Data Warehouses and Data Lakes: Why You Need Both
Big data by itself doesn't mean big value.
- By Barry Devlin
- March 7, 2016
I imagine that long-time users, supporters, and vendors of data warehousing resonated with my first TDWI Upside article Data Warehousing: Thirty Years Old and Still Delivering Value. Denizens of data lakes and believers in big data may, on the other hand, have been bemused: isn't data warehousing so last century?
One aspect of this problem is that marketing demands unending novelty, but I believe the real issue is that functionality and technological implementation are regularly confused. This problem is rampant in all aspects of IT and data management but is particularly evident in the dilemmas of big data vs. traditional data and data lakes vs. data warehouses.
After almost a decade of market focus on the big of big data, the past couple of years saw an increasing interest -- unsurprisingly -- in the challenge of getting real value from it. In particular, we saw a string of reports about data scientists spending 80 percent of their time on data preparation and only 20 percent on valuable research. One example: according to Monica Rogati, vice president for data science at Jawbone, in a mid-2014 New York Times article, "Data wrangling is a huge -- and surprisingly so -- part of the job. ... At times, it feels like everything we do."
The industry, of course, sprang into action to produce tools that reduce the pain and increase the percentage of real work. Those of us with longer memories recall that we've been here before. This is not a problem of big data alone. It is, rather, an issue of data reconciliation, an issue that raises its head whenever data comes from multiple, disparate sources.
Data reconciliation functionality is central to data warehousing, in the instrumented collection of data (via ETL, for example), in its structuring (via modeling tools), and in its storage (via RDBMS, usually). This combination of functionality facilitates the consolidation of disparate data into one physical store, which is the most useful way of creating data consistency. I believe it to be the only viable way.
However, what was never explicitly stated was that only core data had of necessity to go through the enterprise data warehouse (EDW) because it is that data that literally holds the key to consistency. In traditional data warehousing, all of the business data could normally fit there and so it was pushed through the EDW by default. With big data, this is neither possible nor desirable, but with most depictions of data lakes, the baby has been thrown out with the lake water: there is no formal concept of or place for a store of core, reconciled data.
Whatever we choose to call it in marketing terms, a modern data management architecture demands multiple data stores -- I call them pillars -- optimized for the different storage and processing needs of different data types. I use the word pillars to differentiate pillars from the layers of a traditional data warehouse and to distinguish them from silos to emphasize the need for consistency across the pillars. This consistency is instantiated (in part) by a single core business information repository, as shown in Figure 1, where key data is reconciled and provides the links between data in different pillars. For explanations of the other terms in this figure, please refer to my book, Business unintelligence.
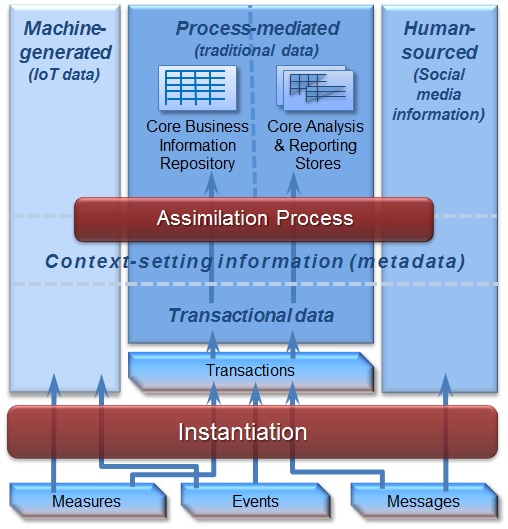
Figure 1: Pillars and the core business information repository.
Figure 1 is a logical architecture. It emphasizes some of the functionality required in a modern data management environment to support big data and traditional data, reporting and analytics, exploration and production. Without using either of the terms, it locates and explains both the data warehouse and the data lake. I far prefer both/and to either/or.
About the Author
Dr. Barry Devlin is among the foremost authorities on business insight and one of the founders of data warehousing in 1988. With over 40 years of IT experience, including 20 years with IBM as a Distinguished Engineer, he is a widely respected analyst, consultant, lecturer, and author of “Data Warehouse -- from Architecture to Implementation" and "Business unIntelligence--Insight and Innovation beyond Analytics and Big Data" as well as numerous white papers. As founder and principal of 9sight Consulting, Devlin develops new architectural models and provides international, strategic thought leadership from Cornwall. His latest book, "Cloud Data Warehousing, Volume I: Architecting Data Warehouse, Lakehouse, Mesh, and Fabric," is now available.