Extending the Traditional Data Warehouse
How can we include new technologies with our existing DW environment? We present a new architecture that combines the stability and reliability of the older BI architectures while embracing the new and innovative technologies and techniques now available.
- By Claudia Imhoff
- March 15, 2016
Introduction
The real value of a decision-making environment is not in the creation of reports or simple multi-dimensional analytics. It is in the use of all forms of data for more sophisticated forms of analysis (diagnostic, predictive, and prescriptive analyses) and the production of superior analytic presentations through data visualization and storytelling capabilities.
The way to support these critical capabilities is by extending the traditional data warehouse environment to include more fluid, less controlled components in addition to the enterprise data warehouse. Everyone in the enterprise -- not just the specially trained data scientists or statisticians -- should be able to access all architectural components to include these valuable insights in their everyday decision making.
Supporting All Analytics: The Enterprise Analytics Architecture
Many technologists and implementers are confused about how and where these new capabilities and requirements will fit into their existing BI architectures, if at all. Does the enterprise data warehouse still have a role? Where do new technologies such as Hadoop, in-memory storage, columnar storage, and real-time analytic engines fit in? How can we satisfy the enterprise's need for real-time analytics on real-time data?
To answer these questions, a new architecture is needed -- one that combines the stability and reliability of the older BI architectures while embracing the new and innovative technologies and techniques now available. The extended data warehouse architecture, or XDW (see Figure 1), is just such an architecture.
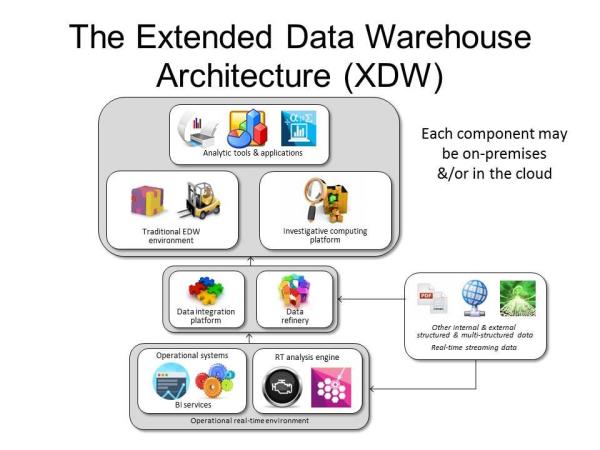
Figure 1: The extended data warehouse architecture.
Let's answer the first question: Does the traditional enterprise data warehouse (EDW) still have a role in the new architecture? The answer is a resounding yes -- at least for the foreseeable future of BI -- although its role has changed somewhat. It has become the source of established standard production reports, historical comparisons, and analytics. The data warehouse is still the best source of reliable, consistent, integrated quality data for critical or sensitive BI analyses for financial, compliance, or regulatory requirements. It is also the source for standard dashboard components such as KPIs and standard metrics such as profitability used by operations, marketing, sales, and other departments.
Nothing beats this workhorse and its associated data integration, quality, and profiling processes (data integration platform) for these important and trusted production BI deliverables. The XDW does acknowledge that the EDW has limitations as well, especially when dealing with unusual types of data, experimental or investigative analyses, and real-time analyses.
To answer the second question -- Where do Hadoop and the other innovative analytical technologies fit in? -- we must recognize innovations in both relational and non-relational data management systems, in analytic capabilities, and in data integration processes. These require that we move outside the traditional EDW to add new components to our analytics architecture.
There are three components that extend the EDW environment to support the next-generation BI as described. First is the investigative computing platform. This is where the new innovations in relational and NoSQL technologies such as Hadoop and Spark shine. This component is used for the exploration of big data sources and developing specialized analytics such as data mining, cause-and-effect analyses, "what if" explorations, and pattern analysis, as well as the difficult general, unplanned investigations of this data.
Some organizations may use the investigative computing platform as a simple sandbox for experimentation; others create a full analytic platform or use it as an extension of the data refinery (described below). This component gives companies the ability to freely and quickly analyze and experiment with large volumes of data with phenomenal performance. The output from these activities could be used by the EDW, a real-time analysis engine in the operational environment, or by a standalone line-of-business application.
