Top Twelve Priorities for Data Warehouse Modernization
By Philip Russom, Senior Research Director for Data Management, TDWI
No matter the vintage or sophistication of your organization’s data warehouse (DW) and the environment around it, it probably needs to be modernized in one or more ways. That’s because DWs and requirements for them continue to evolve. Many users need to get caught up by realigning the DW environment with new business requirements and technology challenges. Once caught up, they need a strategy for continuous modernization.
To help you organize your modernization efforts, here’s a list of the top twelve priorities for data warehouse modernization, including a few comments about why these are important. Think of the priorities as recommendations, requirements, or rules that can guide user organizations into successful strategies for implementing a modernization project.
1. Embrace change. Data warehouse modernization is real; a recent TDWI survey says that 76% of DWs are evolving moderately or dramatically. Given the rampant amount of change in markets and individual businesses, it’s unlikely the status quo will serve you and your organization for much longer. Besides, change is an opportunity for improvement, as long as you manage it with specific directions in mind.
2. Make realignment with business goals your top priority. This is the leading driver according to a recent TDWI survey. Learn the goals of the business and collaborate with business and technical people to determine how business goals map to technology and data. Then base your modernizations on the requirements thus defined. If alignment is achieved, the whole business will modernize, not just the warehouse. And that’s the real point.
3. Make DW capacity a high priority on the technology side. The second most pressing driver is greater capacity for growing data, users, reports. This is no surprise given the explosive growth of traditional enterprise data and new big data. 3-10TB is today’s norm for DW data volume in the average-size organization; however, the norm will soon become 10-100TB, as DW programs graduate from lesser data volumes to greater ones. These are known capacity goals for successful DWs, so keep them in mind when planning capacity modernization.
4. Make analytics a priority, too. One third of DW professionals modernize for better and newer analytics. That’s a technology challenge for the warehouse, since diverse analytic techniques have diverse data preparation requirements, and they don’t all fit the traditional warehouse. Therefore, additional data platforms and tools that complement older ones may be in order. Keep in mind that analytics is what business users want; your pristine data and elegant architecture won’t mean much, if modernization fails to deliver relevant analytics.
5. Don’t forget the related systems and disciplines that also need modernization. Top priorities are analytics, reporting, and data integration, followed by development methods and team characteristics. Align the modernization of the DW, so it can ably provision the data in a manner that these other disciplines require for their success.
6. Don’t be seduced by new, shiny objects. There are lots of new and cool technologies and tools available today, and many get evaluated for DW modernization. Before adopting one, be sure it goes beyond the bling to satisfy real-world requirements in a performant and cost-effective manner.
7. Assume that you’ll need multiple manifestations of modernization. To get the desired results, you should consider multiple modernization strategies, but try not to execute them all at once, in a big bang.
8. Be familiar with today’s tools and techniques for the modern data warehouse environment (DWE). Extending the number and type of standalone platforms within a DWE is one of the strongest trends in data warehouse modernization, because it adds value in the form of additional platforms, without ripping out or replacing established platforms.
9. Adjust the large-scale architecture of your DWE. The rise of the multi-platform DWE is forcing the modernization of system architectures. For most situations, you will keep and improve your centralized, relational DW. But you should expect to complement it with other platforms, then migrate data and balance workloads among platforms. This requires you to rework the large-scale architecture, which determines how diverse platforms integrate and interoperate, plus which data goes where and how data show flow among platforms.
10. Reevaluate your DW platform. The condition of your data is important, but it’s all for naught if the platform can’t capture, manage, and deliver data with speed, scale, and broad functionality at a reasonable cost. Replacing a DW platform is disruptive and expensive for a business. Therefore, consider leaving your existing DW platform in place, but update it and complement it with other systems. Even so, grossly deficient or outmoded platforms should be replaced.
11. Consider Hadoop for various roles in the DWE. Hadoop’s massive and cheap storage offloads older systems by taking responsibility for data staging, ELT push down, and the archiving of detailed source data (retained for advanced analytics). Hadoop also serves as a massively parallel execution engine for a wide variety of set-based and algorithmic analytic methods. Conventional wisdom says Hadoop usually complements a DW without replacing it. That’s what early adaptors do with Hadoop in DWEs today. And the number of organizations integrating Hadoop with a DW continues to increase.
12. Develop plans and recurring cycles for DW modernization. Most DW teams have settled on a quarterly schedule for updating DWs. This applies to tasks of many sizes; well-contained phases of some modernization projects may fit this scheme, as well. However, large-scale modernizations typically need their own plan. The more disruptive a modernization (such as rip-and-replace), the more critical to success is the multi-phase plan (sometimes the multi-year plan). Modernization affects business users and their processes; for minimal disruption, business managers should be involved in developing and executing modernization plans.
ANNOUNCEMENT
To learn more about modernizing data warehouses and related IT systems, attend my TDWI webinar Data Warehouse Modernization in the age of Big Data Analytics, coming up on April 14, 2016. Register online for the webinar: http://bit.ly/DWMod16
This webinar will quantify trends in data warehouse modernization and catalog technologies that are relevant. It will also document strategies and user best practices for organizing modernization projects. The goal is to help DW professionals and their business counterparts plan the next generation of their data warehouse, in alignment with business goals.
Posted on March 24, 20160 comments
New big data sources and data types – and the need to get business value from new data – are forcing organizations to evolve their data management practices.
By Philip Russom, TDWI Research Director for Data Management
I recently participated as a core speaker in the Informatica Big Data Ready Virtual Summit, sharing a session with Amit Walia, the Chief Product Officer at Informatica Corporation. Amit and I had an interactive conversation where we discussed one of the most pressing questions in data management today, namely: How should an organization get ready to capture and leverage big data? This is an important question, because many organizations in many industries are facing big data, with its new data sources, data types, large volumes, and fast generation rates. Organizations need to modernize their data integration (DI) infrastructure, so they can capture and leverage the new data for new business insights and analytics.
