Page 2 of 3
The Rise of Data Science
By David Champagne, Chief Technology Officer, Revolution Analytics
Every trend generates a countertrend, and the field of data analytics isn't immune to this phenomenon. For the past five years, the field has been steadily democratized by the astonishingly rapid emergence of exciting new tools and technologies for crunching through increasingly large mountains of data. The availability of all this "new gear" has made some aspects of data analysis seem easier, or at least less daunting. Therein lies the problem.
Back in the "good old days," data was the stuff generated by scientific experiments. Remember the scientific method? First you ask a question, then you construct a hypothesis, and you design an experiment. You run your experiment, collect and analyze the data, and draw conclusions. Finally, you communicate your results and let other people throw rocks at them.
Nowadays, thanks largely to all of the newer tools and techniques available for handling ever-larger sets of data, we often start with the data, build models around the data, run the models, and see what happens.
This is less like science and more like panning for gold. Several data analysts interviewed for this article describe the current trend as "throwing spaghetti against the wall and seeing what sticks." When you consider the paramount important of data analytics in virtually every human endeavor (finance, healthcare, telecommunications, manufacturing, travel, government, etc.), the sticky spaghetti image seems particularly unappetizing.
The countertrend is what Nathan Yau calls "the rise of the data scientist." Nathan is a Ph.D. candidate at UCLA. His area of concentration is statistics with a focus in data visualization, and he writes a popular blog, FlowingData. From his perspective, really good data analysis is more than merely a matter of crunching numbers. It's a blend of talents and specialties. Here's a snippet from Yau's seminal post on the subject:
Think about all the visualization stuff you've been most impressed with or the groups that always seem to put out the best work. Martin Wattenberg. Stamen Design. Jonathan Harris. Golan Levin. Sep Kamvar. Why is their work always of such high quality? Because they're not just students of computer science, math, statistics, or graphic design.
They have a combination of skills that not just makes independent work easier and quicker; it makes collaboration more exciting and opens up possibilities in what can be done.
Yau's post, along with posts in a similar vein from other bloggers, has sparked an energetic conversation in the data analytics community. Not all data analysts are entirely comfortable with the idea of being called "data scientists," but there is an emerging sense of agreement that to be genuinely effective, a data analyst must bring more to the table than a keen ability to analyze data.
At this point, it seems fair to ask: How are data scientists different from data analysts? Drew Conway, a former member of the intelligence community, is now a Ph.D. student in political science at New York University. He recently posted a Venn diagram (see Figure 1) that depicts his vision of the overlapping skill sets required for data scientists. In Conway's formulation, the major skill sets are:
- Hacking skills
- Math and statistical knowledge
- Substantive expertise
As Conway sees it, a true data scientist must "speak hacker." That certainly makes sense, because most data today exists as a sequence of ones and zeroes in a database. Data scientists don't have to be hard-core computer geeks, says Conway, but they do have to know their way around the IT landscape because that's where the data lives. Hacking abilities are important because data tends to reside in multiple locations, and in multiple systems. Finding and retrieving data sometimes requires the skills of a burglar -- even when the data is in the public domain, owned by your organization, or owned by another organization that has agreed to let you use it.
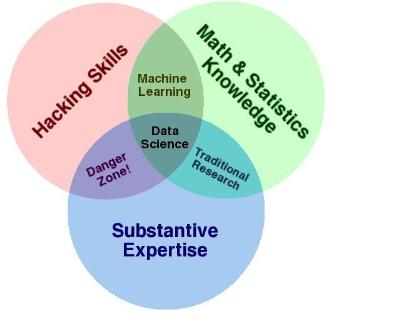
Figure 1: Skill sets for data scientists
Source: Drew Conway
Mike King, a quantitative analyst at Bank of America, says that even a great statistician can be stymied by the tangle of legacy systems at a large enterprise -- and that's why hacking skills are so crucial in a real-world environment.
"You need to be familiar with different databases, different operating systems, and different programming languages. Then you have to get those systems to communicate with each other," says King. "You must learn where and how to extract the data you need, and you better enjoy the process of figuring it out. This is not a trivial process, especially in a large organization. You need to be resourceful and create your own solutions."
In addition to good hacking skills, a data scientist needs domain expertise. "You have to understand the business so you can communicate effectively with the people around you," says King. "In the real world, these pristine, textbook data sets are scarcely existent out of the box -- you have to build your own. That requires talking to a lot of people in the business and explaining what you need in clear terms, not tech-jargon."
Michael Elashoff is director of biostatistics at CardioDx, a cardiovascular genomic diagnostics company in Palo Alto, CA. The company fuses expertise in genomics, biostatistics, and cardiology to develop clinically validated genomic tests that aid in assessing and tailoring care of individuals with cardiovascular disease, including coronary artery disease (CAD), cardiac arrhythmias, and heart failure. Elashoff says the term "data scientist" is really "more of an acknowledgement that people in this field need multiple types of expertise. It recognizes the fact that looking at data requires more than just analytic skill."
Conway's description of a data scientist "is very similar to what I do, but I still call myself a statistician," says Elashoff. "I need some knowledge of biology and computer programming to do my job, but I would never claim to know enough about biology to replace a biologist. I'm reasonably good at programming, but for some tasks you really need a programmer."
The Venn diagram "is useful, but I don't think that one person can do all of this," says Elashoff. An interdisciplinary team, however, could possess the skills depicted in the diagram. "It's pretty hard to be an expert in all these areas."
Perhaps the term "data scientist" reflects a desire to see data analysis return to its scientific roots. "I'm not a big fan of the spaghetti method," says Zubin Dowlaty, vice president/head of innovation and development at Mu Sigma, a global analytics services company. "It makes me nervous when people run a lot of analytic techniques just to get the answer they want, instead of being objective. Doing this job properly requires the rigor of a scientist. The scientist can see things that other people cannot see."
With or without a new moniker, the analytics industry is changing, says Dowlaty. "The trend is toward a multi-disciplinary approach to extracting value from data. It's not just about math anymore. You also need technology skills, but what ultimately separates the analyst from the scientist is the dimension of artistic creativity. It's the soft skills that make the big difference."
Michael Driscoll, author of the Dataspora Blog, holds a Ph.D. in Bioinformatics from Boston University and an A.B. from Harvard College. He has a decade of experience developing data platforms and predictive algorithms for telecom, financial, and life sciences institutions. From his perspective, the rise of data science represents the next logical phase of innovation in business intelligence.
"Think of it as a three-layer cake with data management at the bottom, BI software in the middle, and insights at the top," says Driscoll. "Data management is, increasingly, a solved problem. BI vendors could be facing a mass extinction. We're moving up the stack into the next phase, towards analytics, which drives products."
Whether you call it data analysis or data science, the future of this field looks brilliant and exciting.
David Champagne is the chief technology officer of Revolution Analytics, a provider of software and support for the popular open source R statistics language, and a software architect, programmer, and product manager with over 20 years experience in enterprise and Web application development. As principal architect/engineer for SPSS, Champagne led the development teams and created and led the text mining architecture team. He is a graduate of Southern Illinois University.