The next component is a relatively new one for many implementers of analytics environments -- the data refinery. Its purpose is to ingest raw detailed data in batch and/or in real time from new and unusual sources of big data such as sensors, social media, and RFID tags and load it into a managed relational or non-relational data store. The data refinery -- just like its counterpart, an oil refinery -- distills the raw (big) data into useful and usable information and distributes it to other components (e.g., the investigative computing platform or EDW). The technologies supporting data preparation or data wrangling fit into this new area nicely.
The final extension to the data warehouse architecture answers the third question and consists of a real-time (RT) analysis platform found within the operational environment. Its purpose is to support the development and/or deployment of real-time analytical applications or streaming analytics for such applications as fraud detection, web event analysis, traffic flow optimization, and risk analysis. The models and rules embedded in the RT analysis platform are most likely developed in the EDW or investigative components or within the RT Analysis Platform itself, requiring tight integration and freely flowing data to and from these components.
Integration of Three Analytics Environments
Enterprises are rapidly implementing these environments to enjoy fuller, richer analytics about their critical entities. However, the three environments cannot be implemented in isolation from each other; the analytics must ultimately be brought together physically (or more likely virtually) and presented to the business community for decision-making. You will find that data virtualization becomes another critical data integration tool for the XDW.
As an example of the interconnected nature of the XDW components, Figure 2 shows the various analytics environments working together -- in this case virtually -- to present the results to a call center representative or a visitor to the enterprise's website. The EDW produces routine customer analytics such as lifetime value scores, segmentation, buying behaviors, and propensity to purchase. External data flows in with more customer data such as their current location and social media posts. These may flow directly into the investigative computing platform or through the refinery first before experimental analyses are performed. Historical analytical results are used to create the various analytical models and other advanced analyses.
Together the experimental results and the historical models or analytics are fed into the RT analysis engine, where they are combined with streaming analytics, ultimately creating a next-best product offer, intervention activities based on churn likelihood, or location-based offers to customers. To bring all these analytics results together, many companies use virtualization technologies for a more flexible, mix-and-match approach.
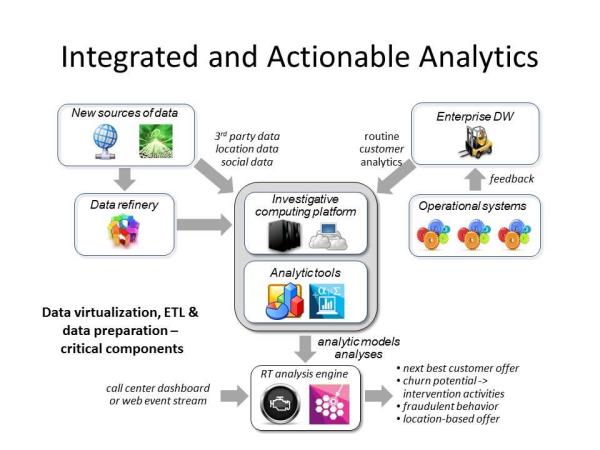
Figure 2: All XDW components must work together to create actionable analytics.
Summary
There can be no doubt that this period of innovation has had and will continue to have a dramatic effect on our analytical environments. Today's enterprises must look to the future with a critical but open mind while maintaining realistic and grounded knowledge about its existing technologies for analytics.
New forms of data management functions, analytical capabilities, and deployment methods mean that every enterprise, regardless of size or complexity, can reap great benefits from their analytical environments. The smart companies will study these carefully along with the business problems they face to determine the best way forward.
About the Author
A thought leader, visionary, and practitioner, Claudia Imhoff, Ph.D., is an internationally recognized expert on analytics, business intelligence, and the architectures to support these initiatives. Dr. Imhoff has coauthored five books on these subjects and writes articles (totaling more than 150 to date) for technical and business magazines.
She is also the founder of the Boulder BI Brain Trust (#BBBT), a consortium of 250 independent analysts and consultants with an interest in BI, analytics, and the infrastructures supporting them. The BBT was retired after 16 years in 2022.