Breaking through with Business Process Discovery
BPA is intended to increase efficiency and effectiveness by analyzing current business processes and identifying their shortcomings.
- By Christopher Houck
- December 13, 2007
Abstract
Information technology provides an important way for organizations to improve business process inefficiencies. Most use some form of business intelligence (BI) to plan and implement their process improvement initiatives, making BI applications more and more entrenched with other applications such as business process analysis (BPA).
BPA is intended to increase efficiency and effectiveness by analyzing current business processes and identifying their shortcomings. This information can then be used to design new business processes that fit both the current and future needs of the organization. Manual interview and discovery methods used to analyze processes are usually costly, take a great amount of time to complete, and introduce a high percentage of human error. People tend to report what they think they’re doing rather than their real actions. This leads to the development of a flawed or inaccurate model of the situation. With an inaccurate process map and poor idea of the process to be improved, many process improvement projects fail.
Businesses can find analytic success through business process discovery (BPD), a new concept that automates and eliminates the human element. BPD provides the intelligence needed at all levels of an IT organization by making sense of the data reported from BI and process analytics tools.
In this article we will explain: - How BI is increasingly ingrained with other applications
- Why current process improvement initiatives are producing incomplete results
- How process analytics can yield broader analytic sets
- The mechanics of business process discovery
- How process discovery relates to and complements BI and process analytics
Discovering the Business Process
Every major initiative an organization undertakes requires a positive impact. The enterprise will spend resources (time, people, and money) on a project that will deliver a benefit and have a return on investment (ROI). This ROI is often related to improving a business process, such as improving the sales process to increase revenue or improving a customer service process to decrease costs.
Business intelligence is a powerful tool to assist in business process improvement initiatives. BI solutions help companies achieve real benefit by providing data and analytics to analyze and control business processes. In addition to providing the raw data, implementing BI as part of the process can make the process itself more efficient. However, in this process improvement context, there are some important caveats that must be explored before declaring BI to be the silver bullet.
An analogy will help illustrate a key difference between the way medical science and an IT department would approach a process improvement initiative. Both physicians and project managers (PMs) start with a vague description of the “problem” needing improvement or resolution. The physician and PM both go through a structured interview process to diagnose the problem.
It is here, however, that the methodologies traditionally deviate. Whereas the PM will usually jump to immediately prescribing a solution, the well-trained physician will undertake the scientific method of hypothesis testing through data analysis before prescribing a course of action.
For example, one study showed that lung clots (pulmonary embolisms) were correctly diagnosed only 30 percent of the time but, when combined with a CT scan to confirm the hypothesis of a diagnosis, 99 percent of the cases were diagnosed accurately.
By failing to conduct an automated test to validate the hypothesis developed during the manual interview process, the corporate PM is introducing a high percentage of human error into the solution. Indeed, BI is used by many organizations to provide the data to support the hypothesis, and there are a number of analytical methodologies (such as Six Sigma) that are based on this scientific principle of objective data analysis. Business process discovery (BPD), however, is a new concept that automates and eliminates the human element, and this is where businesses can find analytical success. BPD provides the intelligence needed at all levels of an IT organization by making sense of the data reported from BI and process analytics tools.
At an abstract level, BI solutions can be thought of as the combination of a data repository, a set of tools to visualize the data, and analytical routines and workflows to achieve some analytical function. While BI is an established and mature technology set, it is still experiencing exciting and dramatic innovation across the board. At the data level, the introduction of unstructured data, streaming data sources, and distributed or virtual repositories is expanding the potential for the scope of the analysis. Data visualization continues to be an area where we see the trend from static flat reports to graphical, real-time interactive displays of the information in forms that are intuitive to the user. Analytical techniques continue to be developed and refined, and are being woven into workflows that are now providing analytical applications to allow a wider community of users to leverage the power of BI.
