August 6, 2009: TDWI FlashPoint - Metadata, the Secret Data Asset
In this issue of TDWI FlashPoint, Patty Haines explains why business metadata is a valuable asset, Philip Russom shares the requirements for business-to-business (B2B) data exchange, and Jill Dyché details how data governance is different at every company.
Welcome to TDWI FlashPoint. In this issue, Patty Haines explains why business metadata is a valuable asset, Philip Russom shares the requirements for business-to-business (B2B) data exchange, and Jill Dyché details how data governance is different at every company.
Metadata: The Secret Data Asset
Patty Haines, Chimney Rock Information Solutions
As your data warehouse grows, it should become the most important information asset within your organization. Ideally, it should do more than just provide the most accurate data. It should become the source for the most accurate and complete documentation about your organization and your data.
Some operational system projects are implemented without complete documentation or business-focused documentation about your data. After a few years of enhancements to operational systems, documentation becomes inaccurate or outdated.
This is where the data warehouse comes in. During the life of your data warehouse, all data elements from source tables and files are analyzed. Secrets unfold as the development team works on the data warehouse. When combined, these tidbits of information provide a wealth of information for business users. This may be the first time some business users understand a standard process or a method used for a critical task or process within your organization.
Data warehouses are designed to provide easy-to-use, accurate, and consistent data. To do this, they require complete and clear business-related documentation for each piece of data users can access. Here are the steps necessary for building this documentation:
Collect. Collect metadata in any manner possible and from any available source: from source system documentation, user guides, Web pages, legal guides, source code, validation tables, database constraints, and people, including the business community and IS support staff.
Document. To efficiently collect metadata, you must first define a standard format to document what has been found so it can easily be used by the data warehouse team. There should be a structure to this documentation, as well as procedures for documenting definitions, data examples, domain values, issues, and data complexities. You must identify a responsible person and update dates with a control mechanism so documentation is not lost or compromised.
Validate. You can validate the information you collect in several ways. For example, you can identify the domain of values by analyzing and comparing the validation tables, database constraints, and the actual values found in the data. The final review should be completed by different groups within the business community and the IS support groups who are experts in the subject to ensure all data is accurate and documented in complete business terms.
Develop business metadata. After you validate your information, you must determine how this metadata will be shared and presented to the business community and data warehouse users. Most query and reporting tools can use business names for data; they also provide a method to display descriptions for data elements. A standard format for these business names and descriptions, including a definition, possible values, and format should be developed. Many tools also provide functions to export names and descriptions into a printable format that can be distributed or stored in a shared environment. Have business users review this documentation for accuracy and readability.
Share the metadata. Another method for presenting metadata to the business community is to conduct training sessions about using the query and reporting tools and about reviewing the business metadata with business users. Users' success with your data warehouse depends on their understanding of what data is available, what it means, and how it is being provided.
Periodic user workshops also work well, providing a forum for users to present their experiences with the data warehouse, request enhancements, and make updates to the metadata. These workshops can be as short as “Lunch and Learn” sessions open to the entire organization. Newsletters can provide a refresher on the data and metadata.
Conclusion
It won’t be long until your business community uses business-focused documentation for nontraditional data warehouse uses such as internal staff training or sharing processes and methods across your organization. Business metadata can be a challenge to build but will soon be recognized as one of your organization's most valuable assets.
Patty Haines is president of Chimney Rock Information Solutions, a company specializing in data warehousing and master data management. She can be reached at 303.697.7740.
Top
No Free Lunch: The Problem with Data Governance
Jill Dyché, Baseline Consulting
I was recently asked by a BI client to attend a meeting of its newly formed data governance council. The members of the council were new to data governance and to participation in an enterprise-scale committee. The meeting included discussions of enterprise data modeling, the need for metadata, who could fund a data steward, and what members knew about semantic technologies. It was as if the council’s members were ordering off a menu they would not be eating from.
No matter how much the economy ebbs and flows--and here’s to the flow--data is still moving around, inside and outside of corporations, at a faster clip than ever. Data governance--the policy making and decision rights around enterprise information--has been getting hotter since the term “infoglut” entered the executive lexicon and companies started blaming the failure of their business initiatives on the lack of consistent and accurate data.
