October 6, 2005 - TDWI FlashPoint: Baking BI into Operational Systems
Amit Jain and Tom Victory discuss operational systems.
Welcome to TDWI FlashPoint. In this issue, Amit Jain and Tom Victory discuss operational systems.
CONTENTS
FlashPoint Snapshot
FlashPoint Snapshots highlight key findings from TDWI's wide variety of research.
Which types of users do you plan to enable with insights from ERP/CRM BI projects?
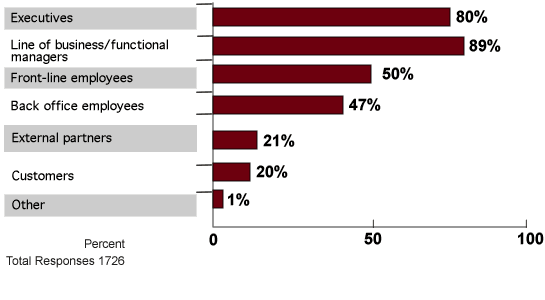
Which best describes your position?
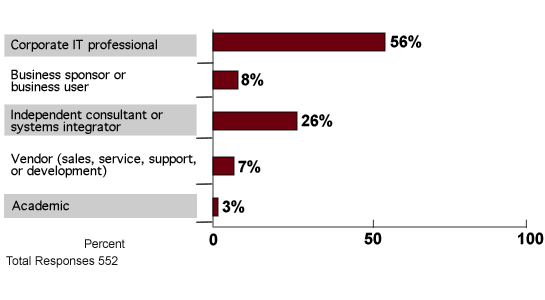
Based on 552 respondents.
Rounding and multi-choice questions are responsible for percent totals that do not equal exactly 100 percent.
Source: Developing a BI Strategy for CRM/ERP Data survey data, October 2004. Click here to access the report based on this survey data.
Top
Baking BI into Operational Systems
By Amit Jain and Tom Victory
DecisionPath Consulting
I Wish the Source Had One More Field...
Midway through analysis in many BI projects, designers find themselves wishing that the source operational systems had captured just a few more pieces of data: “If only we had the employee’s job history instead of just the current position,” or “If only we could link returns to the original order. Then we could... ”
This unmet need for more decision-oriented data from operational systems results from a structural problem that afflicts most IT organizations. The problem is that for most operational systems, the data warehouse extract requirements are the only requirements that reflect the needs of BI.
In most IT departments, the big operational systems dominate budgets, resources, and executive attention. When billing, fulfillment, or payroll systems go down, the business impact is immediate and dramatic. Yet most of the executive-level decisions that drive sustainable competitive advantage come from BI information, not from operational outputs. The challenge BI projects have is to capture resources and attention commensurate with the value of the decisions that depend on BI.
The solution is for the managers of BI projects to recast how they think about requirements. Instead of treating the information available from source systems as given, BI teams should be prepared to work with the source systems to capture more and better data. Adding BI-specific data to source systems can open entirely new subject areas to analysis and create opportunities for better decision making.
Case Study
Consider the (hypothetical) replacement of an antiquated point-of-sale system at a rapidly growing big-box electronics retailer. The goal of the new system is to reduce the average checkout time by 10 seconds per customer in the hope of improving customer service.
The requirements definition phase begins with the checkout clerks, as the system designers test usability, reliability, and transaction speed. The designers then speak with the secondary users, such as the auditors who review the register tapes, the accountants who track sales, and the supervisors who manage the checkouts.
However, there is a large community of users outside of the physical store who depend on sales transactions data. The finance organization depends on the data for budgeting and planning. The CFO wants up-to-the-minute sales data when he speaks to Wall Street analysts. Supply-chain planners use transaction data for planning and managing stock levels. Marketers use sales data for identifying hot products and improving the product mix. These users are typically not consulted during the implementation of a new cash register system, and hence their data needs are often not considered.
Beyond just using the point-of-sale information to complete the sale itself, capturing a few extra data items in the cash register system could dramatically increase the information impact on downstream decisions. Here are a few examples:
New Store Locations
New Data Captured: Customer home zip code
Decision Impact: For a growing retailer, strategic planners need sales data to determine where to open new locations. Sales data by itself is useful, but its value increases dramatically if the sale can be matched to a customer location. Asking each customer for a home zip code, while not necessary for completing the sale, opens a universe of new demographic analysis possibilities for store planners.
Checkout Productivity
New Data Captured: Checkout transaction duration
Decision Impact: With detailed information about checkout productivity, store managers can make better labor decisions about training and the mix between full-time and part-time employees. It also becomes possible to measure the cost impact of paperwork-intensive sales such as extended warranties or cell phone service contracts.
Intraday Product Mix
New Data Captured: Sale time of day
Decision Impact: The point-of-sale system might be designed to report total sales by item by day in each store. This level of detail is more than sufficient for the accountants, and even for the inventory planners. Adding time of day, however, would be valuable to marketers, who could differentiate between the needs of the lunch crowd (interested in a quick look at CDs) and evening shoppers who make large appliance purchases.