Consider an example of BI in action at a hypothetical retail bank. This large bank has a tremendous amount of data and many skilled analysts who are highly motivated to increase the revenue associated with their particular service offerings. The service manager wants to cross-sell a new service to existing customers who call in to the service center, but the call center manager is concerned about extending the average call time and the additional call center staff that may be required. Rather than relying only on gut instinct and making a decision, the manager carries out an analytical study using the bank’s BI solution, which houses, among other things, information about what services every customer uses, as well as cost data on the call center’s operations. Using a combination of statistical tools and analysis, some highly skilled statisticians, and a month of extensive analysis, the team discovers the characteristics of the customers that make the probability of up-selling a new service worth the extra cost (in call center time).
Over the past decade, we have generally seen BI move from a relatively static, centralized data warehouse and reporting function used by a small set of executives and highly skilled analysts, to more real-time, interactive analysis woven into operational workflows.
Moving from Strategic to Operational
By adding a workflow and “best-practice” use of appropriate analytical and statistical techniques, BI has moved from being used for enterprise reporting and strategic analysis to improving operational business processes. Whereas the results of analytical analysis from BI tools may be used to change operational processes (as our previous example shows), analytical applications enable a broader audience to use the prepackaged analysis more frequently for operational decisions.
These analytical applications combine all of the elements of BI (data, visualization, analytics, and workflow) to provide a solution to a specific operational process. While these analytical applications theoretically retain all of the power of BI, in practice, the data is limited to what is required for the scope of the operational process. This limits the potential for ad hoc analysis, but also constrains its use and limits the potential for misinterpretations.
To illustrate this point, let’s revisit our retail bank and consider the overworked call center manager who has to maintain service levels while keeping costs at a minimum with the added complexity of dynamic call scripts based on our up-sell and cross-sell analytics. The manager has the difficult task of determining appropriate staffing of the center across skill sets. Our well-educated analysts come to the rescue and put together a forecasting solution for our manager that combines historical workloads with an appropriate forecasting methodology and a queuing model to predict service levels by staffing level. Our call center manager now has an analytical application that can determine staffing levels. By providing this information, the BI investment has again delivered real benefit to a business process, this time as an operational process of determining shift-staffing levels.
Defining the Process
Before any process-improvement initiative begins, however, it is fundamental to understand the definition of a business process. A business process is a clearly defined set of activities and a set of well-defined relationships between those activities that identify appropriate ordering and sequencing of behaviors to achieve the goal or output of the business process. While there are many ways to depict a business process, a common mechanism is to draw the actions and use directional arrows to indicate the order in which they occur.
Referring to the retail bank example, examine the call-center business process (Figure 1). If only three different call processes occur—updating accounts, checking balances, and transferring funds—the business process is simple. The agents wait for a call and then process the call depending on what service the customer wants. To build the appropriate analytical application to perform forecasting, the correct business process and metric process must be known to ensure that data is collected on the service offering (Figure 2).
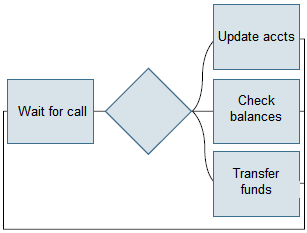
Figure 1. The call-center process
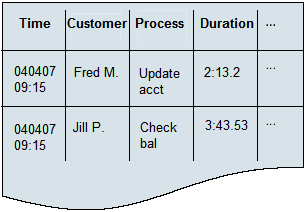
Figure 2. A transaction record
Business activity monitoring (BAM) solutions are available that take these kinds of metrics and provide the ability to create a dashboard for the process, as well as interrogate data to drill in to finer levels of granularity. This provides a view of the health of the business process and starts to bring a process control to the operational business processes. Furthermore, this allows BI to not only support the analysis and diagnosis phases of a business process improvement initiative, but also support the control and operation of a business process (Figure 3).
