., competency centers).
ETMs assist with competitiveness, decisions, business change, and innovation. According to this report’s survey, the leading general benefits of ETMs (in survey order) are improvements in competitiveness, decision making, responses to business change, business performance, and innovation. These benefits are being realized today, because two-thirds of organizations surveyed are already using ETMs and 79 percent consider ETMs an opportunity.
Despite the benefits, a number of barriers stand in the way of adopting ETMs. Many people feel held back by their IT team’s lack of skills, staffing, infrastructure, and buy-in. Others have trouble seeing the business value of leading-edge technologies. Some work in risk-averse organizations that lack a culture of innovation for either IT or the business. Nonetheless, both business and technical respondents report working through these issues to adopt ETMs.
Some ETMs are more like tool features that are emerging in a variety of tool types. The most pervasive is self-service functionality, which is found in tools for reporting, analytics, data prep, and so on. The point is to give certain classes of users tools that are simple, intuitive, and integrated with common data sources, requiring little-to-no setup or assistance from IT. Fifty-four percent of users surveyed consider themselves successful with IT-free self-service.
Open source software (OSS) has become an important wellspring for innovation. Hadoop (whether from Apache or a software vendor), tools associated with it (MapReduce, Spark, Hive, HBase), and other similar data platforms (NoSQL databases) have emerged from their Internet-company roots and are now being adopted by mainstream enterprises. These ETMs are examples of how influential OSS has become for innovative products. Interfaces to these platforms’ data are also common emerging features in vendor-supplied tools for data integration, data prep, data exploration, reporting, and analytics.
DW environments presently include multiple ETMs, many based on open source. All these OSS-based or OSS-inspired ETMs are now entering DW environments, along with slightly older ETMs like DW appliances, analytics DBMSs, and columnar DBMSs. This emergence has driven a trend toward multi-platform DW environments, where the core relational warehouse is joined by a long list of standalone data platforms, most of them ETMs.
Posted by Fern Halper, Ph.D. on July 30, 20150 comments
By David Stodder, TDWI Director of Research for Business Intelligence
We are past the half-way point of 2015. Major League Baseball is celebrating its all-stars in Cincinnati as teams contemplate trades that they hope will make them stronger for the second-half run. Meanwhile, fall sports are starting to stir; National Football League teams open their training camps around the end of the month. Even pumpkin farmers are aware of time passing; to have fully grown pumpkins for Halloween, they need to have their seeds planted by now. While the air is warm and the sun isstill high in the sky, it’s a good time to contemplate significant trends in our industry this year.
The top trend on my list would be the flourishing of Apache Spark, the open source parallel processing framework (or “engine”) for developing analytic applications and systems working with big data. If Spark “went supernova in 2014,” as Stephen Swoyer put it in a fine article earlier this year, the energy from its explosion is forcefully generating a lot of industry activity in 2015. And not just among the small, newer vendors: IBM, Intel, Microsoft, and other mainstream vendors have issued major Spark announcements and product releases already this year, with more to come. Describing Spark’s potential impact, IBM experts have called Spark “the next Linux.”
As I learned at Strata in February and even more at the Spark Summit in June, Spark is shaking up the big data realm, whichhasbeen dominated by Hadoop, MapReduce, Hive, and Storm technologies. While compatible with them, Sparkoffers performance and scalability advantages over these technologies, including through support for multi-step pipelines that reduce the wait for steps to complete, and support for in-memory data sharing.
One of Spark’s most important attributes is a unified approach tothe management and interaction with a greater diversity of data. The Spark framework can support not only batch processing a la Hadoop but also interactive SQL, real-time processing, machine learning, and stream analytics. At Strata, I met with Matei Zaharia, CTO of Databricks, which was founded by Zaharia and other members of the University of California, Berkeley’s AMPLab team that created Spark and launched it as an Apache project. He did not envision organizations being satisfied with putting all their data into massive Hadoop data lakes; he saw instead increasing diversity in data sources that users seek to access, which requires the unified framework and processing layer that Spark provides.
Spark has changed the parameters of the debate about how SQL-based business intelligence and visual analytics tools and application users might access big data. With Spark SQL, one of the four primary AMPLab-developed libraries that fit into the Spark framework, organizations could bypass some of the steps that have been necessary to move and transform Hadoop files into data warehouses before they can fully analyze the data. Application programming interfaces, such as SparkR for R language programming, are broadening the toolkit available for analytics.
