Welcome to TDWI FlashPoint. In this issue, Arkady Maydanchik discusses the benefits of building and using a data quality scorecard. CONTENTS - FlashPoint Snapshot
Best Practices in Operational BI: Converging Analytical and Operational Processes - Column
Building and Using a Data Quality Scorecard - FlashPoint Rx
Don't Rely on Source System Data Accuracy FlashPoint Snapshot FlashPoint Snapshots highlight key findings from TDWI's wide variety of research. What is the status of your operational BI environment? 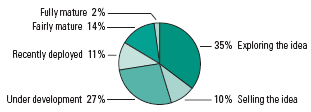 Based on 423 respondents. Source: Best Practices in Operational BI: Converging Analytical and Operational Processes (TDWI Best Practices Report, Q3, 2007). Access the report. Top Building and Using a Data Quality Scorecard Arkady Maydanchik, Data Quality Group Data Quality Scorecard Defined A data quality scorecard is the centerpiece of any data quality management program. It provides comprehensive information about the quality of data in a database and allows both aggregated analysis and detailed drill-downs. A well-designed data quality scorecard is the key to understanding how well the data supports various reports, analytical and operational processes, and data-driven projects. It is also critical for making good decisions about data quality improvement initiatives. A common misconception is that the objective of a data quality assessment project is to produce error reports. Such a view significantly diminishes the ROI of assessment initiatives. Project teams spend months designing, implementing, and fine-tuning data quality rules; they build neat rule catalogues and produce extensive error reports. Without a data quality scorecard, however, all they have are raw materials and no value-added product to justify further investment into data quality management. Indeed, no amount of firewood will make you warm in the winter unless you can make a decent fire. The main product of data quality assessment is the data quality scorecard! The image below represents the data quality scorecard as an information pyramid. At the top level are aggregate scores, which are high-level measures of the data quality. Well-designed aggregate scores are goal driven. They allow us to evaluate data fitness for various purposes and to indicate the quality of various data collection processes. From the perspective of understanding the data quality and its impact on the business, aggregate scores are the key piece of data quality metadata. In the middle are score decompositions and error reports that allow us to analyze and summarize data quality across several dimensions and for different objectives. Let's consider these components in more detail. 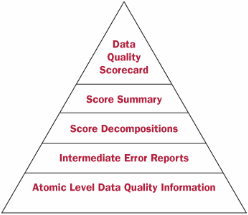 Aggregate Scores On the surface, the data quality scorecard is a collection of aggregate scores. Each score consolidates errors identified by the data quality rules into a single number-a percentage of good data records among all target data records. Aggregate scores help make sense out of the error reports produced in the course of data quality assessment. Without aggregate scores, error reports often discourage, rather than enable, data quality improvement. Be careful when choosing which aggregate scores to measure. Scores that are not tied to a meaningful business objective are useless. For instance, a simple aggregate score for the entire database is usually rather meaningless. Suppose we know that 6.3% of all records in the database have some errors. So what? This number does not help me if I cannot say whether it is a good or bad value, and I cannot make any decisions based on this information. On the other hand, consider an HR database that is used to calculate employee retirement benefits, among other things. If you can build an aggregate score that says 6.3% of all calculations are incorrect because of data quality problems, such a score is extremely valuable. You can use it to measure the annual cost of data quality to the business through its impact to a specific business process or to decide whether to initiate a data-cleansing project by estimating its ROI. It is possible—and desirable—to build many different aggregate scores by selecting different groups of target data records. The most valuable scores measure data fitness for various business uses. These scores allow us to estimate the cost of bad data to the business, to evaluate potential ROI of data quality initiatives, and to set appropriate expectations for data-driven projects. In fact, if you define the objective of a data quality assessment project as calculating one or several of such scores, you will have a much easier time finding sponsors for your initiative. Other important aggregate scores measure quality of data collection procedures. For example, scores based on the data origin will provide estimates of the quality of the data obtained from a particular data source or through a particular data interface. A similar concept involves measuring the quality of the data collected during a specific period of time. It is usually important to know if the data errors are historic or were introduced recently. The presence of recent errors indicates a greater need for data collection improvements. Such measurement can be accomplished by an aggregate score with constraints on the timestamps of the relevant records. To summarize, analysis of the aggregate scores answers these key data quality questions: - What is the impact of the errors in your database on business processes?
- What are the sources and causes of the errors in your database?
- Where in the database can most of the errors be found?
Score Decompositions The next layer in the data quality scorecard is composed of score decompositions, which show contributions of different components to the data quality. Score decompositions can be built along many dimensions, including data elements, data quality rules, subject populations, and record subsets. For instance, in the previous example we may find that 6.3% of all calculations are incorrect. Decomposition may indicate that 80% of these errors are caused by a problem with the employee compensation data; in 15% of cases the reason is missing or incorrect employment history; and in 5% of cases the culprit is invalid birth date. This information can be used to prioritize a data cleansing initiative. Another score decomposition may indicate that more than 70% of errors are for employees from a specific subsidiary. This may suggest a need to improve data collection procedures in that subsidiary. The level of detail obtained through score decompositions is sufficient to reveal the source of most data quality problems. However, if we want to investigate data quality further, more drill-downs are necessary. The next step would be to produce reports of individual errors that contribute to the score (or sub-score) tabulation. These reports can be filtered and sorted in various ways so that we can better understand the causes, nature, and magnitude of the data problems. The bottom of the data quality scorecard pyramid represents reports showing the quality of individual records or subjects. These atomic-level reports identify records and subjects affected by errors and could estimate the probability that each data element is erroneous. Summary The data quality scorecard is a valuable analytical tool that allows us to measure the cost of bad data to the business and to estimate ROI of data quality improvement initiatives. Building and maintaining a dimensional time-dependent data quality scorecard must be one of the first priorities in any data quality management initiative. Arkady Maydanchik is a recognized practitioner and educator in the field of data quality and information integration. A cofounder of Data Quality Group LLC, he is the author of Data Quality Assessment (Technics Publications LLC, 2007). Top FlashPoint Rx FlashPoint Rx prescribes a "Mistake to Avoid" for business intelligence and data warehousing professionals from TDWI's Ten Mistakes to Avoid series. Ten Mistakes to Avoid When Planning Your CDI/MDM Project Mistake 4. Relying on Source System Data Accuracy If you’ve had any involvement with your company’s enterprise data warehouse, you’ve probably encountered the challenge of operational system accountability: that is, convincing source system owners that it’s their job to address data quality. CDI technologies allow the merging of content from multiple sources to create a master record about a customer. While any data quality tool can correct a customer address, it can’t identify and resolve duplicate or disparate records and reconcile them into one when subordinate attributes are different. The quality of the master record is not dependent on the accuracy of the data from an individual source system, since the CDI or MDM technology can spot synonyms, duplicates, and errors in the source data. For instance, when an operational system has duplicate customer entries because of inconsistent descriptive detail (for instance, the customer goes by both “Bob” and “Robert,” or has different home addresses), it can selectively match other details to determine which descriptive attribute is best to include in the master record. The good news about CDI is that the hub can identify unique customers without affecting the day-to-day development activities of operational system programmers. When the time comes and the operational system team decides to correct its data, it can leverage CDI to identify duplicate or disparate customer records. This excerpt was pulled from the Q3 2006, TDWI Ten Mistakes to Avoid series, Ten Mistakes to Avoid When Planning Your CDI/MDM Project, by Jill Dyché and Evan Levy. Top
|