Amit Walia and I boiled down this complex issue to seven recommendations, which I will now summarize:
Achieve agility and autonomy, as required of big data and analytics. The creation of data management solutions must keep up with the pace of business by adopting agile and lean development methods. New tool functions that assist with agility and autonomy include those for data exploration and profiling, self-service data access, and rapid dataset prototyping (or “data prep”).
Govern big data, as you would any enterprise data asset. Big data has a bit of a “hall pass” today, because it’s new and exotic. But eventually, it will be assimilated as yet another category of enterprise data. Prepare for that day, by assuming that new data demands governance, stewardship, privacy, security, quality, and standards.
Include Hadoop in your data integration infrastructure. Hadoop can replace some of the database management systems and file systems you’re using today, while scaling at a reasonable cost and handling new data types. Modern users’ DI architectures already include Hadoop for landing, staging, push-down processing, archiving, hubs, and lakes.
Integrate fit-for-purpose data to enable data exploration and profiling. The trend is to integrate big data in its raw, original state, into a big data platform, such as Hadoop or a large relational MPP implementation. That way, users can explore and profile new big data to determine its business value. Later, users can repurpose discovered data many ways, sometimes at runtime, as new requirements arise for analytics or operations.
Embrace real-time data ingestion, as required by some forms of big data and analytics. A modern DI infrastructure supports many speeds and frequencies of data ingestion, because diverse data sources and business processes have diverse requirements relative to time. A new challenge for DI is to capture and process, streaming data in real time, to enable near time analytics and business operations.
Prepare to integrate big data by upgrading skills and team structures. TDWI surveys say that a lack of skill is the biggest barrier to success with new big data. Data management professionals need training for Hadoop, NoSQL, natural language processing, and new data types (e.g., JSON, social media, streams). These competencies should be added to those of existing DI competency centers.
Modernize data management solution development by combining agile, stewardship, and collaborative methods. Both agile and stewardship methods recommend the use of a pair of specialists, working together closely: a data specialist and a business representative (or steward). This “dynamic duo” accelerates requirements gathering, ensures data-to-business alignment, and delivers solutions faster than ever.
If you’d like to hear more of my discussion with Informatica’s Amit Walia (and hear other expert speakers in the Informatica Big Data Ready Virtual Summit, too), please replay the Informatica Webinar by clicking here.
Posted on January 6, 20160 comments
An Introduction to Apache Spark and its uses in Business Intelligence (BI), Data Warehousing (DW), and Advanced Analytics
Blog by Philip Russom
Research Director for Data Management, TDWI
At TDWI, we’re hearing a lot of interest in Apache Spark, although it’s still new and most users are unfamiliar with it. So, please allow me to define Spark for you, explain its potential benefits, and describe actual use cases.
Apache Spark is a parallel processing engine. It specializes in big data, and works well with Hadoop environments. However, Apache is not just for Hadoop; it provides parallel processing for other environments, too. Spark is known for high speed and low latency, which it achieves by leveraging in-memory computing and cyclic data flows.
Spark is fast. Very fast. Benchmarks show Spark to be up to one hundred times faster than Hadoop MapReduce with in-memory operations. Spark is ten times faster than MapReduce with disk-bound operations. The point is that Spark has the low latency required of new data-driven practices, like data exploration, discovery, streaming analytics, and SQL-based analytics.
Spark functions apply directly to applications in BI, DW, DI, & analytics. Spark today includes four libraries of functionality, and each is of interest to professionals in BI, DW, and analytics. The libraries support ANSI-standard SQL, streaming data, machine learning, and graph analytics.
A Spark library provides native support for ANSI and ISO standard SQL. In a recent TDWI survey, 69% of users surveyed said that ANSI- and ISO-standard SQL on Hadoop is required for broad enterprise use. That’s because a modern enterprise wants to leverage pre-existing SQL skills and SQL-based tools. Furthermore, users want fast queries on Hadoop, to enable data exploration, analytics, and other interactive, data-driven practices. Spark and its SQL support promise to enable these – in both batch or interactive sessions, for Hadoop and other environments – which in turn will spark big data analytics for users in BI, DW, and analytics.
Spark offers broad compatibility. Spark SQL reuses the Hive front-end and metastore, to provide compatibility with existing Hive data, queries, UDFs. Spark SQL’s server mode extends interoperability via industry-standard ODBC/JDBC. Spark can process data in S3, HDFS, HBase, Hive, Cassandra, and any Hadoop InputFormat.
Spark can be deployed many ways. Spark requires some kind of shared file system (NFS compliant), so its deployment options are diverse. Spark runs on its standalone cluster, Hadoop YARN, Apache Mesos, and Amazon EC2; on premises or cloud. A single job, query, or stream processing can be deployed in either batch or interactive mode via Scala, Python, and R shells.
Spark has one console for the seamless development of diverse functionality. Apache Spark includes libraries for four high-level applications: SQL, streaming data, machine learning, and graph analytics. These are integrated tightly, so users can create applications that mix SQL queries and stream processing alongside complex analytic algorithms.
Spark and its libraries enable several application types for BI, DW, and analytics:
- SQL analytics and related set-based applications – e.g., data exploration and discovery, customer-base segmentation, financial analyses, dimensional modeling and analysis, reporting, ETL pushdown that requires SQL
- Stream capture and analysis -- monitoring facilities (utilities, factories), tracking social sentiment, predictive machine maintenance, reroute vehicle traffic, manage mobile assets, any time-sensitive process
- Graph analytics -- anomaly detection for fraud or risk, behavioral analysis, entity clustering, patient outcome optimization
- Mixtures of the above – a trend among users is to mix multiple analytic methods in a single application, because each reveals different insights
Want to learn more about Spark? Click here to replay my recent TDWI Webinar, where go into more detail about Spark and its uses in BI, DW, and analytics.
