Introduction to Unified Data Management
By Philip Russom
BACKGROUND
In most organizations today, data and other information are managed in isolated silos by independent teams using various data management tools for data quality, data integration, data governance and stewardship, metadata and master data management, B2B data exchange, content management, database administration and architecture, information lifecycle management, and so on. In response to this situation, some organizations are adopting what TDWI calls unified data management (UDM), a practice that holistically coordinates teams and integrates tools. Other common names for this practice include enterprise data management and enterprise information management. Regardless of what you call it, the “big picture” that results from bringing diverse data disciplines together yields several benefits, such as cross-system data standards, cross-tool architectures, cross-team design and development synergies, leveraging data as an organizational asset, and assuring data’s integrity and lineage as it travels across multiple organizations and technology platforms.
But unified data management isn’t purely an exercise in technology. Once it paves the way to managing data as an organizational asset, the ultimate goal of UDM becomes to achieve strategic, data-driven business objectives, such as fully informed operational excellence and business intelligence, plus related goals in governance, compliance, business transformation, and business integration. In fact, the challenge of UDM is to balance its two important goals—uniting multiple data management practices and aligning these with business goals that depend on data for success.
The purpose of this report is to help organizations plan and execute effective UDM efforts. Many need the help, because UDM is a relatively new shift in best practices for data management. Toward that end, the report drills into the business initiatives that need UDM, the data management practices and tools that support it, and the organizational structures that enable the cross-functional collaboration that’s critical to UDM success.
Definitions of UDM
With all the above in mind, here’s a nutshell definition of UDM:
TDWI Research defines unified data management as a best practice for coordinating diverse data management disciplines, so that data is managed according to enterprisewide goals that promote technical efficiencies and support strategic, data-oriented business goals.
The term UDM itself seems focused on data management, which suggests that it’s purely a technical affair. But this is misleading, because UDM—when performed to its full potential—is actually a unification of both technology practices and business management. For UDM to be considered successful, it should satisfy and balance both of the following requirements:
- UDM must coordinate diverse data management disciplines. This is mostly about coordinating the development efforts of data management teams and enabling greater interoperability among their servers. UDM may also involve the sharing or unifying of technical infrastructure and data architecture components that are relevant to data management. There are different ways to describe the resulting practice, and users who have achieved UDM call it a holistic, coordinated, collaborative, integrated, or unified practice. Regardless of the adjective you prefer, the point is that UDM practices must be inherently holistic if you are to improve and leverage data on a broad enterprise scale.
- UDM must support strategic business objectives. For this to happen, business managers must first know their business goals, then communicate data-oriented requirements to data management professionals and their management. Ideally, the corporate business plan should include requirements and milestones for data management. Hence, although UDM is initially about coordinating data management functions, it should eventually lead to better alignment between data management work and information-driven business goals of the enterprise. When UDM supports strategic business goals, UDM itself
becomes strategic.
Let’s expand TDWI’s terse definition of UDM by drilling
into more specific details and issues.
UDM is largely about best practices from a technical user’s
viewpoint. Most UDM work involves collaboration among
data management professionals of varying specialties
(such as data integration, quality, master data, etc.). The
collaboration fosters cross-solution data and development
standards, interoperability of multiple data management
solutions, and a grander concept of data and data
management architectures.
UDM isn’t a single type of vendor-supplied tool. Even so, a
few leading software vendors (including all the vendor
companies sponsoring this report) are shaping their
offerings into UDM platforms. Such a platform consists of
a portfolio of multiple tools for multiple data management
disciplines, the most common being BI, data quality, data
integration, master data management, and data governance.
For the platform to be truly unified, all tools in the
portfolio should share a common graphical user interface
(GUI) for development and administration, servers should
interoperate in deployment, and all tools should share key
development artifacts (such as metadata, master data, data
profiles, data models, etc.). Having all these conditions is
ideal, but not an absolute requirement. As one interviewee
put it: “The tools’ servers have to interoperate or—at
the end of the day—the solution isn’t unified. So that’s
a ‘must have,’ as is shared metadata. If there are multiple
development GUIs, I can live with that.”
UDM often starts with pairs of practices. UDM is a
matter of degree. In other words, it’s unlikely that any
organization would want or need to coordinate 100%
of its data management work via UDM or anything
similar. Instead, organizations opportunistically select
combinations of data management practices whose
coordination and collaboration will yield appreciable
benefits. The most common combinations are pairs, as
with data integration and data quality or data governance
and master data management. Over time, an organization
may extend the reach of UDM by coalescing these pairs
and adding in secondary, supporting data management
disciplines, such as metadata management, data modeling,
and data profiling. Hence, the scope of UDM tends to
broaden over time into a more comprehensive enterprise
practice. And the scope can get rather broad, as a user
interviewed for this report explained: “Enterprise-scale
data management is like most things: it’s a mix of people,
process, and technology. The range of each is diverse, so
there’s potentially a place for just about anything.”
A variety of organizational structures can support UDM. It
can be a standalone program or a subset of larger programs
for IT centralization and consolidation, IT-to-business
alignment, data as an enterprise asset, and various types of
business integrations and business transformations. UDM
can be overseen by a competency center, a data governance
committee, a data stewardship program, or some other
data-oriented organizational structure. UDM is often
executed purely within the scope of a program for BI and
data warehousing (DW), but it may also reach into some
or all operational data management disciplines (such as
database administration, operational data integration, and
enterprise data architecture).