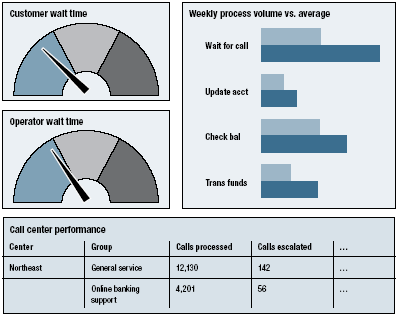
Figure 3. A call-center dashboard
For example, consider that the average call time starts to increase from three minutes to five minutes, greatly increasing the call center operations costs and increasing queue times for customers. By using this BAM solution, it is possible to see what processes are responsible for the slowdown and when the slowdown started to occur.
Significant assumptions were made, however, as this solution was explored. First, the process model for the call center was known. Second, the data to find the root cause was loaded into the BAM solution. To illustrate the first assumption, what would happen to the explanation if customers requested multiple services in the same session? Call times would increase—not because a single activity would take longer, but as the result of repeated services. The dashboards and metrics would show that, at a process level, everything was within bounds (other than the overall call length).
The second assumption presumes the data required was pulled from the process meter to provide the ability to discern a root cause to the issue. For example, consider the scenario in which the calls last longer because people are updating their accounts to take advantage of a new marketing program. The cost can then be attributed back to marketing, but unless this data element was included in the meter, it would not be reflected in the BAM solution.
At first glance, these two assumptions—known process model and limited data extracts from the process—seem innocuous enough. After all, the 80/20 rule is something people have used for a long time. If with these two assumptions an 80 percent solution is achieved, that should be good enough—but it’s not.
Business process improvement initiatives are often driven to optimize small exceptions to the normal business process. The 80/20 rule has worked so well that over the course of the last couple of decades, most businesses have optimized their core processes so that the process runs efficiently well over 80 percent of the time. However, the 20 percent of the time that it does not now represents the vast majority of the cost of the process.
Consider the insurance industry. Well over 80 percent of all insurance claims are processed without issue, but those 20 percent (or less) of claims that require intervention or adjudication represent an enormous cost. This holds true for many processes in most industries.
The implication to the BAM solution is that the process exceptions are the most interesting and important data points. In addition, the two assumptions both lower the chances that those exceptions will be discoverable using the solution.
Finding the Process
To eliminate this problem, if the two assumptions (a predefined process and limited data extracts from the process) are removed, a much larger value proposition emerges. First, examine the implication of starting without a process model and instead taking an emergent approach where the process model reveals itself from the data.
The initial benefits are clear. Many business processes are completely undocumented, and where documentation exists, it’s usually suspect. This problem is so well known that there are research reports written on the psychological reasons underpinning the poor accounting of business processes during interviews.
Even with the best of process documentation, the documentation represents only what the process is intended to do (and executes as expected a majority of the time). It is the unexpected process variations, or exceptions, that are driving costs, and they are not documented.
Consider the call center process (Figure 4). Upon investigation of the actual process instances and by reconstructing the process map, it becomes apparent that the call center employees are executing more than just their three processes. Rather unexpectedly, 6 percent of all the services being provided to the customers are transfers to technical support for customer questions about online banking. Because there is a special number and support service for online banking, we didn’t predict receiving online banking queries at the call center operations. By examining the data without an a priori notion of the process, however, that data is evident.
Figure 4. The actual call-center activity
Just as with the BAM solution, this process view provides a full set of process metrics. In contrast, by placing them directly in a process context, a picture of the natural flow of the business process is readily apparent. Looking at the process from this bird’s eye view makes it clear where the process is executing properly and where there are issues. Moreover, this fully quantified process view allows the manager to look for process exceptions based on subsets of activity that are executing differently.
For example, think about a very simple process such as retail shopping. Consumers buy items from a store periodically, and occasionally they return an item. Over all of one retailer’s customers, this simple process may look like Figure 5.