Posted by Philip Russom, Ph.D. on December 7, 20150 comments
Blog by Philip Russom
Research Director for Data Management, TDWI
To help you better understand what today’s emerging technologies and methods (ETMs) are – especially those related to business intelligence, analytics, and data warehousing – I’d like to share with you the series of 25 tweets I recently issued on the topic. I think you’ll find the tweets interesting, because they provide an overview of ETMs in a form that’s compact, yet amazingly comprehensive.
Each tweet below is a short sound bite or stat bite drawn from the recent TDWI report “Emerging Technologies for Business Intelligence, Analytics, and Data Warehousing,” which I researched and wrote with my colleagues David Stodder and Fern Halper. Many of the tweets focus on a statistic cited in the report, while other tweets are definitions stated in the report.
I left in the arcane acronyms, abbreviations, and incomplete sentences typical of tweets, because I think that all of you already know them or can figure them out. Even so, I deleted a few tiny URLs, hashtags, and repetitive phrases. I issued the tweets in groups, on related topics; so I’ve added some headings to this blog to show that organization. Otherwise, these are raw tweets.
Examples of Emerging Technologies and Methods (ETMs)
1. Most Emerging Techs & Methods (#ETMs) fall into 3 layers of BI, #analytics & #EDW tech stack.
2. #ETMs for #BI include #DataViz, #DataExploration, #DataPrep, #Dashboards, #MashUps, #MobileBI.
3. #ETMs for #Analytics operate on data from #SocialMedia, #IoT, streams, #MachineData.
4. #ETMs for #DataMgt include #Hadoop, #ApacheSpark, #NoSQL, in-DBMS #analytics, in-mem DBMS, columnar.
Examples of Emerging Methods and Platforms
5. #EmergingMethods include agile & lean dev methods applied to whole BI/DW/#analytics tech stack.
6. Other #EmergingMethods include #CompetencyCenters, #CollaborativeBI, #StoryTelling, #DataGovernance.
7. Emerging platforms include many types of clouds, #SaaS, #OpenSource, appliances...
The Importance of ETMs
8. #TDWI SURVEY SEZ: Emerging Techs & Methods (#ETMs) are very important (53%) or somewhat (39%).
9. #TDWI SURVEY SEZ: Emerging Techs & Methods (#ETMs) are opportunity to compete, evolve, perform (79%).
10. #TDWI SURVEY SEZ: Two-thirds of respondents (64%) already have #ETMs in production.
Benefits and Barriers for ETMs
11. #TDWI SURVEY SEZ: Top benefits of #ETMs = competitiveness, decision support, biz performance, innovation.
12. #TDWI SURVEY SEZ: Top barriers to #ETMs = LACK of skills, budgets, biz value, innovation.
13. #TDWI SURVEY SEZ: Other barriers to #ETMs = poor state of IT infrastructure & poor #DataGovernance.
User Satisfaction with Current State of ETMs
14. #TDWI SURVEY SEZ: 41% dissatisfied with their enterprise adoption of Emerging Techs & Methods (#ETMs).
15. Adoption of agile development methods is one of strongest trends in BI, #analytics, #EDW today.
16. #TDWI SURVEY SEZ: 55% dissatisfied with time required of development for BI, #analytics, #DataMgt.
User Success with Current State of ETMs
17. #TDWI SURVEY SEZ: Users successful with #ETMs for #SelfServiceBI (54%) & #DataPrep (50%).
18. #Hadoop & #NoSQL #ETMs are challenging for tools & apps built for relational data.
Emerging Data Types for Analytics
19. #TDWI SURVEY SEZ: 84% analyze structured data today. Suprising that 16% are not; maybe text analytics?
20. #TDWI SURVEY SEZ: #IoT data used by <20% of respondents today, but 40% more will use within 3 years.
21. Other data sources poised for growth = Machine data (sensors, devices) & #RealTime #EventStreaming.
22. #TDWI SURVEY SEZ: In clouds, users already do #EDW (35%), #Analytics (31%), sandbox (29%), DataInt (24%).
23. #TDWI SURVEY SEZ: 49% have production #PredictiveAnalytics today; another 39% will in 3 yrs.
ETMs for Data Warehousing & Data Management
24. #TDWI SURVEY SEZ: 3-yr hi growth in #DataMgt #ETMs = #RealTime, streams, #DataPrep, #Hadoop, #CloudDW.
25. Top security #ETMs for #DataMgt = #DataProtection (encrypt, mask, token), not just user name/pswd.
Want to learn more about Emerging Technologies and Methods (ETMs)?
For a more detailed discussion – in a traditional publication! – get the TDWI Best Practices Report, titled “Emerging Technologies for Business Intelligence, Analytics, and Data Warehousing,” which is available in a PDF file via a free download.
You can also register for and replay the TDWI Webinar, where David Stodder, Fern Halper, and I discuss the findings of the TDWI report.
Posted by Philip Russom, Ph.D. on November 9, 20150 comments
Take a look at 5 new resources that can help you evolve your analytics strategies beyond spreadsheets and dashboards. Create more value from your data when you move beyond simple business intelligence (BI) reporting to data discovery and advanced analytics. Use these recently released resources to develop your competitive advantage.
1. |
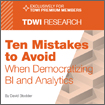 |
Ten Mistakes to Avoid When Democratizing BI and Analytics
Premium member resource—freely available until May 29
Download Now |
|
2. |
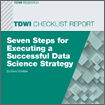 |
Seven Steps for Executing a Successful Data Science Strategy
Download Now |
|
3. |
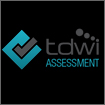 |
TDWI Analytics Maturity Model Assessment & Guide
Download Now |
|
4. |
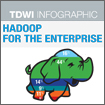 |
TDWI Infographic: Hadoop for the Enterprise
Download Now |
|
5. |
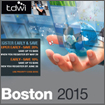 |
Upcoming Live Event: Special Offer Below
TDWI Boston 2015 | The Analytics Experience
July 26-31, 2015
Six action-packed days filled
with classes, case studies, and hands-on training (WebAction, Tableau, Luminoso, Yellowfin, Archipelago, Data Mining with R, Hadoop, and more) offer an accelerated learning experience for business and
technical leaders and implementers.