UDM unifies many things. As its name suggests, it unifies
disparate data disciplines and their technical solutions.
On an organizational level, it also unifies the teams that
design and deploy such solutions. The unification may
simply involve greater collaboration among technical teams,
or it may involve the consolidation of teams, perhaps
into a data management competency center. In terms of
deployed solutions, unification means a certain amount of
interoperability among servers, and possibly integration of
developer tool GUIs. Technology aside, UDM also forces
a certain amount of unification among business people,
as they come together to better define strategic business
goals and their data requirements. When all goes well, a
mature UDM effort unifies both technical and business
teams through IT-to-business alignment germane to data
management.

Related Terms and Concepts
Most likely, you’ve never heard the term “unified data
management.” After all, most organizations coordinating
diverse data management disciplines do so without giving
their actions a name. For example, the survey for this
report asked: “What do you call the coordination of data
management disciplines in your organization?” Two-thirds
(66%) of survey respondents answered: “We don’t have a
formal name for it.” (See Figure 1.) Likewise, in the user
interviews conducted for this report, users and consultants
alike described how they coordinate data management
work and align it with stated business goals for data—but few had a name for it. Even stranger, most software
vendors that offer a portfolio of multiple data management
tools have no term for the coordinated use of the portfolio!
However, a third of survey respondents (34% in Figure 1)
have given coordinated data management a name, and they
typed that name into the online survey. The names they
report using reveal much about how users think about such
coordination. (See Figure 2.)
Generic terms for UDM. A lot of users keep it simple
by referring to their coordinated efforts as simply data
management (16%) or information management (11%).
In fact, most users interviewed for this report stated that
they just assume that good data management involves
technical people of diverse disciplines learning from each
other, complying with data and development standards,
considering cross-discipline architectures, and the other
best practices this report associates with UDM.
Generic terms, but with enterprise aspirations. If you put
the word “enterprise” in front of common terms like
data management and information management (thus
denoting a broad enterprise scope), then you get two of
the most popular terms entered into this report’s survey:
enterprise data management (EDM, 15%)1 and enterprise
information management (EIM, 11%)2. By coincidence,
these acronyms are strongly associated with vendors that
have promoted them, namely EIM with SAP and Business
Objects and EDM with DataFlux and SAS.
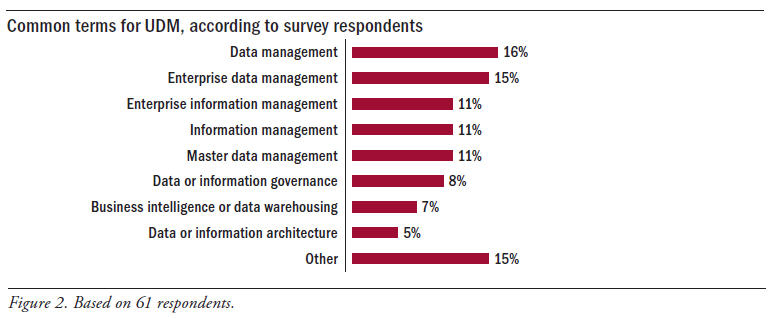
UDM as a subset of other programs. A number of survey
respondents called their UDM-like activities by the names
of other data management practices, in particular master
data management (MDM, 11%), data or information
governance (8%), BI or data warehousing (7%), and
data or information architecture (5%). Each is a rather
broad program, and each typically involves multiple
data management practices. So it’s possible that users
responding to the survey were thinking of UDM as a
subset that helps unify the data management solutions
created and maintained by these programs.
Why Care about UDM Now?
There are many reasons why organizations need to step up
their efforts with UDM:
Technology drivers. From a technology viewpoint, the lack
of coordination among data management disciplines leads
to redundant staffing and limited developer productivity.
Even worse, competing data management solutions can
inhibit data’s quality, integrity, consistency, standards,
scalability, architecture, and so on. On the upside, UDM
fosters greater developer productivity, cross-system data
standards, cross-tool architectures, cross-team design and
development synergies, and assuring data’s integrity and
lineage as it travels across multiple organizations and
technology platforms.
Business drivers. From a business viewpoint, data-driven
business initiatives (including BI, CRM, regulatory
compliance, and business operations) suffer due to low
data quality and incomplete information, inconsistent
data definitions, noncompliant data, and uncontrolled
data usage. UDM helps avoid these problems, plus it
enables “big picture” data-driven business methods such
as data governance, data security and privacy, operational
excellence, better decision making, and leveraging data as
an organizational asset.
1 See the 2009 TDWI Monograph Enterprise Information Management: In Support
of Operational, Analytic, and Governance Initiatives, online at tdwi.org/research/
monographs.
2 See the 2009 TDWI Checklist Report Enterprise Data Management, online at
tdwi.org/research/checklists.
Philip Russom is the senior manager of Research at TDWI, where he oversees many of TDWI’s research-oriented
publications, services, and events. He can be reached at
[email protected].
This article was excerpted from the full, 27-page report, Unified
Data Management: A Collaboration of Data Disciplines and Business
Strategies. You can download this and other TDWI Research free of
charge at tdwi.org/research/reportseries.
The report was sponsored by ASG, DataFlux, Informatica, SAP, Talend,
Teradata, and Trillium Software.