Predictive Analytics
- By Wayne Eckerson
- May 10, 2007
Extending the Value of Your Data Warehousing Investment
By Wayne W. Eckerson
What is Predictive Analytics?
Predictive analytics can help companies optimize existing processes, better understand customer behavior, identify unexpected opportunities, and anticipate problems before they happen. Almost all of TDWI’s Leadership Award winners in the past six years have applied predictive analytics in some form or another to achieve breakthrough business results.
High Value, Low Penetration. With such stellar credentials, the perplexing thing about predictive analytics is why so many organizations have yet to employ it. According to our research, only 21% of organizations have “fully” or “partially” implemented predictive analytics, while 19% have a project “under development” and a whopping 61% are still “exploring” the issue or have “no plans.” (See Figure 1.)
Predictive analytics is also an arcane set of techniques and technologies that bewilder many business and IT managers. It stirs together statistics, advanced mathematics, and artificial intelligence and adds a heavy dose of data management to create a potent brew that many would rather not drink! They don’t know if predictive analytics is a legitimate business endeavor or an ivory tower science experiment run wild.
Status of Predictive Analytics
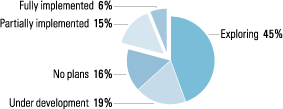
Figure 1. Predictive analytics is still in early-adopter phase.
Based on 833 respondents to a TDWI survey conducted in August 2006.
Where Do You Start? But once managers overcome their initial trepidation, they encounter another obstacle: how to apply predictive analytics optimally in their company. Most have only a vague notion about the business areas or applications that can benefit from predictive analytics. Second, most don’t know how to get started: whom to hire, how to organize the project, or how to architect the environment.
Definitions
Before we address those questions, it’s important to define what predictive analytics is and is not. Predictive analytics is a set of business intelligence (BI) technologies that uncovers relationships and patterns within large volumes of data that can be used to predict behavior and events. Unlike other BI technologies, predictive analytics is forward-looking, using past events to anticipate the future. (See Figure 2.)
The Spectrum of BI Technologies
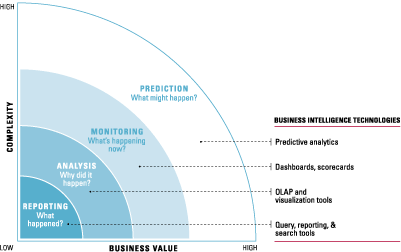
Figure 2. Among business intelligence disciplines, prediction provides the most business value but is also the most complex. Each discipline builds on the one below it—these are additive, not exclusive, in practice.
Applications. Predictive analytics can identify the customers most likely to churn next month or to respond to next week’s direct mail piece. It can also anticipate when factory floor machines are likely to break down or figure out which customers are likely to default on a bank loan. Today, marketing is the biggest user of predictive analytics with cross-selling, campaign management, customer acquisition, and budgeting and forecasting models top of the list, followed by attrition and loyalty applications. (See Figure 3.)
Applications for Predictive Analytics
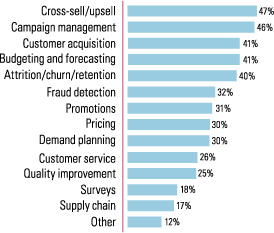
Figure 3. Based on 167 respondents who have implemented predictive analytics.
Respondents could select multiple answers.
Versus BI Tools. In contrast, other BI technologies—such as query and reporting tools, online analytical processing (OLAP), dashboards, and scorecards—examine what happened in the past. They are deductive in nature—that is, business users must have some sense of the patterns and relationships that exist within the data based on their personal experience. They use query, reporting, and OLAP tools to explore the data and validate their hypotheses. Dashboards and scorecards take deductive reasoning a step further: they present users with a de facto set of hypotheses in the form of metrics and key performance indicators (KPIs) that users examine on a regular basis.
Predictive analytics works the opposite way: it is inductive. It doesn’t presume anything about the data. Rather, predictive analytics lets data lead the way. Predictive analytics employs statistics, machine learning, neural computing, robotics, computational mathematics, and artificial intelligence techniques to explore all the data, instead of a narrow subset of it, to ferret out meaningful relationships and patterns. Predictive analytics is like an “intelligent” robot that rummages through all your data until it finds something interesting to show you.
