The Ramifications of Trusted Data
What is "trusted data" and how do we achieve it?
- By Philip Russom, Ph.D.
- November 4, 2010
The term trusted data has been bandied about a lot lately, and everyone seems to have a different definition. It’s an important concept, so in this column I will define the term and consider the ramifications for business intelligence and other data-driven business processes.
For some, trusted data is an emotional matter. In the context of business intelligence (BI), they want to feel confident that data presented in reports, cubes, dashboards, and other BI products is in the best condition possible because data in good condition makes their jobs easier and their actions more accurate, timely, effective, and compliant. People who consume data through operational applications have similar concerns.
Emotions aside, the condition of trusted data is easily quantified. Namely, condition is a technical measurement of data’s completeness, quality, age, schema, profile, and documentation. The assumption is that trusted data should come from carefully selected sources, be transformed in accordance with data’s intended use, and be delivered in formats and time frames that are appropriate to specific consumers of reports and other manifestations of data.
Hence, the trustworthiness of data is quantified mostly by the technical properties that define its condition. You still need to be mindful of the emotional impact that data’s condition has on the perceptions of people who consume the data for BI.
(Commentary continues below)
Related Resources
White Papers:
Six Steps to Managing Data Quality with SQL Server Integration Services
Strategies for Eliminating Performance Problems in Data Integration Environments
Webinars:
What’s Required for Enterprise Business Intelligence Deployments
Unifying the Practices of Data Profiling, Integration, and Quality (dPIQ)
(Commentary continues)
Why We Need Trusted Data
A lack of trusted data leads to a number of poor practices. For example, if BI data isn’t trustworthy because it’s not in good condition, then poor decisions are based on poor data. Whether data is in good condition or not, the mere perception that the data’s not trusted can lead users to ignore supplied BI data and instead build their own BI data stores, such as rogue data marts, spreadsheets, and personal productivity databases.
Less-than-trustworthy data creates problems in operational processes, too. When data is (or is perceived to be) of poor quality or incomplete, users and managers base tactical and operational decisions on guesses.
If you can turn these problems around, then trusted data has benefits. Whether in BI or operations, people will use data they trust, which, in turn, leads to greater consistency, compliance, and accuracy in business processes based on the data.
How to Get Trusted Data
Achieving trustworthiness for data is a multi-step process:
- Select sources that are appropriate, certified, and diverse
- Process data to transform and aggregate it for the intended use, improve its quality, and enhance its meta and master data
- Collaborate cross-functionally and govern data for compliance
- Deliver data in the right time frame, in forms suited to its intended use
The process and its best practices involve a mix of:
- Organizational effort: Business and technical people and teams, in collaboration
- Technical automation: Information management tools and techniques for integration, quality, databases, applications, metadata and master data management, and so on
A Final Word
Giving business and technical users data they trust is key to good business intelligence. If users don’t have confidence in the data of a data warehouse and other BI data stores, they may argue over the data’s accuracy, refuse to use the reports and analyses fed from the data, or build their own data stores. Non-trusted data likewise hinders operational excellence, as people side-step prescribed processes and misuse applications. All these paths are non-productive and lead to faulty decision making and operational actions.
Stay tuned. In an upcoming Experts in BI newsletter, I’ll drill down into the Six Cs of Trusted Data, namely how data integration and related techniques build trust by making data complete, current, consistent, clean, compliant, and collaborative.
To learn more, replay Philip Russom’s recent TDWI Webinar on Trusted Data for BI online.
Philip Russom is senior manager of TDWI Research. Philip can be reached at [email protected] .
Related Resources
White Papers:
Webinars:

What’s Required for Enterprise Business Intelligence Deployments
December 7, 2010
Speaker: Mark Madsen
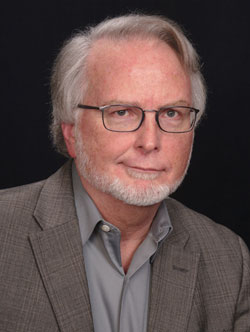
Unifying the Practices of Data Profiling, Integration, and Quality (dPIQ)
December 8, 2010
Speaker: Philip Russom