Welcome to TDWI FlashPoint. In this issue, we flash back to a column by Dave Wells that appeared in the March 22, 2007 issue. Dave discussed the problem of "manualytics" then and says it's as relevant today as it was a year and a half ago. CONTENTS FlashPoint Snapshot FlashPoint Snapshots highlight key findings from TDWI's wide variety of research. Which term does your group use? 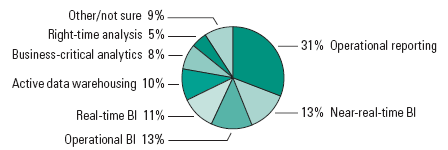 Based on 423 respondents. Source: Best Practices in Operational BI: Converging Analytical and Operational Processes (TDWI Best Practices Report, Q3, 2007). Access the report. Top FlashPoint FlashBack From Manualytics to Analytics Dave Wells, Independent Consultant Manualytics? I guess it means analytics the hard way. The term (and the inspiration for this article) comes from my coworker and friend Jennifer Hay. On completion of an especially labor-intensive job to provide me with a handful of statistics, she suggested that "we really have to find a better way than manualytics." The Nature of Manualytics What is manualytics? It is getting the numbers to support analytic processes through a lot of manual efforts—to find the data that you need, to get data from several different sources, to standardize and consolidate that data, to correlate the data, and to present it to the person who requested it. Manualytics typically involves one person working at a computer to collect and consolidate data and to create tables and charts. This person is not so much a business analyst as a business manualyst. The manualyst begins with a concept of how to create the desired numbers and then seeks the data to support that concept. Some of the needed data may be found in a data warehouse or downloaded from operational systems and databases. Where data isn't readily available in trusted systems, the next step is to copy and paste from other spreadsheets. A seasoned manualyst has quite a collection of spreadsheets to rely upon and will commonly also use data from the spreadsheets of colleagues. On occasion, the solution may be the manual entry of data found in a report or on a Web page. The Problem with Manualytics Does this sound like something that you have observed or experienced in your own job? I believe that manualytics occurs in every business and every organization. Regardless of your organization's analytic maturity, someone somewhere is handcrafting tables and charts in Excel. I don't deny that spreadsheets are a useful tool to meet one-time needs for information. But the downside is that all too often we apply manualytics to recurring and redundant information needs, reinventing the process each time a need occurs. And that's only the beginning. There are many potential problems of one-off analytics: - Inefficient processes and unnecessary work—Manualytics is handcrafting, whereas analytics at their best are derived through repeatable processes. Reinventing the processes with each cycle is labor-intensive—from problem definition, through design, and into implementation.
- Inconsistent results—If you're comparing numbers from different points in time, the accuracy is suspect when data sources, data cleansing methods, algorithms to derive information, and graphing techniques have been recreated with each cycle of analysis.
- Compounding of errors—When data from one spreadsheet is used to populate columns in another, any inaccuracy or imprecision from the source data is naturally propagated into the target data. Applying derivations in the target spreadsheet then hides the errors, so that imprecise and incorrect data is accepted as factual. A snowball effect occurs when today's target data becomes tomorrow's source data.
- Inability to trace from target to source—A typical manualytics process is one of trial and error until the desired result is achieved. The result is an undocumented set of numbers that cannot be readily verified. When the manualyst is asked, "Where did you get these numbers?" he or she will find it difficult to respond with certainty. This is true almost immediately after tables and charts are produced—and increasingly so as time passes.
- Uncertainty and confusion—At some point, the product of manualytics will inevitably be correlated or compared with results from source systems or from formal analytics. They certainly will tell different stories. Manualytics erodes the frequently stated goals of BI: trust in the data, confidence in decision processes, and a single version of the truth.
- Risk—When the results of manualytics are used to make business-critical decisions, and especially when they are applied in audit-sensitive areas such as regulatory compliance, there is a real and substantial risk. Untraceable data and undocumented processes are contrary to sound compliance practices and certainly are not ideal when making important business decisions.
Lemons to Lemonade Despite these issues, the practice of manualytics is inevitable. I believe not only that it occurs in every business and every organization but also that it will continue to occur. This is true for two simple reasons: (1) When given answers, we will find new questions; and (2) When given data, we will load it into spreadsheets. The challenge, then, is not to eliminate manualytics but to evolve them. When manually produced tables and charts are viewed as nascent analytic needs and prototype solutions, they become opportunities instead of problems. A basic process to turn manualytic lemons into analytic lemonade is illustrated here:  Identify by finding and cataloging the manualytic activities and results that exist throughout your organization. It is unlikely that you will find all instances, so concentrate your efforts on those that occur repeatedly. These are the easiest to find and the most likely to present real opportunities. Standardize by looking at multiple occurrences to determine which elements—data sources, algorithms and formulas, charting techniques, etc.—are similar and which are different between occurrences. Accept the similarities as the basis of standard analytic requirements, and then resolve the differences to define requirements for which a repeatable solution is practical. Consolidate the highly similar requirements that you are likely to find throughout large organizations into aggregate requirements that represent combined needs. Seek out the best data sources and integrate that data into your analytic environment. Finally, design and automate the analytic processes to satisfy the requirements. The Cycle Continues The obvious results of this process are that you will discover real analytic needs that are being satisfied through manual processes, and you will deploy production-quality analytics to meet those needs. Perhaps less obvious is the impact of the newly deployed analytics. As part of a production analytic environment, they are likely to reach new audiences. You’ll give answers, and people will find new questions; you’ll provide data, and they’ll load it into spreadsheets. That will spawn new manualytics—and new opportunities. Dave Wells is a consultant, mentor, and teacher in the field of business intelligence. He focuses on strategic, functional, and organizational alignment as the keys to building and sustaining valuable and high-impact BI cultures and systems. Top FlashPoint Rx FlashPoint Rx prescribes a "Mistake to Avoid" for business intelligence and data warehousing professionals from TDWI's Ten Mistakes to Avoid series. Ten Mistakes to Avoid When Selecting and Deploying ETL Tools Mistake 4. Discounting Low-Cost or Free Options It’s important to evaluate project needs realistically. Many organizations buy expensive products without considering whether a cheaper tool would be easier to use, faster, or more cost effective. Unlike many other technology products, prices have not dropped significantly on ETL tools over the last few years. Products tend to be clustered at two ends of the cost spectrum: large ETL suites that carry a high, six- or seven-figure price tag, and more narrow, ETL-only products that cost considerably less. Oracle and Microsoft have recently entered the market with ETL products that are bundled with the database or are available at very low cost. These products provide a high degree of functionality, so don’t discount their value. “Is a large ETL suite overkill?” This is a question you should ask early in the evaluation process. High-priced, full-featured products are great if you exploit all the features, but they are overly complex and inefficient tools if you use only a portion of what they offer. On the other hand, if you buy a product because it’s less expensive, you run the risk that it won’t meet all of your needs. If it doesn’t meet your needs, you’ll end up paying developers to write code to do what the product can’t, buying supplemental products, or at worst, you might end up with shelfware. This excerpt was pulled from the Q2 2006, TDWI Ten Mistakes to Avoid series, Ten Mistakes to Avoid When Selecting and Deploying ETL Tools, by Mark Madsen. Top
|