No Silver Bullet. However, it’s important to note that predictive analytics is not a silver bullet. Practitioners have learned that most of the “intelligence” in these so-called decision automation systems comes from humans who have a deep understanding of the business and know where to point the tools, how to prepare the data, and how to interpret the results. Creating predictive models requires hard work, and the results are not guaranteed to provide any business value. For example, a model may predict that 75% of potential buyers of a new product are male, but if 75% of your existing customers are male, then this prediction doesn’t help the business. A marketing program targeting male shoppers will not yield any additional value or lift over a more generalized marketing program.
The Business Value of Predictive Analytics
Incremental Improvement. Although organizations occasionally make multi-million dollar discoveries using predictive analytics, these cases are the exception rather than the rule. Organizations that approach predictive analytics with a “strike-it-rich” mentality will likely become frustrated and give up before reaping any rewards. The reality is that predictive analytics provides incremental improvement to existing business processes, not million-dollar discoveries.
“We achieve success in little percentages,” says a technical lead for a predictive analytics team in a major telecommunications firm. She convinced her company several years ago to begin building predictive models to identify customers who might cancel their wireless phone service. “Our models have contributed to lowering our churn rate, giving us a competitive advantage.”
The company’s churn models expose insights about customer behavior that the business uses to improve marketing or reengineer business processes. For example, salespeople use model output to make special offers to customers at risk of churning, and the managers to change licensing policies that may be affecting churn rates.
Measuring Value. Our survey reinforces the business value of predictive analytics. Among respondents who have implemented predictive analytics, two-thirds (66%) say it provides “very high” or “high” business value. A quarter (27%) claim it provides moderate value and only 4% admit it provides “low” or “very low” value. (See Figure 4.)
What Is the Business Value of Predictive Analytics to Your Organization?
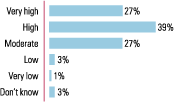
Figure 4. Based on 166 respondents who have implemented predictive analytics.
Respondents who selected “very high” or “high” in Figure 4 say they measure the success of their predictive analytics efforts with several criteria, starting with “meets business goals” (mentioned by 57% of respondents). Other success criteria include “model accuracy” (56%), “ROI” (40%), “lift” (35%), and “adoption rate by business users” (34%). (See Figure 5.)
How Do You Measure Success?
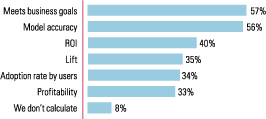
Figure 5. Based on 110 users who have implemented predictive analytics initiatives that offer “very high” or “high” value. Respondents could select multiple choices.
How Do You Deliver Predictive Analytics?
What Now? While some organizations have discovered the power of predictive analytics to reduce costs, increase revenues, and optimize business processes, the vast majority are still looking to get in the game. Today, most IT managers and some business managers understand the value that predictive analytics can bring, but most are perplexed about where to begin.
“We are sitting on a mountain of gold but we’re not mining it as effectively as we could,” says Michael Masciandaro, director of business intelligence at Rohm & Haas, a global specialty materials manufacturer. “We say we do analytics, but it’s really just reporting and OLAP.”
Rohm & Haas has hired consultants before to build pricing models that analyze and solve specific problems, but these models lose their usefulness once the consultants leave. Masciandaro says building an internal predictive analytics capability could yield tremendous insights and improve the profitability of key business areas, but he struggles to understand how to make this happen.
“How do you implement advanced analytics so they are not a one-off project done by an outside consultancy?” asks Masciandaro. “How do you bring this functionality in-house and use it to deliver value every day? And where do you find people who can do this? There are not too many of them out there.”
The Process of Predictive Modeling
Methodologies. Although most experts agree that predictive analytics requires great skill—and some go so far as to suggest that there is an artistic and highly creative side to creating models—most would never venture forth without a clear methodology to guide their work. In fact, process is so important that in 1996 several industry players created an industry standard methodology called the Cross Industry Standard Process for Data Mining (CRISP-DM).
Regardless of methodology, most processes for creating predictive models incorporate the following steps:
1. Project Definition: Define the business objectives and desired outcomes for the project and translate them into predictive analytic objectives and tasks.
2. Exploration: Analyze source data to determine the most appropriate data and model building approach, and scope the effort.
3. Data Preparation: Select, extract, and transform data upon which to create models.
4. Model Building: Create, test, and validate models, and evaluate whether they will meet project metrics and goals.
5. Deployment: Apply model results to business decisions or processes. This ranges from sharing insights with business users to embedding models into applications to automate decisions and business processes.
