Making Big Data Smarter
Transforming unstructured knowledge analytics practices into smart big data will increase your organization's innovation speed and relevancy. Here's how to jazz up your unstructured data analysis.
By Kate Pugh and Randy Bean
We sat with a colleague at a large development bank. He explained an ambitious program of synthesizing content from academic journals, the press, blogs, and government policy papers. We began to iterate different ways to approach the challenge, such as semantic crawling, taxonomy-based rules engine, and temporal term correlation. Though a bit rusty making a recommendation (as tools have progressed rapidly in the last year), we were confident on one thing: invest in the feedback, not just the data.
"Big data" is front of mind for a rapidly growing roster of global enterprises. Whether big data refers to exponentially larger volumes of data, the speed at which new data is being created, or new types of data, responding to the challenges and opportunities of the "data revolution" has become a corporate priority.
One of the most compelling opportunities of the big data boom is learning from unstructured data. What do we mean by unstructured data? Organizations are now actively analyzing their documents and content, using text mining and other analytical tools, to detect customer sentiment or to spot compelling trends as they develop. It is not just numbers (structured data) that are the focus of big data scientists now; it is customer activity, corporate actions, social media (e.g., Facebook and Twitter) patterns, and in-house intranet themes that organizations are mining for new insight and meaning. Unstructured knowledge is a core asset -- to woo customers, win feature wars, claim a new market segment, or innovate into new product areas.
Existing approaches to data using traditional business architecture and business intelligence methodologies tend to approach the problem as a project, answering a specific question such as "What branches could be more profitable if we extended hours?" However, it is the cumulative insight, and the progressive conversations from analysis to analysis, that differentiate the excellent organizations from those that lag.
Consider discovery and development -- in business or the natural or social sciences -- as a closed-loop process. Discovery, or "research," is about new and emergent insights and innovations. It entails revealing fundamentally new patterns in the data -- including unconventional combinations and correlations -- and informing the development team of new product designs, enabling tools or process structures. (Unstructured-data research is an area ripe for new innovations with big data tools.)
Development is commonly defined as a continuous experimentation with evolving models to understand patterns in the data. Development is often limited to awaiting changes in research results and "drilling down" to understand the changes. We contend that development should instead be a proactive process, tapping the natural and social world to combine the wisdom of different disciplines, societies, and markets. It should center on feedback and reflection: reassessing existing models in the light of experience, coupled with diverse lenses on the world.
A development paradigm features a continuous feedback loop between data, models, and dialogue. It isn't just a matter of more iteration. Rather, to reform your unstructured data development lens with insights means shifting budget from tools and data pull-backs to tacit knowledge integration.
Tacit knowledge is the hidden know-how that we have about how the world works, or about how different concepts, events, or theories connect. There are many ways to draw out tacit knowledge. Some focus on unpacking the wisdom of a "sage" or a team by conducting interviews. Some focus on unpacking an insight through performing concept mapping with an expert. Some send the experts to a wiki. However, few of these approaches are focused on the explicit integration of insights into practice. We have found that the conversation-based process does just this. It draws out the insights of several experts -- for example, a sociologist, a supply chain manager, or an apparel buyer -- in the presence of those who will be applying that knowledge in model development.
A well-facilitated, timely conversation can surface unexpected insights. Those who will apply the knowledge ("brokers") bring in their own context and ask pointed questions about putting ideas into practice. Those who have the knowledge ("originators") tap into patterns or connections that they themselves didn't even know they knew. Knowledge Jam, the subject of Sharing Hidden Know-How (Jossey-Bass/Wiley, 2011), is a process that does this: it engages a diverse group of originators and brokers in conversation to make practical sense of patterns -- and collectively imagine the future.
The trick is to get the right kind of diversity into the room (or virtual room). When diverse Knowledge Jam participants talk about trends in the data, they look beyond the usual suspects. You might hear: "What was happening in the contiguous market for precious metals?" "What does gender have to do with retweeting?" "What 1960s movie star wore dresses with an exposed metal zipper?"
Consider the graphic below. The Knowledge Jam is used at the outset of a discovery and development program. Experts from a number of fields are gathered with modelers to "jam" and create an initial lens. In the back and forth of that conversation -- for example, between social scientists and engineers -- the group decides to include new fields or time intervals. They tell each other why they'd choose data filters, weight variables, and revisit logic. An initial model is used. Then, with each development iteration, new patterns and visualizations are brought back into a Knowledge Jam. Participants then integrate newfound patterns across a variety of perspectives, and find a collective explanation of what they are seeing. Integrating multiple views, the model is refined in a more innovative way.
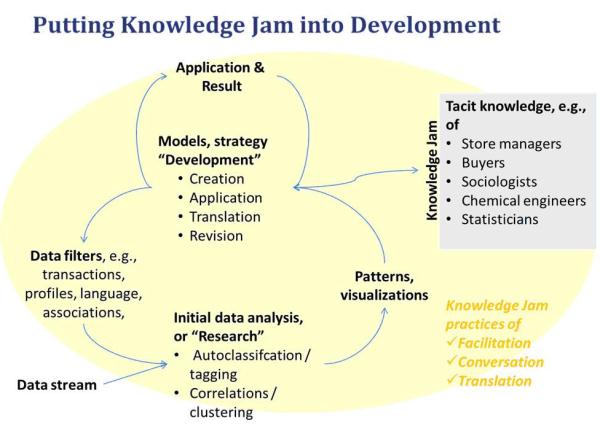
According to Scott Page, in The Difference (2007), when you bring together different heuristics and perspectives, you increase the problem-solving speed and predictive potential of a group. Yet, it doesn't stop there. The insights flow into productive applications, such as store hours or sewer siting. Then you hold more Knowledge Jams to reflect on real-world results. In this development cycle, diverse views are brought in a timely manner to help actors to interpret, re-model, and redirect the filter for the next iterations.
"We're not in the anthropology business," you might say. What does this take? How do you manage this? To put Knowledge Jam into large-scale innovation takes planning and facilitation. It means orchestrating well-structured meetings where these conversations can occur. It means keeping strong records of how predictions were met and how the different perspectives contributed to those predictions. It means being both organized and open to surprise.
As enterprises organize to address the opportunities and new avenues opened by big data, unstructured knowledge analytics has emerged as a core competency. The ability to transform unstructured knowledge analytics practices into "smart big data," employing innovative approaches such as the Knowledge Jam, will increase your organization's innovation speed and relevancy. The trick is to harness diversity as an asset and balance the surprise insights with the discipline of iteration. We believe knowledge-sharing processes open up exciting new avenues for businesses to leverage this largely unchartered and unharnessed asset – the rich trove of unstructured big data.
Kate Pugh is a consultant with NewVantage Partners, leading the practice in unstructured content. She is author of Sharing Hidden Know-How (Jossey-Bass/Wiley, 2011) and on the faculty of Columbia University's Information and Knowledge Strategy Masters Program. You can contact the author at [email protected].Randy Bean is managing partner and a founder of NewVantage Partners. He is a thought leader in guiding organizations in leveraging data as a strategic asset. NewVantage Partners provides executive thought-leadership and advises leading Fortune 1000, government, and global development agencies. You can contact the author at [email protected].