Learn More |
|
REGISTER NOW & SAVE BIG |
|
The Analytics Experience | Boston 2015
July 26–31, 2015
Sign up now for the SUPER EARLY registration discount
20% off until May 29—Save up to $855!
Use priority code SEB20
Learn More
|
|
Posted by TDWI on May 15, 20150 comments
By Philip Russom, Research Director for Data Management, TDWI
To help you better understand Hadoop’s evolution into mainstream enterprise usage—and why you should care—I’d like to share with you the series of 25 tweets I recently issued on the topic. I think you’ll find the tweets interesting, because they provide an overview of enterprise Hadoop and its best practices in a form that’s compact, yet amazingly comprehensive.
Every tweet I wrote was a short sound bite or stat bite drawn from my recent TDWI report
Hadoop for the Enterprise. Many of the tweets focus on a statistic cited in the report, while other tweets are definitions stated in the report.
I left in the arcane acronyms, abbreviations, and incomplete sentences typical of tweets, because I think that all of you already know them or can figure them out. Even so, I deleted a few tiny URLs, hashtags, and repetitive phrases. I issued the tweets in groups, on related topics; so I’ve added some headings to this blog to show that organization. Otherwise, these are raw tweets.
Introduction to Hadoop for the Enterprise
1. #Hadoop is expanding into more industries, use cases & enterprise breadth. More in #TDWI Webinar Apr. 14 Noon ET http://bit.ly/1F9d2iy
2. #Hadoop for the Enterprise tech drivers: scalability, low cost, & many data types.
3. #Hadoop for the Enterprise biz drivers: #analytics, data exploration, value from #BigData.
Hadoop Adoption is Up
4. #TDWI SURVEY SEZ: #Hadoop adoption accelerating. Production clusters up 60% in 2 yrs.
5. #TDWI SURVEY SEZ: Half of respondents have #Hadoop clusters in development, coming online in 12 months.
6. #TDWI SURVEY SEZ: 60% of users surveyed will have #Hadoop in production by 2016.
Benefits and Barriers
7. #TDWI SURVEY SEZ: 89% surveyed say #Hadoop is opportunity for biz/tech #innovation.
8. #TDWI SURVEY SEZ: #Hadoop’s benefits: improve #analytics, #EDW, scalability, exotic data.
9. #TDWI SURVEY SEZ: #Hadoop’s barriers: weak skills, biz case, security, open source tools.
Organizational Issues with Enterprise Hadoop
10. As #Hadoop goes enterprise scope, ownership, staffing, dev methods & economics shift.
11. #Hadoop clusters are becoming central, shared IT infrastructure in mainstream firms.
12. #TDWI SURVEY SEZ: Common #Hadoop job titles are: #DataScientist, architect, analyst, developer.
13. #TDWI SURVEY SEZ: Firms train employees in #Hadoop cuz they can’t find or afford folks to hire.
The Many Use Cases for Enterprise Hadoop
14. #TDWI SURVEY SEZ: Leading future #Hadoop uses: ent data hubs, archives, misc BI/DW.
15. #TDWI SURVEY SEZ: Half of respondents will add #DataQuality & #MDM for #Hadoop data.
16. #TDWI SURVEY SEZ: Established #Hadoop practice extends a #DataWarehouse (46%).
17. #TDWI SURVEY SEZ: Data lakes (36%) & enterprise data hubs (28%) are new practices for #Hadoop.
18. #TDWI SURVEY SEZ: Archiving on #Hadoop is upcoming for new (36%) & old (19%) data.
19. #TDWI SURVEY SEZ: #Hadoop for content mgt (17%) & operational ent apps (11%) are new.
Hadoop’s Roles in Enterprise Data Strategies and Architectures
20. #TDWI SURVEY SEZ: 66% feel #Hadoop is important to their enterprise data strategy.
21. #TDWI SURVEY SEZ: #Hadoop is becoming key to multi-platform #DataWarehouse environments (DWEs).
22. #TDWI SURVEY SEZ: a third of #Hadoop clusters are off premises, on cloud, SaaS, managed provider. Surprising!
Hadoop Development Details
23. #Hadoop cluster size scales down to dept use (8 nodes) or up to enterprise (1000 nodes).
24. #TDWI SURVEY SEZ: #Hadoop clusters per enterprise = 10 on average, with median at 4.
25. #TDWI SURVEY SEZ: 58% of #Hadoop dev done w/mix of hand-coding & hi-level tools. 23% coded only.
Want to learn more about Hadoop for the Enterprise?
For a more detailed discussion—in a traditional publication!—get the TDWI Best Practices Report
Hadoop for the Enterprise, which is available in a
PDF via a free download.
You can also register for and
replay my TDWI Webinar, where I present the findings of
Hadoop for the Enterprise.
Posted by Philip Russom, Ph.D. on April 27, 20150 comments
Attendees of a recent TDWI Webinar asked excellent questions.
By Philip Russom, TDWI Research Director for Data Management
Recently, on April 14, I broadcast a TDWI Webinar in which I presented some of the findings from my new TDWI report on "Hadoop for the Enterprise." You can download a free copy of the report in a PDF, and you can replay the Webinar. With each link, you may need to scroll down to find what you want. If you’re new to Hadoop, you may wish to first read the 2013 TDWI Best Practices Report Integrating Hadoop into Business Intelligence and Data Warehousing.
Attendees of the Webinar posed several very good questions about various issues around Hadoop. Please allow me to share a few attendee questions and the answers I sent them via e-mail:
What is a Hadoop cluster? And why would an organization need more than one?
