Welcome to TDWI FlashPoint. This issue presents Part One of a two-part column by Foster Hinshaw discussing how to maximize your data assets.
Maximizing Your Data Assets, Part 1
Foster Hinshaw, Dataupia
Data is to business as water is to fish
Data is at the core of every business. By following some simple best practices, you can gain additional insight from your data.
Pop-quiz time: Which of the following revolves around data? 1) Web 2.0 Widgets; 2) service-oriented architecture (SOA); 3) rich content services; 4) operational efficiencies. Answer: All of the above.
The fact that data is at the core of every business is nothing new. The first samples of human writing are records of transactions—written in stone. Now that’s an Information Lifecycle Management (ILM) strategy for you!
What is new today is the volume of data that every business, regardless of size, must manage. Every industry—including retail, financial, telecommunications, healthcare, e-commerce—collects vast amounts of data that is stored or archived but is often inaccessible in a meaningful timeframe. Let’s be clear—we’re not talking about the time it takes to load data from a tape. The timeframe opens when the business user requests information and closes when the information is delivered. No matter that it might take just 20 minutes to retrieve records from an optical storage disk. How long did it take to locate the disk, physically retrieve it, and mount it? If the data was in a backup format that no longer matched current models, how much time would that add?
Storing and archiving data is good, but not being able to access it in time for it to be useful is a big problem. The data warehouse appliance was introduced to alleviate the accessibility issue in a cost-effective manner. Its increased market adoption across industries has been well-touted. Many of the world’s leading Fortune 500 organizations are currently enjoying the benefits of the latest data appliance technology.
Data always contains valuable information—consumer buying patterns, cell phone call records, debit card usage, etc.—that provides immeasurable business benefit. One of the best examples of effective use of everyday data involves a personal experience I recently had with an online retailer. I phoned to order a sweater for my spouse and was told they were out of the color I wanted (green). I was about to hang up when the salesperson said, “Wait, I see your spouse also likes teal. Would you like to purchase a teal sweater instead?” Problem solved, crisis averted—because the retailer was able to access customer data to personalize my shopping experience. Similar examples can be found across many vertical markets as companies realize the power that exists within their data.
Clearly, collecting data to better serve or market to customers is one reason that businesses amass arsenals of information. Another notable driver is compliance. With more (and more stringent) regulations such as Sarbanes-Oxley, data collection is not just a nice-to-have but a must-have. Regardless of the specific reasons, the data is there and has tremendous potential if put to good use.
The Quest to Improve Accessibility
Evolution of the data warehouse appliance was driven by a quest to improve accessibility. There are two major barriers to accessibility of data: volume and complexity.
Before the data warehouse appliance, data warehouses relied on a combination of powerful database server hardware and some kind of attached storage (networked or direct). Some IT shops could afford massive machines that provided both. This architecture was one approach to accommodating the requirements of intensively computed complex queries and Big Data, but it wasn’t ideal. Computational resources were not what was needed. Working with Big Data poses a different kind of challenge: it puts a strain on I/O resources. The data warehouse appliance addresses this by matching CPU resources with adequate I/O channels.
Sheer volume of data creates other problems. First, there is a limit to the amount of direct attached storage an architecture can support, so even midsize data warehouses came to rely on storage that was available over the network. Now, we have the capacity to hold massive amounts of data, but that’s not enough. Unfortunately, we still have to move it around the network—from the storage servers to the database servers—and that creates performance issues, accessibility constraints, and administrative overhead. The data warehouse appliance resolved that issue by providing a machine that combined data processing and storage.
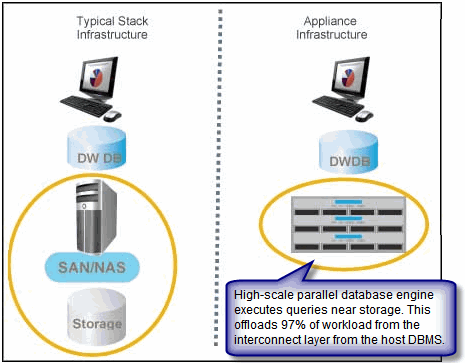
A data warehouse appliance simplifies data warehouse infrastructure by reducing the number of components (and vendors) and by offloading most of the query manipulation workload from the interconnect layer and from the host DBMS.
The second hurdle the data warehouse appliance sought to overcome was complexity, i.e., the difficulty in accessing data from a business-process perspective. In effect, complexity is a barrier to business agility and the ability to change with competitive opportunities and pressures. For example, less complexity enables IT to support a new sales/marketing campaign upon request and without the headache. Resolving the hardware, software, and connectivity issues doesn’t make data more accessible on its own. If the infrastructure is still difficult to work with, IT is still challenged to support the business’s need for data.