Spark is not as mature as Hadoop or the SQL-on-Hadoop offerings in the market. Spark is also not the only “star” in the open source interactive analytic SQL query galaxy; Presto, which is now strongly backed by Teradata, is another interesting distributed SQL query engine to watch. All of these technologies are enabling organizations to do broader and deeper analytics with data and are becoming important parts of emerging diverse, “hybrid” data architectures (pardon a shameless plug: this topic will be covered at our Solution Summit in Scottsdale later this year).
Spark is a major trend in 2015. What are other trends you are seeing? I would be interested to hear your thoughts.
Hyperlinks embedded in this blog:
Apache Spark: https://spark.apache.org/
Swoyer article: http://tdwi.org/articles/2015/01/06/apache-spark-next-big-thing.aspx
IBM announcement: https://www-03.ibm.com/press/us/en/pressrelease/47107.wss
Intel: https://software.intel.com/sites/campaigns/sparks/IgnitingSparks.php
Microsoft: http://azure.microsoft.com/blog/2015/07/10/interactive-analytics-on-big-data-with-the-release-of-spark-for-azure-hdinsight/
“the next Linux”: https://youtu.be/CrGB_2GJ-fA
Strata: http://strataconf.com/
Spark Summit: https://spark-summit.org/
Databricks: http://www.databricks.com/
AMPLab: https://amplab.cs.berkeley.edu/
Presto: https://prestodb.io/
Teradata Presto announcement: http://www.teradata.com/News-Releases/2015/Teradata-Launches-First-Enterprise-Support-for-Presto/?LangType=1033&LangSelect=true
Posted by David Stodder on July 13, 20150 comments
Inspirational User Case Studies and Educational Product Demonstrations
By Philip Russom, TDWI Research Director for Data Management
When I attend a user group meeting or a vendor’s conference, my top two priorities are (1) to hear case studies from successful users and (2) to see practical demonstrations of the vendor’s products. I got both of those in spades last week, when I spent three days attending Informatica World 2015 in Las Vegas.
It was a huge conference, with about 2,500 people attending and five or more tracks running simultaneously. I couldn’t attend all these sessions, so I decided to focus on the keynotes and the Data Integration Track. To give you a taste of the conference, allow me to share highlights from what I was able to attend, with a stress on case studies and demos.
User Case Studies
An enterprise architect at MasterCard discussed their implementation of an enterprise data hub. The hub gives data analysts the data they need in a timely fashion, provides self-service data access for a variety of users, and serves as a unified platform for both internal and external data exchange.
Tom Tshontikidis explained why and how Kaiser Permanente migrated its large collection of data integration solutions from a legacy product (heavily extended via hand coding) to PowerCenter and other Informatica tools.
Two representatives from Cleveland Clinic spoke of their journey from quantity based metrics for performance management (which mostly laid blame on employees for missed targets) to quality based predictive analytics (which now sets realistic goals for helping their patients).
Dr. John Frenzel is the chief medical information officer at the MD Anderson Cancer Center. At Informatica World, he discussed how big data analytics is accelerating clinical research. Among the many great tips he shared, Frenzel described how data scientists at MD Anderson work like consultants, traveling among multiple teams, to share their expertise.
An IT systems architect at a major telecommunications company told the story about how they needed to simplify operations, so it could transform into better integrated – and hence more nimble – global organization. In support of those business goals, IT replaced hundreds of systems, mostly with six primary ones. This gargantuan consolidation project was mostly powered by Informatica tools.
Tom Kato of Mototak Consulting spoke in a few sessions. In one, he described how to manage data from cradle to grave, using best practices and leading tools for Information Lifecycle Management (ILM). In another, he explained his use of the Informatica Data Validation Option (DVO) in an early phase of the merger between American Airlines and US Airways.
John Racer from Discount Tire explained why validating data is important to assuring that data arrives where it’s supposed to be and in the condition intended. He discussed practical applications in cross-platform data flows, application migrations, and data migrations, involving tools from Informatica and other providers.
Product Demonstrations
Some of the coolest demos were presented by users. For example, I saw a management dashboard built by folks at a major energy company, using a visualization tool and data from PowerCenter. The dashboard enables business users to do pipeline capacity management and related operational tasks, many with near time data.
The Informatica Data Validation Option (DVO) kept coming up in presentations by both Informatica employees and customers. I was glad to see this, because I’ve long felt that data integration users do not validate data as often as they should. For example, validation should be part of most ETL testing and all data migration projects.
For a variety of reasons, I was glad see Secure@Source demo’d. The demo clarified that this is not a security tool, per se, although it can guide your security and other efforts. Instead, Secure@Source provides analytics for assessing data-oriented risks relevant to security, privacy, compliance, governance, and so on. Essentially, you create policies and other business rules (typically inspired by your compliance and governance policies), and Secure@Source helps you identify risks and quantify compliance.