The Wikipedia article on “Computer Cluster” is a good general description of all clustered server pools. The article doesn’t mention Hadoop, but Hadoop’s clustering strategy is in line with the article, except that Hadoop can run on heterogeneous servers, whereas the article recommends that all servers be identical. The point of any cluster is to get scalable and high-perfromance computational power, but at a relatively low cost because of commodity priced hardware.
An organization may need more than one Hadoop cluster, due to departmental funding and sponsorship (which is common with analytic applications) or other organizational dynamics. As I pointed out in the Webinar, as users decide on a strategy for Hadoop on an enterprise scale, they tend to abandon the departmental focus in favor of central IT providing Hadoop as a shared enterprise asset (as IT often does with corporate networks, racks of servers, and storage subsystems).
You don't need big data to take advantage of Hadoop?
That’s correct. I’ve found many user organizations with a small Hadoop implementation (8 nodes seems common) used as the data layer under a departmental analytic application or analytics sandbox of some sort. Hadoop makes sense when the department has exotic data (perhaps in lots of files), which Hadoop excels with. Use cases include sentiment analytics with schema-free human language text or supplier analytics with multi-structured XML or JSON files.
Note that, in the examples, the data volumes are modest, but it’s still “big data” in the sense that it’s not the usual structured and relational data. For many users dealing with big data (whether on Hadoop or elsewhere), the value proposition is that big data is new and different, and therefore offers new insights and more complete views of customers. Even when big data is truly big (tens of terabytes or more), users don’t have much trouble managing it; hence, big data is not a scalability crisis, as some people have claimed.
Hadoop has a well-deserved reputation for scaling up linearly. But these examples show that Hadoop also scales down successfully.
Do companies transfer master data into Hadoop to support analytics in a real-time or batch data replication process?
Yes, but that’s still rather rare today. In fact, only 10% of survey respondents who have Hadoop in production today are doing master data management (MDM) on Hadoop. But 45% anticipate doing so within three years. Similarly, data quality is in a similar position, with 11% doing it today versus 55% in the future. Personally, I’ve seen it take a while to ramp up all the data management best practices when a new data platform appears. That seems to be the case with Hadoop. But the proliferation of Hadoop into more of the enterprise is driving up requirements for data management best practices, too.
Let’s now focus on your question. Modern MDM architectures typically support a mix of operational and analytic purposes; they do the same on Hadoop.
Today, Hadoop is strong on volume but weak on real-time operation. So MDM (and other operations) are usually exclusively batch oriented. Given strong Hadoop projects like Storm and Spark, real-time data operations will become more favorable soon.
Can we get a use case for Hadoop and MDM?
As I mentioned in the Webinar, MDM on Hadoop is pretty rare today, but survey results show it will soon be far more common, along with similar practices like data quality.
There are many ways to architect an MDM solution, but many are built atop or around some kind of hub, which includes a database or operational data store (ODS) plus appropriate interfaces in and out of the hub. At TDWI, we’ve seen a number of organizations start migrating subsets of enterprise data to Hadoop, and simply modeled databases and ODSs seem to migrate to Hadoop successfully. The straightforward tabular structures of these (unlike complex warehouse dimensions) usually fit well with Hive tables or HBase in the Hadoop environment. With the so-called enterprise data hub on Hadoop gaining in popularity, we should expect to see more migrations like this in coming years.
A lot of MDM master databases (or systems of record) have very wide records, because they’re also used to compile the “complete view” of customers and other enterprise entities. I’ve heard conflicting opinions from Hadoop users; some think Hive tables are best for wide records, while others swear HBase is best. I hear similar debates involving query mechanisms, including HiveQL, Pig, Drill, and Impala. If you contemplate similar tasks, I recommend you take a known ODS to Hadoop and test on both Hive and HBase, with a variety of query approaches.
Can HBase replace a classic data warehouse, and can it compete from a performance side?
If you have a “classic” data warehouse, then I’ll assume it is designed for dimensional models, optimized for complex queries, and supported by a rich metadata layer with auditing capabilities. HBase today is not particularly good with any of those, so it makes an unlikely replacement.
Even so, some pieces of the warehouse environment do well on HBase. For example, many warehouses include a number of operational data stores (ODSs). These may be physically managed in the warehouse’s core database instance, or they may be running on standalone hardware servers and database instances. Either way, I’ve interviewed users who’ve migrated these pieces to HBase—or Hive or both. They say it’s an easy migration, tweaking on the new platform is minimal, and performance is fine, as long as batch processing is all you need. Furthermore, moving these pieces to Hadoop frees up capacity on the warehouse, so it can grow into more data and use cases that truly must reside in the core warehouse platform. Or, if the migrated ODSs were on standalone platforms, then Hadoop seems to work as a consolidation strategy.
There has been less talk [about] making Hadoop transaction oriented, i.e., ACID compliant. Is there any trend or survey outcome?
To be honest, I haven’t looked into transaction processing on Hadoop, although I’ve heard that some people in both open source and vendor communities are working on it.
Why would I be so remiss? Because the leading use cases I see today don’t require transaction processing and hence the four ACID properties. That includes extensions of data warehousing and data integration, plus a wide range of analytics. Upcoming use cases—data archiving and content management—don’t involve transaction processing either. Furthermore, if you want open source software, the other NoSQL database management systems are strong on transaction processing (as are older open source databases), so you may wish to look into those.
I’m sorry to cop out on you with a non-answer. But at least you can see that transaction processing on Hadoop is a low priority for those of us excited about doing data warehouse, data integration, reporting, and analytics on Hadoop.
Posted by Philip Russom, Ph.D. on April 15, 20150 comments
Evolving Best Practices for Data Management
By Philip Russom, TDWI Research Director for Data Management
I recently broadcast a really interesting Webinar with David Lyle, a vice president of product strategy at Informatica Corporation. David and I had a “fireside chat” where we discussed one of the most pressing questions in data management today, namely: How can we prepare great data for great analytics, while still leveraging older best practices in data management? Please allow me to summarize our discussion.