A few years ago, when data governance was just warming up, people from both the business and IT would call me and explain why their companies needed to manage their data and how we might be able to help them. I’d talk to them for a bit, realize they were right, and then ask what they thought was the best approach for getting started. “If we could just get everyone in a room…” they’d say.
Flash forward a few years and we’ve realized that the “get everyone in a room” scenario can be fraught with problems. We’ve watched as business people have pushed back--“Data governance? Don’t we need, like, corporate governance first?”--and IT people struggled to engage them. Likewise, we heard IT people dismiss data governance as a business responsibility: “We can’t tell them their policies for their data--they have to tell us.”
When it did work, it tended to fizzle. I’ve talked before about what I call the “kickoff and cold cuts” approach to data governance. Someone calls a lunchtime meeting, complete with sandwiches from the local deli, inviting data stakeholders to hold forth on “data is a corporate asset.” Between the potato salad and the oatmeal cookie there’s a heated discussion about “why we need to fix our data,” with agreement all around. When a follow-up meeting is scheduled, everyone signs up. However, there’s no free lunch in the next meeting. Fewer people show up, and no one knows quite what to do about the data problem. Someone anoints the group a “council,” but by the third meeting the council is already losing steam, and it degenerates into a series of complaint sessions and ownership debates.
The truth is, data governance must be deliberately designed before it’s launched. It’s fine to get everyone in a room as long as the right people are selected and they discuss relevant issues and are authorized to make decisions about them. Far too often, though, people don’t approach data governance the right way, which varies depending on a company’s culture, information usage, incumbent skill sets, and existing organizational structures. The truth is there’s no template for data governance. It’s different at every company.
Having said that, there are two main approaches to data governance: top down and bottom up. Knowing which approach best suits your company can take you a long way in launching a data governance program that’s adopted and sustainable. It will help manage expectations with constituents at the outset, thus ensuring long-term success.
Consider two of our clients. One, a multinational bank, is hierarchical and formal. Decision making is top down. Relatively meager expenses travel high in the organization for sign-off. Executives are big on “town hall” meetings and roundtable discussions. Consensus reigns. For the bank, we designed a top-down data governance process that included establishing a vision, creating a set of guiding principles that would become the touchstones for individual decisions, deliberate assignment of decision rights across stakeholder groups, and a rigorous and repeatable execution process.
The other client is a high-tech firm where even business execs are tech savvy. People come to work at 10 p.m. with a six-pack, the dog, and some really good ideas, and start coding until 10 the next morning, when they’ll toss the empty beer cans in the recycling bin, load the dog in the back of the Subaru, and head over to the trailhead. These employees fix their own problems and make their own decisions. Anarchy is the rule. With them, we embarked on a data governance proof of concept that looked like this:
(Click to enlarge)
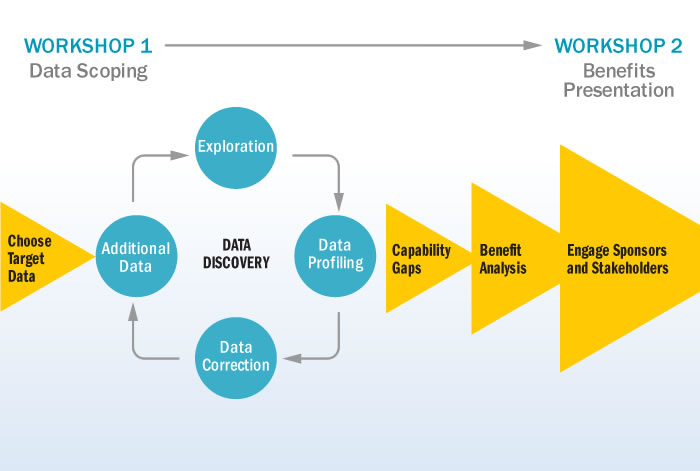
Needless to say, data governance works completely differently at the bank and the high-tech firm. At the bank, three different governing bodies are involved in the data governance process, each with its own checks and balances. The high-tech company relies on a more grassroots--yet formal--effort. The stewards use an online knowledge base to submit decisions for review, subject to occasional tie breaking by executives. These stewardship units are endorsed by divisional managers who want to see measurements for data quality, integration, and deployment velocity improve.
