Analytics is Only One Scenario in Decision-Making Support
Focusing on the "simpler" forms of analytics might be as valuable as investigating the more complex analytics approaches.
- By Barry Devlin
- July 24, 2018
The fundamental starting point for any information architecture is recognizing the key characteristics of information used by the business. Such characteristics guide architects to decisions about how diverse types of information must be stored, managed, used, and -- eventually -- disposed of. In Business unIntelligence, I identified three characteristic axes of information: (1) timeliness/consistency, (2) structure/context, and (3) reliance/usage. Using these characteristics allows us to distinguish between data warehouses, lakes, and marts, for example, and to determine which information (or data) belongs in each.
In my review of "Thirty Years of Data Warehousing," I noted that architects and designers have long used similar classifications to define and design information management systems. However, a comparable classification system has long been missing for information delivery and usage systems. We have seen a plethora of conflicting and/or overlapping terminology over the past three decades: decision support systems, business intelligence, data discovery, analytics of various flavors, and machine learning. The result is a mystifying product landscape leading to confusion among business users about which tools and methods should apply in different decision-making activities.
One common attempt to make sense of this landscape conflates decision-making support with analytics and proposes that it evolves over time and with decision-making maturity within organizations. Stages include -- depending on the author -- descriptive (what happened?), diagnostic (why did it happen?), predictive (what might happen?), and prescriptive (making it happen). As shown in the figure below, this approach further suggests that as the business follows this evolutionary path, value increases, as does the complexity of the endeavor.
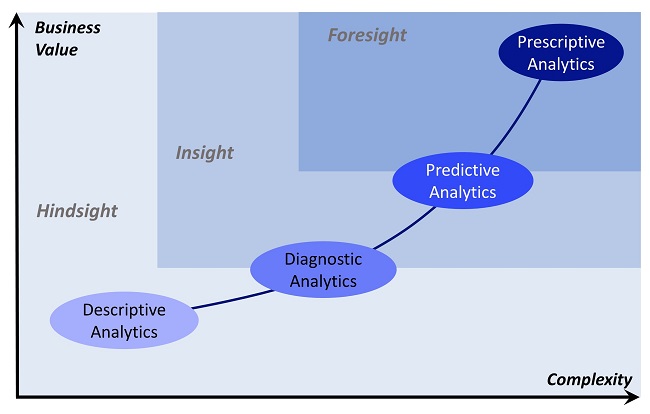
The evolutionary model of analytics.
Regarding such evolutionary models, thought leader Tom Davenport (a professor of IT and management at Babson College and a senior advisor to Deloitte Analytics) said, "A key with these multi-step models of analytics, however, is not to despair about how far you have to go, but simply to try to advance from where you are. ... The sooner we start employing more sophisticated analytics, the sooner we can get some real value from the Internet of Things."
Davenport's statement points to two fundamental misunderstandings of decision making that these evolutionary pictures may generate: (1) that all decision making is built on analytics and (2) that increasing value necessarily follows from moving up through putative stages of analytics evolution, with the corollary that businesses should strive to apply predictive or prescriptive analytics to maximize value in all cases.
Categorizing Information Delivery and Usage
A deeper look at the business goals of information delivery and usage systems and how information is used in support of these goals is instructive. It shows the intrinsic value of even the "simpler" forms of analytics and how focusing on improving them might be as valuable as investigating the more complex analytics approaches. The following categorization is the work of Martijn ten Napel and colleagues at Dutch co-operative Free Frogs.
Management begins with measurement in many cases. The two most fundamental use cases in information delivery and usage focus on measuring the business: monitoring and accountability reporting. Both involve tracking business measures against expectations and looking for exceptions and unusual occurrences. They differ in timing. Monitoring increasingly tends toward real time, while reporting emphasizes periodic accounting measures such as months or budget years. The former demands up-to-the-minute information; the latter is based on summary information integrated from multiple sources.
To call these use cases descriptive analytics distracts from their purpose and how they work. Once delivered, they should ideally remain largely the same, in order that business successes and failures, as well as their progress, can be quickly identified. Both scenarios are inherently passive and must be defined and optimized in their own right.
Only when exceptions are detected does monitoring demand immediate action or accountability reporting lead to longer-term or strategic changes. In both cases, (diagnostic) analysis is required to determine the appropriate responses. This use of information is active, seeking to understand causation and to determine the likely outcomes of proposed actions. Analysis here implies an investigation of well-defined, bounded, and available information with specific goals in mind. As in the case of the previous categories, analysis exists in its own right, without any need for "evolution" to a next, better stage.
Data exploration or discovery uses similar -- and more elaborate -- techniques as analysis but in a more free-flowing manner as the basis for innovation or identification of unexpected threats or opportunities. This scenario has very different business drivers than analysis and demands diverse and highly contextualized information across broad swathes of the business process. It often requires significant collaborative effort among data scientists and holistic business experts to achieve results, in contrast to analysis, which is usually a more solitary pursuit by a subject matter expert.
Finally, Data exploration or discovery is, as the name clearly says, the process of using information about the past and present to predict or forecast likely future events, outcomes, or behaviors using statistical or machine learning models. Prediction is a natural business follow-on to monitoring, whereas data exploration is more closely related to accountability reporting both in terms of information needs and timing. In some cases, prediction may enable prescription -- action to influence future outcomes. However, the phrase prescriptive analytics is now seen to be a misnomer: action follows but is separate from analysis.
BI practitioner and blogger Martijn ten Napel takes these categories forward to discuss the implications for the interaction between producers and consumers of information. The detail is beyond the scope of this article, but the blog series, "The Complexity of Information Projects," provides valuable insights into how to use this categorization to achieve ongoing success in information delivery and usage projects.
A Final Word
The five categories I've listed offer a complete classification structure for all cases of information delivery and usage, similar to that in the three information axes defined in Business unIntelligence. I invite readers to explore other possible categories. Most important, they enable us to step back from evolutionary models of analytics and correctly ascribe value to the different types of decision-making support systems, from simple reporting to advanced analytics.
In many cases, a focus on getting reliable and repeatable results from the simpler information delivery and usage scenarios, such as monitoring and reporting will deliver early and valuable business results while the more complex predictive and prescriptive efforts still struggle to build the required infrastructure.
About the Author
Dr. Barry Devlin is among the foremost authorities on business insight and one of the founders of data warehousing in 1988. With over 40 years of IT experience, including 20 years with IBM as a Distinguished Engineer, he is a widely respected analyst, consultant, lecturer, and author of “Data Warehouse -- from Architecture to Implementation" and "Business unIntelligence--Insight and Innovation beyond Analytics and Big Data" as well as numerous white papers. As founder and principal of 9sight Consulting, Devlin develops new architectural models and provides international, strategic thought leadership from Cornwall. His latest book, "Cloud Data Warehousing, Volume I: Architecting Data Warehouse, Lakehouse, Mesh, and Fabric," is now available.