Informatica’s Krupa Natarajan spent most of a session demonstrating Informatica Cloud. This product has been in production since 2006, so there’s a lot of robust functionality to look at. Long story short, Informatica Cloud comes across as a full-featured integration tool, not some after-thought hastily ported to a cloud (as too many cloud-based products are). Although Krupa didn’t say it explicitly, the demo brought home to me the point that data integration with a cloud-based tool is pretty much the same as with traditional tools. That good news should help users get more comfortable with clouds in general, as well as the potential use of cloud-based data management tools.
Further Learning
If you go to www.YouTube.com and search for “Informatica World 2015” you’ll find many useful speeches and sessions that you can replay. Here’s a couple of links to get you started:
Keynote by Informatica’s CEO, Sohaib Abbasi. This is a “must see,” if you care about Informatica’s vision for the future, especially in the context of the proposed acquisition of Informatica.
Interviews filmed on site by theCUBE. All the interviews are good. But I especially like the interviews with my analyst friends: John Myers and Mark Smith.
Posted by Philip Russom, Ph.D. on May 18, 20150 comments
Take a look at 5 new resources that can help you evolve your analytics strategies beyond spreadsheets and dashboards. Create more value from your data when you move beyond simple business intelligence (BI) reporting to data discovery and advanced analytics. Use these recently released resources to develop your competitive advantage.
1. |
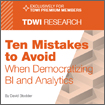 |
Ten Mistakes to Avoid When Democratizing BI and Analytics
Premium member resource—freely available until May 29
Download Now |
|
2. |
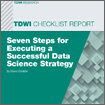 |
Seven Steps for Executing a Successful Data Science Strategy
Download Now |
|
3. |
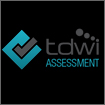 |
TDWI Analytics Maturity Model Assessment & Guide
Download Now |
|
4. |
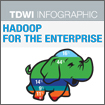 |
TDWI Infographic: Hadoop for the Enterprise
Download Now |
|
5. |
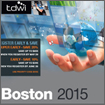 |
Upcoming Live Event: Special Offer Below
TDWI Boston 2015 | The Analytics Experience
July 26-31, 2015
Six action-packed days filled
with classes, case studies, and hands-on training (WebAction, Tableau, Luminoso, Yellowfin, Archipelago, Data Mining with R, Hadoop, and more) offer an accelerated learning experience for business and
technical leaders and implementers.
Learn More |
|
REGISTER NOW & SAVE BIG |
|
The Analytics Experience | Boston 2015
July 26–31, 2015
Sign up now for the SUPER EARLY registration discount
20% off until May 29—Save up to $855!
Use priority code SEB20
Learn More
|
|
Posted by TDWI on May 15, 20150 comments
By Philip Russom, Research Director for Data Management, TDWI
To help you better understand Hadoop’s evolution into mainstream enterprise usage—and why you should care—I’d like to share with you the series of 25 tweets I recently issued on the topic. I think you’ll find the tweets interesting, because they provide an overview of enterprise Hadoop and its best practices in a form that’s compact, yet amazingly comprehensive.
Every tweet I wrote was a short sound bite or stat bite drawn from my recent TDWI report
Hadoop for the Enterprise. Many of the tweets focus on a statistic cited in the report, while other tweets are definitions stated in the report.
I left in the arcane acronyms, abbreviations, and incomplete sentences typical of tweets, because I think that all of you already know them or can figure them out. Even so, I deleted a few tiny URLs, hashtags, and repetitive phrases. I issued the tweets in groups, on related topics; so I’ve added some headings to this blog to show that organization. Otherwise, these are raw tweets.
Introduction to Hadoop for the Enterprise
1. #Hadoop is expanding into more industries, use cases & enterprise breadth. More in #TDWI Webinar Apr. 14 Noon ET http://bit.ly/1F9d2iy
2. #Hadoop for the Enterprise tech drivers: scalability, low cost, & many data types.
3. #Hadoop for the Enterprise biz drivers: #analytics, data exploration, value from #BigData.
Hadoop Adoption is Up
4. #TDWI SURVEY SEZ: #Hadoop adoption accelerating. Production clusters up 60% in 2 yrs.
5. #TDWI SURVEY SEZ: Half of respondents have #Hadoop clusters in development, coming online in 12 months.
6. #TDWI SURVEY SEZ: 60% of users surveyed will have #Hadoop in production by 2016.
Benefits and Barriers
7. #TDWI SURVEY SEZ: 89% surveyed say #Hadoop is opportunity for biz/tech #innovation.