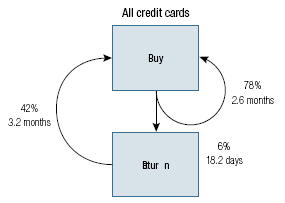
Figure 5. A return of merchandise purchased with a credit card
Now consider that a subset of customers is executing a very different process. Although the process boxes and arrows are the same, the relative percentage of times these customers return goods is much higher and always after just three or four days. By being able to examine the fully quantified process (shown in Figure 6) and examine subsets of process data, it immediately becomes apparent where the process exceptions lie, and in this case, these particular customers are delivering no revenue and driving up costs.
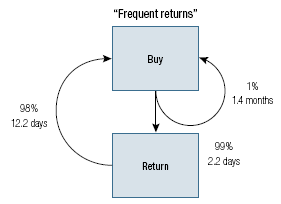
Figure 6. The frequent return of merchandise
There’s another important benefit from using an emergent approach. For example, instead of being concerned with the process from the call center’s perspective (that is, the business process of a call center employee), consider the business process of a customer. Using the same data, it is possible to look at the process from a customer’s perspective to understand how they are utilizing the call center’s services.
What leaps out in Figure 7 is that some of the customers are updating their account information again within the span of just a couple of days. This represents rework. Having identified the unexpected rework, the next step is to drill down and determine the root cause of this process variation.
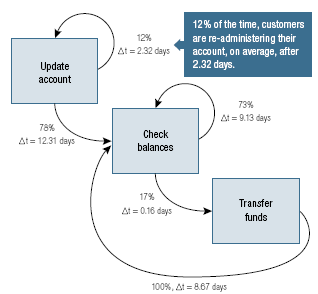
Figure 7. The same process from the customer's perspective
This leads to relaxing the second assumption: limited data being pulled from the process meter. To provide the best opportunity to discover the root cause of the process variation, we must have as much data as possible about each activity that has occurred during the course of the process. For most processes, the activity data could be well into the thousands of attributes; couple that with millions of process executions a day, and the data volumes become immense.
To make the challenge even more difficult, consider that the sources of this data are themselves constantly evolving. In our retail bank example, to fully understand the services the customer is using would require information from online banking, the automated voice recognition system, the internal applications the call center is using, and possibly additional systems. Each of these systems will evolve, presenting new attributes on a periodic basis, perhaps monthly.
The traditional data warehousing approach to store all of this dynamic and often-unstructured data is fraught with challenges. Beyond just storing the information, doing any meaningful analysis using tradition techniques (OLAP, data mining, etc.) is difficult given the dynamic nature of the metadata.
A new approach is needed to make sense of all of the data flowing through the business processes. By using a process model as the underlying mechanism to organize this information into a non-relational model, vast quantities of data can be effectively managed, analyzed, and dissected on the fly. The inherent analytical underpinnings of a process model provide a rich framework for performing advanced analytical techniques on the process data as well.
Business Process Discovery and Process Analytics
Business process discovery (BPD) is the term applied to this new approach for using unstructured, event-level data to automatically derive process definitions and explore process variations. This quantified process model allows for rich, interactive analysis. Users can quickly examine process variations between different groups of process activity. The retail bank may wish to examine the difference in customer activity based on the marketing classification of the customer (high-value, growth customer, etc.) to better understand which service combinations are best packaged into products for each market.
Even more exciting is the ability to use powerful statistical techniques on the resulting process models. Among these techniques are clustering, root-cause analysis, and predictive analytics. Our customer-returns example demonstrates the power of clustering. By examining the process in detail, groups of activities can be clustered and presented to the analyst for investigation—in this case, a behavior pattern emerges of customers returning a product more frequently than the norm. Looking for statistically significant process variations leads to the automatic discovery of process variations that are currently driving process costs.
Root-cause analysis leverages the vast amount of detailed data that is collected during process execution. By mining the data, process analytics can quickly discover meaningful patterns of data that predict the clustering of activity. In the retail banking example, it is easy to discover that the cluster of activity is for customers who are closing their accounts because all of the data associated with the process execution is known, so patterns can be detected to indicate the root cause for this occurrence.