6. Model Management: Manage models to improve performance (i.e., accuracy), control access, promote reuse, standardize toolsets, and minimize redundant activities.
Most experts say the data preparation phase of creating predictive models is the most time-consuming part of the process, and our survey data agrees. On average, preparing the data occupies 25% of total project time. However, model creation, testing, and validation (23%) and data exploration (18%) are not far behind in the amount of project time they consume. This suggests that data preparation is no longer the obstacle it once was.
Recommendations
Now that we’ve defined predictive analytics, assessed its business value, and stepped through key trends and processes, it’s important to provide specific recommendations to BI managers and business sponsors about how to implement a predictive analytics practice. This section offers five recommendations that synthesize best practices from various organizations that have implemented predictive analytics.
1. Hire Business-Savvy Analysts to Create Models
Every interviewee for this report said the key to implementing a successful predictive analytics practice is to hire analytic modelers with deep understanding of the business, its core processes, and the data that drives those processes.
"Good analysts need strong knowledge of narrow business processes," says Keith Higdon of Sedgwick CMS. In claims processing, Higdon says good analysts "understand the business of claims handling; the interplay of variables across claim, claimant, and program characteristics; and what data they can and cannot rely on." Only then, he adds, can analysts create "meaningful models" that result in positive business outcomes.
2. Nurture a Rewarding Environment to Retain Analytic Modelers
Since good analytic modelers are difficult to find, it's imperative that managers create a challenging and rewarding environment. Of course, money is requisite. Top-flight analytic modelers often command higher salaries than classic business or data analysts. But good analytic modelers are motivated by things other than money and status, says Tom Breur, principal of XLNT Consulting in the Netherlands.
"You don't attract analytic modelers with the same incentives as other people," he says. "They want an opportunity to demonstrate their skills and learn new things, so you have to increase their training budgets. I've struggled with human resources departments on this issue."
3. Fold Predictive Analytics into the Information Management Team
The Inside Track. Traditionally, analytics teams are sequestered away in a back room somewhere and report to an ambitious department head (usually sales or marketing) who is seeking to ratchet up sales and get an edge on the competition. Unfortunately, this approach is not optimal, according to most practitioners. Analytic modelers are voracious consumers of data, and must establish strong alliances with the data warehousing team to maintain access to the data.
"Since I work in the data warehousing department within IT, I go to my colleagues and say, 'I need this,' and I usually get it. I get to the head of the queue faster than someone from outside, which means I get more [storage] space, quicker access to data, and more privileges. As a result, my projects get pushed faster. Those are the unwritten rules," says an analytic modeler.
4. Leverage the Data Warehouse to Prepare and Score the Data
Once you've hired the people and established the organization, the next important task is to provide a comprehensive solution for managing the data that the analytic modelers may want to use. While it is not necessary to build a data warehouse to support the analytic process, a data warehouse can make the process infinitely easier and faster.
Saving Time. A data warehouse pulls together all relevant information about one or more domains (e.g., customers, products, suppliers) from multiple operational systems and then integrates and standardizes this information so it can be queried and analyzed. With a data warehouse, analysts only have to query one source instead of multiple sources to get the data they need to build models.
5. Build Awareness and Confidence in the Technology
One of the toughest challenges in implementing analytics is convincing the business that this mathematical wizardry actually works. "Building confidence is a big challenge," says one analytics manager. "It takes a while before business people become confident enough in the models to apply the results. The ultimate litmus test is when business people are willing to embed models within operational processes and systems. That's when analytics goes mainstream in an organization."
But getting to a lights-out analytical environment is not easy. Most organizations, even those with large analytic staffs, still only apply analytics in pockets and have yet to truly operationalize the results.
Conclusion
Applying these five recommendations should enable any organization to implement predictive analytics with a good measure of success. While many people seem intimidated by predictive analytics because of its use of advanced mathematics and statistics, the technology and tools available today make it feasible for most organizations to reap value from predictive analytics.
Wayne W. Eckerson is the director of research and services for TDWI and author of "Performance Dashboards: Measuring, Monitoring, and Managing Your Business" (John Wiley & Sons, 2005). He can be reached at [email protected].
This article was excerpted from the full, 32-page report by the same name. You can download this and other TDWI Research free of charge at www.tdwi.org/research.
The report was sponsored by MicroStrategy, Inc., OutlookSoft Corporation, SAS, SPSS, Sybase, Inc., and Teradata, a division of NCR.
Next
Previous
Back to Table of Contents