Why Is It Hard to Change Operational Systems?
There are three main factors that make it hard for BI teams to change the information collected by operational systems:
1. Operational and BI systems have different measures of success.
Operational systems serve larger numbers of users, at more locations, with very high uptime and performance requirements, while BI systems emphasize flexibility to support changing analytic needs. The focus on uptime and the logistical complexity of operational systems drives higher implementation costs and longer design/development cycles, which in turn make system owners reluctant and slow to add BI features.
2. The departments that pay for the systems get served first.
A key problem that BI projects have in effecting changes in operational systems is that such systems often serve different departments funded from different budgets. In the cash register example, the operational system’s implementation would be funded from the operations budget. Managers working on the project respond first and foremost to their sponsors; the rest of the organization gets their “best effort” with whatever resources are left. If the BI consumers in marketing and finance organizations want more influence over operational systems, they need to provide both sponsorship and budget support.
3. The value of BI is hard to quantify, especially in advance.
Good decisions can make the difference between success and bankruptcy, yet it is difficult to place a specific dollar value on them. For example, an electronics retailer knows that choosing the right locations for new stores is a vital decision, but it is still a challenge to measure exactly how better decision-making influences store profitability. Further, BI’s impact is often seen only in the long term. If the organizational horizon is limited to the current quarter, then it becomes difficult to make a case for BI and gain the resources it needs.
Actions to Increase the BI Impact of Operational Systems
Here are several things that IT management and the BI teams can do as part of a program to increase the organization’s BI maturity:
IT Management
- Explicitly forecast the BI value of changes to operational systems during IT planning and budgeting.
- Prioritize BI opportunities in the context of an overall BI program.
- Include downstream consumers of operational system data in project-steering committees and develop a comprehensive view of BI opportunities.
- Standardize common business terms and data structures across the organization.
BI Teams
- Bridge the cultural divide with the more conservative operational systems by focusing on a few key enhancements, rather than on a laundry list of requests.
- Place a BI team member on operational systems projects to scout new opportunities.
- Develop informal relationships between the BI teams and source system teams to enhance communications. Have an off-site meeting at a coffee shop or ice cream parlor.
- Keep a desired enhancements log, including ideas that are currently technically infeasible.
Operational systems are the key way an organization sees the outside world. They should be designed for capturing all of the information that the organization needs. Modest additions to operational systems can have a big BI impact. Rather than just wait for information to become available from operational systems, BI teams should actively influence operational systems to improve the decision impact of the captured information.
Amit Jain and Tom Victory are senior consultants at DecisionPath Consulting (www.DecisionPath.com).
Top
FlashPoint Rx
FlashPoint Rx prescribes a "Mistake to Avoid" for business intelligence and data warehousing professionals from TDWI's Ten Mistakes to Avoid series.
Ten Mistakes to Avoid for Mature Data Warehouses
Mistake 10: An Inflexible Data Loading Architecture that Inhibits Real-Time Loading
By William McKnight
Real-time data warehousing is loosely defined as warehouses that have no more than five minutes’ delay from an event’s operational occurrence to its appearance in the data warehouse. In the spirit of "more, better, faster," data warehouses that have historically loaded daily have found the need to load data intraday and so on until the last delay hurdle to be crossed is crossed—and that is real time.
Furthermore, the data warehouse, as the only place for clean, historical, accessible, enterprise-cleared data, becomes the de facto place to go for all data needs in mature data warehouse environments. This has opened them up to many so-called operational uses that require real-time data to be effective. In mature environments, data warehouses are not just for batch decision support anymore.
Real-time data warehouses have overcome two main issues. The first is the cooperation and lack of intrusion into the applications being sourced. Many legacy applications are fragile, expensive, and unruly to update. Many are non-relational and ill prepared to support extracts of any kind except during nightly batch windows. These limitations also drive many "operational" functions into the warehouse environment. Ultimately, these limitations can cause operational product replacement.
The second issue is the impact on data warehouse usage itself. If real-time sourcing and loading is a requirement, data access would be active as well. Usage of the warehouse cannot be disrupted by frequent loading activity. Real-time data warehouses require partitioning strategies that will minimally impact warehouse usage for the minimalist "load" that occurs frequently with a real-time warehouse (versus larger daily batch loads).
Overcoming these issues, whether with EAI (enterprise application integration) strategies that clear transactions to the warehouse in real time or the more frequent exercise of "batch" ETL approaches in the architecture, provides a rich reward to the mature data warehouse program—a real-time data warehouse.
This excerpt was pulled from the Q2, 2003, TDWI Ten Mistakes to Avoid series booklet, Ten Mistakes to Avoid for Mature Data Warehouses by William McKnight.
TDWI Members can review this entire publication online. If you would like to own a copy of this publication, contact Margaret Ikeda or dial: 206.246.5059, ext. 113.
Top