The complexity of the infrastructure is revealed during the initial deployment phase whenever IT migrates from one database platform to another, makes precision configurations, or masters new technologies. It follows that hard and soft costs increase in direct proportion to the degree of complexity. Complexity is an even more significant factor over the entire lifecycle of the data warehouse. Infrastructure inflexibility can cause an organization to calcify.
Early appliances, or "data warehouses in a box," fulfilled the strict definition of an appliance, i.e., they were built to a specific purpose. Certainly, a part of how we understand the term "appliance" is the sense that the appliance makes tasks easier to perform.
Data warehouse appliances are far simpler to install and maintain than a typical database and storage infrastructure. Most appliances do simplify day-to-day operating and administration, but upgrading hardware or software, changing the model or schema information, or adding more capacity can be resource-intensive and time-consuming. This overhead limits how quickly a business can have access to data, especially if some kind of transition is in play, such as a merger or acquisition, integration of new data sources, or interdependent technology.
Having mastered the formula for working with large amounts of data, we now see the data warehouse appliance moving into a new stage of development and focusing on streamlining complex infrastructure. Today, it’s not just the huge enterprises that have mountains of data; companies that do not have large IT groups also need to reduce complexity to be able to use their data effectively.
Currently, too many companies spend the greater part of their resources and time optimizing their data warehouse infrastructure and not enough time improving the data’s usage or usability. It’s time to reverse this trend by demonstrating how organizations can unlock their data and make it work for them. The latest evolution of data warehouse appliances does that by providing enterprises with deeper, universal access and uncovering the true potential of their data.
More Knowledge is Better
Data warehousing is all about how to get the most out of data. The term “data warehousing” seems somewhat unfortunate. Warehousing can call up the closing scene from Raiders of the Lost Ark where the Ark is being shelved in a government warehouse, surely never again to see the light of day.
The warehouse, according to Bill Inmon’s intention when he coined the term, is the heart of an operation. The data warehouse exists to facilitate the use of data.
Reality seems to take us in the opposite direction. To make the best use of the data warehouse’s costly resources, data is marshaled through information lifecycle management, formally or informally. Data is ranked in a number of ways such as currency, rate of access, and compliance. As companies become more aware of data as a business asset, those categories are starting to reflect business priorities as well among industry leaders.
No system is perfect, but as long as data earns a place in the sun based on how recent it is or how often it is accessed, its true value will never be exploited. Keeping only recent data online ensures a short-term picture and incomplete insight into the state of the business.
Classifying data according to frequency of access is even more of a dilemma. Typically, companies keep frequently used data online and less requested data near-line or offline. Consider, however, that it is precisely the data that is not frequently accessed that holds the potential for transformative information.
Data that is frequently accessed is probably caught up firmly within the routine operations of a company. The objective of much quasibusiness intelligence (aka reporting) is to help people understand the state of what has recently or is currently occurring in the business. That’s a required goal, but aside from maintaining the health of an organization, there’s growth to consider. A tried-and-true approach to growing a business is to change the status quo, and the information to support transforming decisions is more likely contained in the data that's not being looked at on a regular basis.
Which data is more valuable: the most recent or the most frequently accessed? The data captured by the finance department or that captured by customer service? Are these even the right questions? What companies should really ask is why they have to make the choice at all. Once the barriers to accessibility are overcome, why can’t all classes of business data be available online, so that companies can monitor and diagnose their operations as well as make game-changing moves?
In our next issue of FlashPoint, Part 2 of this column will discuss how you can bring data to the front line.
Foster Hinshaw brings a wealth of creativity as well as technical and operational expertise in both hardware and software to Dataupia, where he is CEO. Hinshaw has designed and developed large, complex systems for business-critical enterprise and departmental applications, as well as Web-based e-commerce systems. Prior to Dataupia, Hinshaw founded Netezza, an enterprise-class business intelligence appliance provider.
Top
TDWI’s BI Maturity Model shows the journey that most organizations undertake when implementing business intelligence and data warehousing (BI/DW). The model shows how the initiatives evolve from low-value, ancillary projects to high-value, strategic services that drive market share. Most organizations resonate with the narrative because it helps them identify where their BI/DW initiative is now, where it needs to go, and how to get there.
Benchmark Your BI Maturity with TDWI's New Assessment Tool |
|