Both old and new requirements are driving organizations toward analytics. David and I started the Webinar by talking about prominent trends:
- Wringing value from big data: The consensus today says that advanced analytics is the primary path to business value from big data and other types of new data, such as data from sensors, devices, machinery, logs, and social media.
- Getting more value from traditional enterprise data: Analytics continues to reveal customer segments, sales opportunities, and threats for risk, fraud, and security.
- Competing on analytics: The modern business is run by the numbers, not just gut feel, to study markets, refine differentiation, and identify competitive advantages.
The rise of analytics is a bit confusing for some data people. As experienced data professionals do more work with advanced forms of analytics (enabled by data mining, clustering, text mining, statistical analysis, etc.) they can’t help but notice that the requirements for preparing analytic data are similar-but-different as compared to their other projects, such as ETL for a data warehouse that feeds standard reports.
Analytics and reporting are two different practices. In the Webinar, David and I talked about how the two involve pretty much the same data management practices, but in different orders and priorities:
- Reporting is mostly about entities and facts you know well, represented by highly polished data that you know well. Squeaky clean report data demands elaborate data processing (for ETL, quality, metadata, master data, and so on). This is especially true of reports that demand numeric precision (about financials or inventory) or will be published outside the organization (regulatory or partner reports).
- Advanced analytics, in general, enables the discovery of facts you didn’t know, based on the exploration and analysis of data that’s probably new to you. Preparing raw source data for analytics is simple, though at high levels of scale. With big data and other new data, preparation may be as simple as collocating large data sets on Hadoop or another platform suited to data exploration. When using modern tools, users can further prepare the data as they explore it, by profiling, modeling, aggregating, and standardizing data on the fly.
Operationalizing analytics brings reporting and analysis together in a unified process. For example, once an epiphany is discovered through analytics (e.g., the root cause of a new form of customer churn), that discovery should become a repeatable BI deliverable (e.g., metrics and KPIs that enable managers to track the new form of churn in dashboards). In these situations, the best practices of data management apply to a lesser degree (perhaps on the fly) during the early analytic steps of the process, but then are applied fully during the operationalization steps.
Architectural ramifications ensue from the growing diversity of data and workloads for analytics, reporting, multi-structured data, real time, and so on. For example, modern data warehouse environments (DWEs) include multiple tools and data platforms, from traditional relational databases to appliances and columnar databases to Hadoop and other NoSQL platforms. Some are on premises and others are on clouds. On the downside, this results in high complexity, with data strewn across multiple platforms. On the upside, users get great data for great analytics by moving data to a platform within the DWE that’s optimized for a particular data type, analytic workload, price point, or data management best practice.
For example, a number of data architecture uses cases have emerged successfully in recent years, largely to assure great data for great analytics:
- Leveraging new data warehouse platform types gives analytics the high performance it needs. Toward this end, TDWI has seen many users successfully adopt new platforms based on appliances, columnar data stores, and a variety of in-memory functions.
- Offloading data and its processing to Hadoop frees up capacity on EDWs. And it also gives unstructured and multi-structured data types a platform that is better suited to their management and processing, all at a favorable cost point.
- Virtualizing data assets yields greater agility and simpler data management. Multi-platform data architectures too often entail a lot of data movement among the platforms. But this can be mitigated by federated and virtual data management practices, as well as by emerging practices for data lakes and enterprise data hubs.
If you’d like to hear more of my discussion with Informatica’s David Lyle, please replay the Webinar from the Informatica archive.
Posted by Philip Russom, Ph.D. on February 2, 20150 comments
Attendees of a recent TDWI Webinar asked excellent questions.
By Philip Russom, TDWI Research Director for Data Management
Recently, on Tuesday April 15, 2014, I broadcasted a TDWI Webinar in which I presented some of the findings from my new TDWI report, Evolving Data Warehouse Architectures in the Age of Big Data. You can download a free copy of the report in a PDF file. And you can replay the Webinar.
Attendees of the Webinar posed several very good questions about various issues in data warehouse architecture. Please allow me to share a few of the attendees’ questions and the answers I sent them via e-mail:
Q. As we update our data warehouse from more reporting to more analytics functions, should we design a brand new data warehouse architecture, or improve from the existing one?
If the existing data warehouse and its architecture fulfill business requirements and technical performance requirements (for speed and scale), then you should try to build out the existing architecture. For that to work, your existing vendor platform under the warehouse must perform well with multiple mixed workloads, including analytic workloads; ask your vendor representative for customer references who’ve succeeded with mixed workloads. Also, building up data sets for advanced analytics typically means loading large data volumes into the warehouse, which may cost more money with some licenses; again, ask your vendor if there are such ramifications under your current license.
If your current core warehouse platform cannot support mixed workloads with high performance (or adding analytic data costs too much money), you may decide to manage and process large data sets for advanced analytics on a separate standalone platform that integrates with your warehouse. But in that case, you still keep your existing data warehouse and most of its data structures intact, just making slight changes for better integration with the new additional platform(s) for advanced analytics.
Q. Given the lack of integration across this multi-platform [data warehouse] environment, how do we avoid the need to replicate DW transactional sources into the big data platforms, as transactions are required in mining?
Good question, and there are number of issues here. First, a well-designed multi-platform environment won’t suffer a “lack of integration.” TDWI’s definition of “logical data warehouse” is that the logical design specifies integration schemes (not just data models) across physically distinct platforms, whether that integration takes a data model approach (as in shared or conformed dimensions, etc.) or a data integration approach (as in jobs for ETL, replication, etc.) or both. Second, I take your point, that replicating data more than needed can lead to a variety of problems, as data gets out of sync and loses integrity. A good architecture can minimize replication, and sometimes alleviate it. Third, for decades, users have faced the same decision you’re looking at: do we store, manage, and analytically process our rich, valuable collection of transactional data in the warehouse proper or on a standalone but integrated platform, such as the usual operational data store (ODS)?