Predictive analytics is an exciting field, especially when applied in a process context. With a process model coupled with the ability to automatically discover groupings of activity with root-cause analysis, you can predict changes in the behavior of processes as they are occurring. This provides the capability for the system to automatically provide the information and send alerts when a process becomes an undesirable exception. For example, for the retail bank, this could be an alert about a customer who has a high probability of closing his or her account based on their activity. Detecting and preventing this behavior can have a dramatic impact on the bank’s profitability.
Merging Discovery with Business Intelligence
BPD doesn’t replace traditional business intelligence solutions. Instead, it complements an existing BI implementation by providing a different, more expansive view of the business processes. The addition of a BPD solution often augments the value of the existing BI investment. The raw data consumed by BPD can be aggregated and delivered into a BI environment to quickly and easily augment existing data and analysis. Just as OLAP technologies augmented the relational views of the data that were in place, this new process-centric view of data augments and enhances a BI solution.
In many ways, BPD can be seen as both a new data source and an analytical engine to complement the BI stack. By combing through the large volumes of unstructured data, BPD can quickly refine and provide a set of aggregated, structured data feeds back into the BI environment. This allows the easy creation of BAM-like dashboards within the context of an existing BI installation.
In addition, BPD also provides the workflows for analysts and process improvement specialists to dig into the process details to explore and identify the variations that are draining value out of the business. Because BPD provides a means to quickly and easily discover process inefficiencies, BPD is being used to deliver on the vision of rapid, round-trip reengineering.
Round-trip reengineering refers to the general business improvement methodology in which short, incremental projects are undertaken to deliver real organizational benefits. An important criterion of round-trip reengineering is that the improvement is measurable from an established baseline. With BPD, that criterion is met automatically because the process improvement starts from a set of process data and associated metrics. After the improvement is made, the effect on the process metrics is immediately evident within the BPD solution. This takes the round-trip reengineering from diagnosis through delivery and validation of the treatment.
Perhaps one of the best things about BPD is that it’s easy to get started. BPD solutions work on unstructured data and without process descriptions, meaning that implementing a solution does not require extensive, tricky integration or lengthy business process analysis sessions. Most solutions must simply be pointed to the event source; they then perform the analysis on the resulting processes that emerge.
Getting started with BPD carries low risk. Due to the nature of the solution, it’s easy to start by looking at the events from a discrete set of IT assets that support a specific set of business processes. After a few quick, low-risk improvement initiatives prove the technology and approach, it is easy to undertake larger deployments.
BPD in Action
The first step in BPD is to identify the process. Once the process has been identified, it is usually easy to identify the IT assets that support it.
Once again, consider the retail bank scenario. The bank’s management team wants to understand the process their customers use to create a new account. While this sounds quite simple, the customers can interact with the bank via online banking (a Web site), through an interactive voice recognition system (IVR), or by calling the call center, where a customer service representative (CSR) will handle the call using a back-end (mainframe, greenscreen-based) system.
Having identified the process and the systems, the bank deploys their BPD solution to observe those technology assets, in this case the IVR, Web, and mainframe systems. While this process may vary slightly among the assets, the general principle is the same. The system observes all activity on the asset and automatically creates an application map after only a short observation period (at most a few days). In practice, this is often achieved by replicating the activity (e.g., Web traffic or terminal traffic) to a separate device that houses the “collector” for the BPD solution, or by streaming a detailed log file into a BPD collector. In this way, the installation is noninvasive and requires no logical integration.
At this point, a developer would go into the BPD solution and validate the definition of the processes. This is a quick exercise of defining the subset of all the workflows and processes embodied in the IT asset that are relevant for the process under consideration (Figure 8).
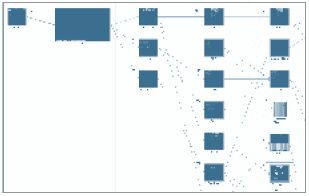
Figure 8. Complete workflow
For example, the retail bank’s CSRs and online systems allow for many more processes than just account creation. While at this point the developer is interested only in the account creation process (Figure 9), over time, many processes will be defined for each IT asset in an incremental fashion.