How do you know whether a top-down or a bottom-up approach is right for your company? Here are some good indicators:
Top-Down Data Governance Indicators
- There is a large or pending corporate initiative whose success will be contingent on information usage across organizations
- Managers and staff across lines of business consistently complain about the same data issues
- An executive or decision maker with authority has begun using the term "data as an asset"
- There is consensus across organizations about the need to assign data ownership
- Tie breaking among organizations is needed for data definitions, business rules, data usage/policies, and security and privacy rules
When considering a top-down approach, you'd describe your company as hierarchical, policy driven, and centralized.
Bottom-Up Data Governance Indicators
- A specific project is being hindered by the lack of data skills
- A team on the business side needs help for a specific initiative and doesn’t know where to begin
- There is a key project that could benefit from tactical data improvements, such as metadata services or address cleansing
- Available budget is a hindrance to moving forward
- The poor quality of data is widely acknowledged for a particular source system, business application, data subject area (e.g., product), or business process (e.g., target marketing)
Data governance design involves more than just deciding whether you’ll take the top-down or the bottom-up approach, but it’s a critical discussion. It’s the bazooka-or-sniper-rifle debate, and it has to be had. Get ready, since it could be one of many.
Jill Dyché is a partner with Baseline Consulting, an acknowledged leader in information design and deployment. Jill and Kimberly Nevala, a senior consultant at Baseline, delivered their new workshop, Data Governance for BI Professionals, on Monday, August 3, at the TDWI World Conference in San Diego. You can read Jill’s blog at www.jilldyche.com.
References
Dyché, Jill [2007]. “A Data Governance Manifesto: Designing and Deploying Sustainable Data Governance,” white paper.
Dyché, Jill, and Kimberly Nevala [2008]. “Ten Mistakes to Avoid When Launching a Data Governance Program,” Ten Mistakes to Avoid series, TDWI.
Foley, John [1995]. “Managing Information: Infoglut,” InformationWeek.
Top
Business-to-Business (B2B) Data Exchange
Philip Russom, TDWI Research
A growing area within operational data integration is inter-organizational data integration. This usually takes the form of documents or files containing data that are exchanged between two or more organizations. Depending on how the organizations are related, data exchange may occur between business units of the same company or between companies that are partners in a business-to-business (B2B) relationship. Either way, this OpDI practice is called B2B data exchange.
Data Standards are Critical Success Factors for B2B Data Exchange
One of the interesting architectural features of B2B data exchange is that it links together organizations that are incapable of communicating directly. That’s because data is flowing from the operational and transactional IT systems of one organization to those of another, and the systems of the two are very different in terms of their inherent interfaces and data models. Hence, data exchange almost always requires a third, neutral data model in the middle of the architecture that exists purely for the sake of B2B communication and collaboration via data.
(Click to enlarge)
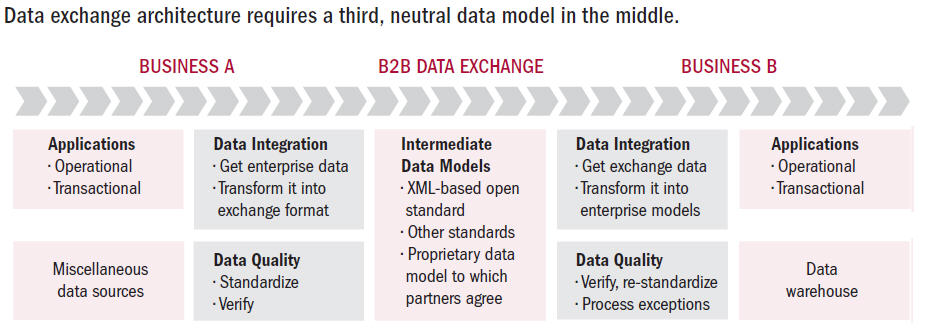
As an analogy, consider the French language. In the seventeenth, eighteenth, and nineteenth centuries, French was the language of diplomacy—literally a lingua franca—through which people from many nations communicated, regardless of their primary languages.