For years, a solution I’ve seen users successfully adopt is to deploy a homegrown ODS that they’ve designed and optimized for transactions. The ODS is on a standalone platform that’s integrated with the core warehouse (plus other ODSs, marts, etc.), running on a relational DBMS atop commodity priced hardware. Note that the upcoming trend is toward ODSs atop Hadoop (but only if the data volumes are massive). The idea is to manage transactional data on a platform that’s much cheaper than the DW, on a standalone platform where the relentless sorting, updating, and processing of that data won’t degrade warehouse performance. Yet, the ODS is easily reached from all tools, plus through data federation and virtualization as well, which minimizes the replication of transactional data.
If you give the ODS the capacity it needs to persist multiple sort orders and data subsets in the ODS, then copying data outside the ODS is further reduced. Also, if you use data mining tools that can work on data “in situ” (i.e., in the ODS’s relational database) without moving data to the tool, then that also reduces copying and moving transactional data.
Q. The need for data warehouses is never going to go away. But isn’t the separation between "operations" and "analytics" starting to blur? In other words, the future isn't DWE; it's a "data environment" that does both.
Operational BI is all about getting operational data into BI faster and more frequently, while also embedding BI functions in operational applications and their processes as well. Operational BI is a very popular practice. It has been for years, and will get even more popular, as organizations adjust their BI efforts to bring them closer to real time (to be more competitive, customer conscious, efficient, etc.). The widespread existence of operational BI corroborates that the line between operations and BI is already quite blurred and will become even more so.
In another trend, many organizations are purposefully evolving toward a more or less loosely unified data environment for most enterprise data. I say “more or less” and “loosely” because early adopters are quick to say that the architecture is not 100 percent of the enterprise and integration is spotty, on an “as needed” basis. As one architect joked, “it’s more archaeology than architecture, because the work usually consists of imposing a logical architecture over mature, preexisting systems.” For early adopters, it makes sense to architect data globally, when customer data and some other data domains are pervasively shared across multiple applications, departments, and processes. It also makes sense in firms where business processes ramble across multiple business units and IT systems. Obviously, there’s an infinitude of resulting enterprise data architectures.
The data warehouse environment (DWE) I’m describing is a local microcosm of such a broad and loosely unified multi-platform data architecture. However, in some organizations today, the data warehouse and similar data platforms are just a few among many other data platforms, integrated on an enterprise scale. But those organizations are as yet the minority, although we at TDWI expect it to be the norm for IT-intense organizations within five years. TDWI’s Vegas conference has been devoted to issues in enterprise-scale data architecture for years, and will continue to be. You might consider attending next February.
Q. Can you point us to white papers on the difference between reporting and analytics [and how that affects DW architecture]?
You can read my blog on the subject. Or you could read the new report on evolving data warehouse architectures, because I adapted material from the blog to become a section in the report, starting on page 24.
Q. What’s the role, or is there a role, for variants like an ODS in the new world [of data warehouse architectures]? Is it part of the real-time world?”
Historically, some of the first standalone systems in a multi-platform data warehouse (going back to the mid-1990s) were ODSs deployed on their own hardware sever with their own DBMS instances. These are still with us, and will continue to be with us, as data warehouse environments evolve into even more platforms used at once. An ODS can be designed and optimized by users for a wide range of data domains and uses (including real-time data), but I’m currently seeing a lot of users deploying ODSs for various types of big data and other data earmarked for advanced analytics.
Q. Saying Inmon vs. Kimball is no longer relevant is like saying Newton is no longer relevant in the world of physics today. It's still important, maybe not as fundamental as 1–2 decades ago.
For decades, Newton practiced alchemy in his copious spare time, because he was convinced that changing lead to gold was possible. Our heroes aren’t always 100 percent right.
Concerning Inmon and Kimball, see the top of page 7 in the report. Also please read the User Story on that same page. “No longer relevant” is your phrase, not mine. In my view, Inmon and Kimball’s innovations are as relevant as ever, and are still being applied daily. And they just keep giving: Inmon has recently extended our understanding of unstructured data and Kimball is currently working new best practices for Hadoop.
It’s the users who’ve changed. Instead of arguing about which to choose, users choose to apply Inmon and Kimball techniques (and others, too) in the same extended warehouse environment. And that’s a wise choice on their part, since hybrids and diversity seem to be winning strategies for a growing number of user organizations and their diversified DW architectures nowadays.
Q. Some organizations consider Hadoop a replacement for their current DW appliance. How is this possible?
As I said in the Webinar, I’ve only found two organizations that took out a data warehouse and put Hadoop in its place. While that corroborates that a replacement is possible, it’s not likely, nor is it a compelling trend.
Instead of replacement, we at TDWI see far more users augmenting their data warehouse environment with the Hadoop Distributed File System (HDFS), plus related Hadoop tools, especially MapReduce, Hive, HBase, and Pig. In short, HDFS handles things that relational warehouses are not designed for, such as unstructured data, algorithmic analytics, millions of files, and petabyte-size data sets. But the relational warehouse is still best for the structured and multidimensional data that goes into standard reports, performance management, and set-based analytics (typically OLAP or SQL-based analytics).
Another possibility is that Hive atop MapReduce and HDFS makes a highly scalable “row store” type of database. Sometimes you don’t need a full-featured (and expensive) relational DBMS, and hence a row store will do just fine. For example, many of the ODSs found today in data warehouse environments are candidates for migration to Hadoop. That includes ODSs that manage large “archives” (I use the word loosely) of transactional data and other operational data that’s persisted and kept long-term for advanced analytics that just need simple tabular structures. Most standalone ODSs of that description today run on mature DBMSs, but could run almost as well (for less money) on Hadoop.