8. #TDWI SURVEY SEZ: #Hadoop’s benefits: improve #analytics, #EDW, scalability, exotic data.
9. #TDWI SURVEY SEZ: #Hadoop’s barriers: weak skills, biz case, security, open source tools.
Organizational Issues with Enterprise Hadoop
10. As #Hadoop goes enterprise scope, ownership, staffing, dev methods & economics shift.
11. #Hadoop clusters are becoming central, shared IT infrastructure in mainstream firms.
12. #TDWI SURVEY SEZ: Common #Hadoop job titles are: #DataScientist, architect, analyst, developer.
13. #TDWI SURVEY SEZ: Firms train employees in #Hadoop cuz they can’t find or afford folks to hire.
The Many Use Cases for Enterprise Hadoop
14. #TDWI SURVEY SEZ: Leading future #Hadoop uses: ent data hubs, archives, misc BI/DW.
15. #TDWI SURVEY SEZ: Half of respondents will add #DataQuality & #MDM for #Hadoop data.
16. #TDWI SURVEY SEZ: Established #Hadoop practice extends a #DataWarehouse (46%).
17. #TDWI SURVEY SEZ: Data lakes (36%) & enterprise data hubs (28%) are new practices for #Hadoop.
18. #TDWI SURVEY SEZ: Archiving on #Hadoop is upcoming for new (36%) & old (19%) data.
19. #TDWI SURVEY SEZ: #Hadoop for content mgt (17%) & operational ent apps (11%) are new.
Hadoop’s Roles in Enterprise Data Strategies and Architectures
20. #TDWI SURVEY SEZ: 66% feel #Hadoop is important to their enterprise data strategy.
21. #TDWI SURVEY SEZ: #Hadoop is becoming key to multi-platform #DataWarehouse environments (DWEs).
22. #TDWI SURVEY SEZ: a third of #Hadoop clusters are off premises, on cloud, SaaS, managed provider. Surprising!
Hadoop Development Details
23. #Hadoop cluster size scales down to dept use (8 nodes) or up to enterprise (1000 nodes).
24. #TDWI SURVEY SEZ: #Hadoop clusters per enterprise = 10 on average, with median at 4.
25. #TDWI SURVEY SEZ: 58% of #Hadoop dev done w/mix of hand-coding & hi-level tools. 23% coded only.
Want to learn more about Hadoop for the Enterprise?
For a more detailed discussion—in a traditional publication!—get the TDWI Best Practices Report
Hadoop for the Enterprise, which is available in a
PDF via a free download.
You can also register for and
replay my TDWI Webinar, where I present the findings of
Hadoop for the Enterprise.
Posted by Philip Russom, Ph.D. on April 27, 20150 comments
Attendees of a recent TDWI Webinar asked excellent questions.
By Philip Russom, TDWI Research Director for Data Management
Recently, on April 14, I broadcast a TDWI Webinar in which I presented some of the findings from my new TDWI report on "Hadoop for the Enterprise." You can download a free copy of the report in a PDF, and you can replay the Webinar. With each link, you may need to scroll down to find what you want. If you’re new to Hadoop, you may wish to first read the 2013 TDWI Best Practices Report Integrating Hadoop into Business Intelligence and Data Warehousing.
Attendees of the Webinar posed several very good questions about various issues around Hadoop. Please allow me to share a few attendee questions and the answers I sent them via e-mail:
What is a Hadoop cluster? And why would an organization need more than one?
The Wikipedia article on “Computer Cluster” is a good general description of all clustered server pools. The article doesn’t mention Hadoop, but Hadoop’s clustering strategy is in line with the article, except that Hadoop can run on heterogeneous servers, whereas the article recommends that all servers be identical. The point of any cluster is to get scalable and high-perfromance computational power, but at a relatively low cost because of commodity priced hardware.
An organization may need more than one Hadoop cluster, due to departmental funding and sponsorship (which is common with analytic applications) or other organizational dynamics. As I pointed out in the Webinar, as users decide on a strategy for Hadoop on an enterprise scale, they tend to abandon the departmental focus in favor of central IT providing Hadoop as a shared enterprise asset (as IT often does with corporate networks, racks of servers, and storage subsystems).
You don't need big data to take advantage of Hadoop?
That’s correct. I’ve found many user organizations with a small Hadoop implementation (8 nodes seems common) used as the data layer under a departmental analytic application or analytics sandbox of some sort. Hadoop makes sense when the department has exotic data (perhaps in lots of files), which Hadoop excels with. Use cases include sentiment analytics with schema-free human language text or supplier analytics with multi-structured XML or JSON files.