Data exchange very often transports operational data tagged with extensible markup language (XML). Although the X in XML stands for extensible, it might as well stand for exchange, because the majority of uses of XML involve B2B data exchange. In many ways, B2B data exchange is XML’s killer app, and XML has become a common B2B lingua franca.
For the data model in the middle of a data exchange architecture to be effective as a lingua franca, all parties must be able to read and write it. This is why open standards are so important to data exchange, including industry-specific standards like ACORD for insurance, HIPAA and HL7 for healthcare, MISMO for mortgages, Rosettanet for manufacturing, SWIFT and NACHA for financial services, and EDI for procurement across industries. From this list, you can see that data exchange is inherently linked to standards that model data with a fair amount of complexity, in the sense of semi-structured data (as with all XML) and hierarchically structured data (as in ACORD and MISMO). Even so, some organizations forgo XML-based open standards in favor of unique, proprietary data models that all parties agree to comply with.
Note, however, that intermediary standards specify the format or model for data exchange, but they rarely specify all content combinations. This is especially the case with product data, because product attributes have so many possible (and equivalent) values, like blue, turquoise, cyan, aquamarine, and so on. Therefore, it behooves the receiving business to unilaterally enforce its own data content standards through a kind of "re-standardization" process.
Special Requirements for B2B Data Exchange
A few situations demanding special requirements for B2B data exchange deserve note:
Some B2B OpDI scenarios require special data stewardship functions. Relevant to B2B data exchange, a data steward may need to handle exceptions that a tool cannot process automatically. For instance, a data steward may need to approve and direct individual transactions or review and correct mappings between ambiguous product descriptions. To enable this functionality in an OpDI solution, it probably needs to include a data integration or data quality tool that supports special interactive functions for data governance and remediation, designed for use by data stewards.
Centralizing B2B data exchange via a hub reduces complexity and cost. Each industry has a variety of document formats and industry standards required for data exchange. Companies must comply with the latest formats and standards in order to maintain their competitive edge, to avoid regulatory penalties, and to prevent a loss of data. Staying current with standards forces users with point-to-point interfaces to invest in constant coding and maintenance. A centralized, hub-based B2B data exchange solution--built atop a vendor tool that is updated as standards change--reduces architectural complexity and maintenance costs.
B2B data exchange sometimes handles complex data models. All B2B data exchange solutions handle flat files, where every line of the file is in the same simple record format. But some solutions must also handle hierarchical data models, which are more complex and less predictable. Hierarchical models are typical of XML and EDI documents.
Product data may be unstructured. In product-oriented industries, B2B data exchange regularly handles product data, which often entails textual descriptions of products and product attributes. Processing textual data may require a tool that supports natural language processing or semantic processing, in addition to the specialized data stewardship described earlier.
User Story: B2B data exchange standards vary by industry
"Early in my career, I worked in financial services, where de jure data standards--especially SWIFT--are prominently used for B2B data exchange. But now I've been in manufacturing for several years, where data standards are mostly de facto, typically 'made up' by one or more business partners. Either way, supporting data exchange ‘standards’ and ad hoc formats is an important part of operational data integration work."
Philip Russom is the senior manager of research and services at TDWI, where he oversees many of TDWI’s research-oriented publications, services, and events. Prior to joining TDWI in 2005, Russom was an industry analyst covering BI at Forrester Research, Giga Information Group, and Hurwitz Group, as well as a contributing editor with Intelligent Enterprise and DM Review magazines.
This article is an excerpt from the TDWI Best Practices Report Operational Data Integration: A New Frontier for Data Management, online at www.tdwi.org/research/reportseries. B2B data exchange will be the focus of a TDWI Webinar on August 12, 2009. Register at www.tdwi.org/webinars.
Top