Choosing an Organizational Structure for Your AI Team
There's more than one way to staff an AI project depending on your situation. Here's how to find the one that will work best for you.
- By Wilson Pang, Alyssa Rochwerger
- May 3, 2021
More often than not, when a company wants to make a big impact with AI, they hire some data scientists and put them on their own team without any thought to organizational structure.
This is a mistake because it often leads to silos in which there's little cross-functional communication, leading to breakdowns in the effectiveness of the AI solutions.
A business problem that can be solved by an AI model alone is unusual. Most problems are multifaceted and require an assortment of skills -- data pipelines, infrastructure, UX, business risk analysis.
In short, machine learning products can't be developed by a team of data scientists alone. They require a team effort, and you need the team to work for the AI to work.
You need to carefully consider organizational structure. There are three primary structures to choose from, with the decision depending on your company's particular situation and needs: the star structure, the matrix structure, or the fully embedded structure.
The Star Structure
A "star" organizational structure may be the right choice for smaller companies or companies just starting an AI journey and that can't yet afford to build out AI throughout the organization. Instead, they'll create a centralized AI team -- basically, a center of excellence -- in charge of AI efforts for the whole organization. They'll work with all the domains and departments. Anyone with any AI needs will come to this team to get their model or application built.
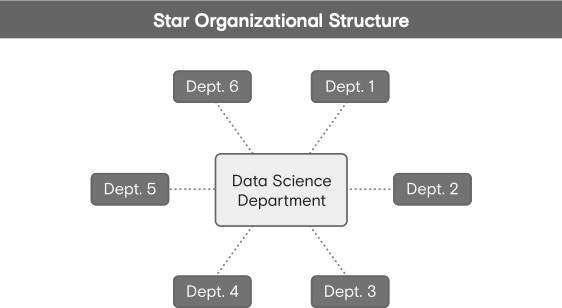
This structure is reasonably flexible. Its centralization allows AI efforts across the company to stay aligned, and it simplifies the allocation of limited resources.
However, once AI ramps up at the company, it'll be very difficult for a star-shaped AI organization to scale up to meet demand. Therefore, in many cases, this structure should be viewed as a temporary solution.
The Matrix Structure
A "matrix" structure is more appropriate for bigger companies that are more AI mature. They might have quite a few AI problems they want to attack in different domains or across product lines.
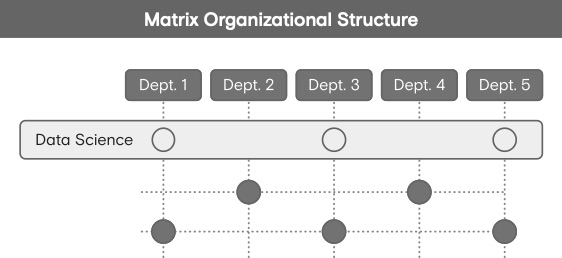
For example, a company in the travel industry might have a hotel product line, a flights product line, and a vacations product line. In a matrix-structured organization, the AI team members are dedicated to these individual problems -- two to three people working on the "hotels" product, a few on the "flights" product, and so on. Each subset would focus very closely on their business problem, working directly with the people from that domain.
Meanwhile, all these team members maintain their horizontal organization as part of the AI team, sharing ideas, learning new techniques, and comparing their domain-specific solutions to see how they can work together better.
The major benefit of a matrix organizational structure is its versatility. The enterprise can handle many problems across business domains simultaneously while allowing the right people to be brought to each one.
The drawback is that because each team member reports to managers in two dimensions, this structure can get extraordinarily complex as the number of individual problems grows. Managers in a matrix organization have to be very careful to make sure that responsibilities are clear so the AI team members don't feel they have to serve competing loyalties.
The Fully Embedded Structure
The largest and most mature companies can move to a fully embedded AI structure. These companies will have used AI for a long time; it might be a core piece of some or all of their products.
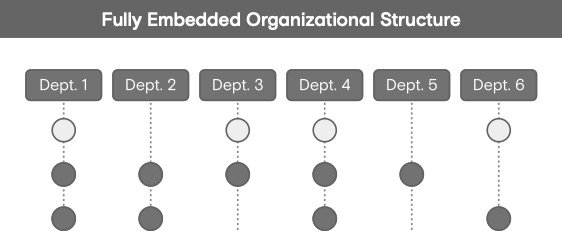
With a fully embedded organizational structure, every individual department will have its own data scientists and engineers who can build and deploy models on their own without reporting into a central organization. The machine learning team is just part of the domain team, and all the problems they work on are for that domain.
A fully embedded structure allows for great focus and cross-functional communication. With a fully embedded structure, it's easier to incorporate many AI solutions across the entire company.
The drawback is that when every domain has its own AI team working independently, coordination problems can develop. It's a good idea to maintain a level of horizontal communication between AI teams, similar to a matrix shape, to keep things consistent across the company.
Organizational Structure Matters
There's no one right way to organize your teams. In fact, there are many ways, and the one you choose will depend on where you are on your AI journey, your AI readiness, the sophistication of your team, the amount of talent you have, what your business objectives are, and how you measure success.
The important thing is to be strategic and intentional about the structure you choose. Building AI solutions takes the support of the entire organization, and by giving thought to organizational structure, you can ensure the kind of cross-functionality that will lead to smart, business-driven AI solutions.
[Editor's note: This article was adapted from a new book by the authors, Real World AI: A Practical Guide for Responsible Machine Learning.]
About the Authors
Wilson Pang joined Appen in November 2018 as CTO and is responsible for the company’s products and technology. Pang has over 17 years of experience in software engineering and data science. Prior to joining Appen, he was senior director of engineering at eBay in California and provided leadership to various domains including data service and solutions, search science, marketing technology, and billing systems. Pang obtained his master’s and bachelor’s degrees in electrical engineering from Zhejiang University in China. You can reach the author via LinkedIn.
Alyssa Rochwerger is a customer-driven product leader dedicated to building products that solve hard problems for real people. She has held numerous product leadership roles for machine-learning organizations. She served as VP of product for Figure Eight (acquired by Appen), VP of AI and data at Appen, and director of product at IBM Watson. Currently, she serves as director of product at Blue Shield of California, where she is happily surrounded by lots of data, many hard problems, and nothing but opportunities to make a positive impact. Alyssa holds a BA in American studies from Trinity College.