Note that, in the examples, the data volumes are modest, but it’s still “big data” in the sense that it’s not the usual structured and relational data. For many users dealing with big data (whether on Hadoop or elsewhere), the value proposition is that big data is new and different, and therefore offers new insights and more complete views of customers. Even when big data is truly big (tens of terabytes or more), users don’t have much trouble managing it; hence, big data is not a scalability crisis, as some people have claimed.
Hadoop has a well-deserved reputation for scaling up linearly. But these examples show that Hadoop also scales down successfully.
Do companies transfer master data into Hadoop to support analytics in a real-time or batch data replication process?
Yes, but that’s still rather rare today. In fact, only 10% of survey respondents who have Hadoop in production today are doing master data management (MDM) on Hadoop. But 45% anticipate doing so within three years. Similarly, data quality is in a similar position, with 11% doing it today versus 55% in the future. Personally, I’ve seen it take a while to ramp up all the data management best practices when a new data platform appears. That seems to be the case with Hadoop. But the proliferation of Hadoop into more of the enterprise is driving up requirements for data management best practices, too.
Let’s now focus on your question. Modern MDM architectures typically support a mix of operational and analytic purposes; they do the same on Hadoop.
Today, Hadoop is strong on volume but weak on real-time operation. So MDM (and other operations) are usually exclusively batch oriented. Given strong Hadoop projects like Storm and Spark, real-time data operations will become more favorable soon.
Can we get a use case for Hadoop and MDM?
As I mentioned in the Webinar, MDM on Hadoop is pretty rare today, but survey results show it will soon be far more common, along with similar practices like data quality.
There are many ways to architect an MDM solution, but many are built atop or around some kind of hub, which includes a database or operational data store (ODS) plus appropriate interfaces in and out of the hub. At TDWI, we’ve seen a number of organizations start migrating subsets of enterprise data to Hadoop, and simply modeled databases and ODSs seem to migrate to Hadoop successfully. The straightforward tabular structures of these (unlike complex warehouse dimensions) usually fit well with Hive tables or HBase in the Hadoop environment. With the so-called enterprise data hub on Hadoop gaining in popularity, we should expect to see more migrations like this in coming years.
A lot of MDM master databases (or systems of record) have very wide records, because they’re also used to compile the “complete view” of customers and other enterprise entities. I’ve heard conflicting opinions from Hadoop users; some think Hive tables are best for wide records, while others swear HBase is best. I hear similar debates involving query mechanisms, including HiveQL, Pig, Drill, and Impala. If you contemplate similar tasks, I recommend you take a known ODS to Hadoop and test on both Hive and HBase, with a variety of query approaches.
Can HBase replace a classic data warehouse, and can it compete from a performance side?
If you have a “classic” data warehouse, then I’ll assume it is designed for dimensional models, optimized for complex queries, and supported by a rich metadata layer with auditing capabilities. HBase today is not particularly good with any of those, so it makes an unlikely replacement.
Even so, some pieces of the warehouse environment do well on HBase. For example, many warehouses include a number of operational data stores (ODSs). These may be physically managed in the warehouse’s core database instance, or they may be running on standalone hardware servers and database instances. Either way, I’ve interviewed users who’ve migrated these pieces to HBase—or Hive or both. They say it’s an easy migration, tweaking on the new platform is minimal, and performance is fine, as long as batch processing is all you need. Furthermore, moving these pieces to Hadoop frees up capacity on the warehouse, so it can grow into more data and use cases that truly must reside in the core warehouse platform. Or, if the migrated ODSs were on standalone platforms, then Hadoop seems to work as a consolidation strategy.
There has been less talk [about] making Hadoop transaction oriented, i.e., ACID compliant. Is there any trend or survey outcome?
To be honest, I haven’t looked into transaction processing on Hadoop, although I’ve heard that some people in both open source and vendor communities are working on it.
Why would I be so remiss? Because the leading use cases I see today don’t require transaction processing and hence the four ACID properties. That includes extensions of data warehousing and data integration, plus a wide range of analytics. Upcoming use cases—data archiving and content management—don’t involve transaction processing either. Furthermore, if you want open source software, the other NoSQL database management systems are strong on transaction processing (as are older open source databases), so you may wish to look into those.
I’m sorry to cop out on you with a non-answer. But at least you can see that transaction processing on Hadoop is a low priority for those of us excited about doing data warehouse, data integration, reporting, and analytics on Hadoop.
Posted by Philip Russom, Ph.D. on April 15, 20150 comments