Finally, let’s remember that not all organizations need a data warehouse, as represented by 15 percent of survey respondents.
Q. Can you recommend any sample success stories on how to integrate Hadoop or similar big data into an existing data warehouse [environment]?
Yes, many real-world use cases and user stories are discussed in the 2013 TDWI report Integrating Hadoop into Business Intelligence and Data Warehousing.
Posted by Philip Russom, Ph.D. on April 30, 20140 comments
By Philip Russom
Research Director for Data Management, TDWI
To help you better understand the ongoing evolution of data warehouse architectures and why you should care, I’d like to share with you the series of 35 tweets I recently issued on the topic. I think you’ll find the tweets interesting because they provide an overview of big data management and its best practices in a form that’s compact, yet amazingly comprehensive.
Every tweet I wrote was a short sound bite or stat bite drawn from my recent TDWI report Evolving Data Warehouse Architectures in the Age of Big Data. Many of the tweets focus on a statistic cited in the report, while other tweets are definitions stated in the report.
I left in the arcane acronyms, abbreviations, and incomplete sentences typical of tweets, because I think that all of you already know them or can figure them out. Even so, I deleted a few tiny URLs, hashtags, and repetitive phrases. I issued the tweets in groups, on related topics; so I’ve added some headings to this blog to show that organization. Otherwise, these are raw tweets.
Basic Components of the Average Data Warehouse Architecture
- Most DW Arch’s have 4 layers: logical, physical, hardware topology, data standards.
- DW logical architecture is mostly about data models, entity models & relationships.
- DW logical arch also defines standards for data models, dev practices, interfaces, etc.
- DW physical architecture is mostly a plan for data deployment on servers.
- DW physical arch also defines topology for hardware & software servers plus interfaces.
Users’ Views of Architectural Components
- #TDWI SURVEY SEZ: Data standards & rules are highest priority (71%) of #EDW architecture.
- #TDWI SURVEY SEZ: Logical design (66%) is the starting point of an #EDW architecture.
- #TDWI SURVEY SEZ: Physical plan (56%) locates logical pieces in an #EDW architecture.
- #TDWI SURVEY SEZ: Only 12% have #EDW that’s “collection of data & platforms without a plan.”
- #TDWI SURVEY SEZ: Only 12% feel Inmon vs Kimball argument is priority for #EDW architecture.
The Evolution of Data Warehouse Architectures
- #TDWI SURVEY SEZ: 79% say their #DataWarehouse has an architecture.
- #TDWI SURVEY SEZ: #EDW arch is evolving dramatically (22%), moderately (54%) or slightly (22%)
- #TDWI SURVEY SEZ: Driving #EDW arch evolution: #Analytics 57%, #BigData 56%, #RealTime 41%.
- #TDWI SURVEY SEZ: Driving #EDW arch evolution: BizPerfMgt 38%, OLAP 30%, UnstrucData 25%.
- #TDWI SURVEY SEZ: Driving #EDW arch evolution: competition 45%, compliance 29%, dep’ts 29%.
The Importance of Data Warehouse Architectures
- #TDWI SURVEY SEZ: Architecture extremely (79%) or moderately (19%) important to #EDW success.
- #TDWI SURVEY SEZ: #EDW Architecture is an opportunity (84%), not a problem (16%).
Benefits and Barriers for Data Warehouse Architecture
- #TDWI SURVEY SEZ: Stuff that benefits from #DWarch: #analytics, biz value, data breadth.
- #TDWI SURVEY SEZ: Barriers to #DWarch success: skills gap, sponsorship, #DataMgt, funding.
Multi-Platform Data Warehouse Environments
- #EDWarch trend: more standalone platforms: #analytics DBMSs, columnar, appliances, #Hadoop, etc.
- As #EDW workloads get more diverse, so do types of standalone data platforms in #EDW environment.
- As types and numbers of data platforms grow in DW environs, architecture gets ever more distributed. #
- Distributed #EDWarch is good&bad: provides workload optimized platforms. But may spawn data silos.
- Logical layer of #EDWarch more important than ever to unite big design across multi data platforms.
Single-Platform versus Multi-Platform DW Architectures
- #TDWI SURVEY SEZ: Totally pure #EDWarchs are rare. Only 15% have central monolithic #EDW.
- #TDWI SURVEY SEZ: Hybrid #EDWarchs are most common today = central #EDW + a few other data platforms (37%).
- #TDWI SURVEY SEZ: 2nd most common Hybrid #EDWarch = central #EDW + many other data platforms (16%).
- #TDWI SURVEY SEZ: Sometimes #EDW plays small role in #EDWarch compared to workload platforms (15%).
- #TDWI SURVEY SEZ: Some organizations (15%) have many workload-specific data platforms, but no true DW.
Big Data’s Influence on Evolving DW Architectures
- #TDWI SURVEY SEZ: 41% will extend existing core #EDW to handle #BigData.
- #TDWI SURVEY SEZ: 25% will deploy new data platforms to handle #BigData.
- #TDWI SURVEY SEZ: 23% have no strategy for their #EDW’s architecture, though they need one.
- #TDWI SURVEY SEZ: Only 6% feel they don’t need a strategy for their #EDW’s architecture.
Reports and Analytics have Different DW Architecture Needs
- Many users preserve #EDW for reporting, BizPerfMgt & OLAP, but take #analytics data elsewhere.
- Data prep for reports differs from same for #analytics. So, many users prep data on separate platforms.
Want to learn more about evolving data warehouse architectures?
For a more detailed discussion—in a traditional publication!—get the TDWI Best Practices Report, titled Evolving Data Warehouse Architectures in the Age of Big Data, which is available in a PDF file via a free download.
You can also register for and replay my TDWI Webinar, where I present the findings of the TDWI report Evolving Data Warehouse Architectures in the Age of Big Data.
Posted by Philip Russom, Ph.D. on April 15, 20140 comments