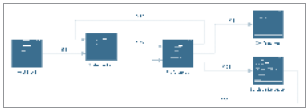
Figure 9. Account creation process
Once the process has been confirmed, the BPD system generates events for every instance of the process observed. At this point, the analyst can create a process perspective by simply choosing the account number as the unique identifier. Next, the system will automatically analyze all of the events, determining the process followed for every account number observed to date. The analyst can then view the resulting process map and start to interrogate the system to look for process variations by filtering using other data elements (Figure 10).
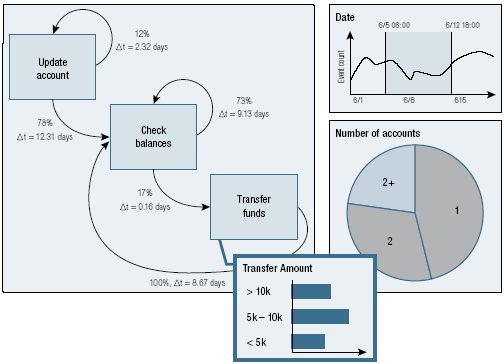
Figure 10. Adding the analysis
This provides a fully quantified powerful way of interrogating the process execution, easily combining all the touch points for the process. This entire process, from installation to process interrogation, often takes less than a week.
BPD Vendors
While BPD is a new solution, there are several different approaches and vendor communities all working toward BPD solutions (see Table 1). Principally, these vendors fall into three categories: the business activity monitoring (BAM) group, the business process management suite (BPMS) vendors, and the complex event process (CEP) vendors. Each of these groups is taking a different approach and tackling the problem slightly differently.
BAM vendors are the traditional vendors in this area, and they continue to focus on providing real-time process metrics and dashboards. As such, they continue to focus deeply on providing real-time exception alerting and on providing actionable information. The challenge for the BAM vendors continues to be overcoming their legacy of hard integration points to a predefined model of metrics.
Group | Sample of Vendors |
BAM | Systar, webMethods, oracle |
BPMS | Global 360, Pegasystems, IDS Scheer |
CEP | StreamBase, Aleri, TIBCO BusinessEvents |
Pureplay | OpenConnect |
Table 1: Vendors
BPMS vendors are working toward a vision of “closed-loop” business-process improvement so that after implementing a process using their BPMS engine, a BDP solution will be available to measure the process improvement. The challenge for BPMS vendors is to provide a solution that extends beyond their BPM solution to include all the touch points in a process, including external systems.
CEP vendors provide a new approach to examining the event streams emerging from existing systems. These systems are often built for extreme scalability, finding early adoption in real-time, financial trading systems where a massive amount of real-time data needs to be continuously processed to find and expose event conditions. Some of these vendors are using an interesting SQL analogy of a continuously running SQL query across a persistent data stream.
The challenge for CEP vendors is to move away from fixed, model-driven approaches to allow for more flexibility in event processing. This provides a business context where the stream of business events is more manageable than real-time data streams of tick-based trading systems.
A few pure-play vendors provide solutions that do offer a model-free view of business processes down to the event level, using events from any system in the IT stack. The challenge for these pure-players is to continue to provide systems that scale to the hundreds of millions of business level events, and provide for real-time, model-free interactivity to analyze and interrogate the resulting process.
Summary
BPD is an exciting new paradigm in BI that provides a new set of data and analytical techniques. Just as OLAP was exciting for hierarchical analysis, BPD provides a new, process-centric analysis on business process event data. BPD innovates across the span of BI, from the data layer through to new process visual analysis methodologies and into process analytics.
Most important, BPD provides the data and analysis capabilities to perform “round-trip” business-process improvement initiatives quickly and with low risk. No longer are process improvement teams working on ad hoc solutions for data collection, or worse, measuring activities with stopwatches. BPD provides the solution for quantified, analytical process improvement.
This article originally appeared in the issue of TDWI.