Taking Data Quality to the Enterprise through Data Governance (Report Excerpt)
By Philip Russom, Senior Manager of Research and Services, TDWI
The Scope of Data-Quality Initiatives
Historically applied to isolated silos in departments or single databases, data quality is progressively applied with more breadth across enterprises. TDWI research shows that a surprisingly large number of organizations (39%) are already applying data quality in some form to the “whole enterprise” (see Figure 1). An additional 26% apply it in a “single department that spans the enterprise,” such as the IT and marketing departments. Meanwhile, low percentages continue the older tradition of applying data quality mostly in “a single department” (14%) or “a single business unit” (13%).
What is the scope of your data-quality initiative?
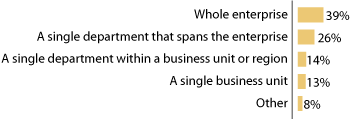
Figure 1. Based on 569 respondents (only those who have a data-quality initiative).
The gist of the market data is that many organizations are well down the road to enterprise-scope use of data-quality techniques and practices—enterprise data quality (EDQ). Users interviewed for this research reported similar progress, corroborating the survey data. But most interviewees quickly added that they had only recently arrived at EDQ, typically in 2003 or 2004. Hence, the data-quality marketplace and user community has only recently crossed the line into EDQ. TDWI suspects that many more organizations are on the cusp, and will cross into EDQ in coming years. Of course, occasional departmental usage will continue alongside EDQ.
One of the reasons data-quality usage is spreading is that it is often piggybacked atop related initiatives that carry it across the enterprise. Just about any data-intense initiative or software solution will ferret out data-quality problems and opportunities. IT and business sponsors have realized this over time, so it’s become commonplace to include a data-quality component in initiatives for governance (49%), CRM (42%), marketing campaigns (34%), compliance exercises (35%), and supply chain management (16%) (see Figure 2).
Which business initiatives does your data-quality initiative support?
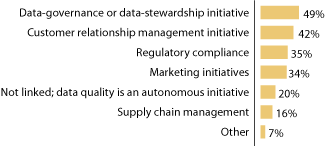
Figure 2. Based on 1153 responses from 569 respondents.
Anecdotal evidence suggests that data quality gives these initiatives better planning, a more predictable schedule, and a higher-quality deliverable. Likewise, dataquality software is often integrated with software for other solutions, like data warehousing and business intelligence (79%), customer data integration (38%), migrations and consolidations (35%), and master data management (35%) (see Figure 3).
Which software solutions does your data-quality initiative support?
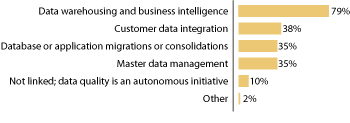
Figure 3. Based on 1137 responses from 569 respondents.
Most Data-Quality Trends Lead to Enterprise Use
The practice of data quality is in a state of transition, as its every aspect is currently evolving due to a strong trend. Most of these trends have a general effect in common—they result from or cause a broadened use of data-quality tools and practices across an enterprise (see Figure 4).
Data-quality products and practices are evolving quickly...
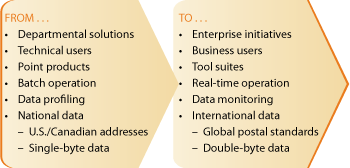
Figure 4. The evolution of data-quality products and practices.
From departmental solutions to enterprise initiatives. Most IT directors interviewed for this report spoke of their first data-quality projects as supporting data warehousing or marketing functions like direct mail. A few mentioned product data issues, like catalog matching and cleansing. Regardless of the isolated silos where they started, datamanagement professionals now pursue the quality of data broadly across their organizations. But many are at a fork in the road: either they keep deploying data-quality silos in more departments, or they fall back and regroup into a centralized team that gains efficiency and consistency across all efforts. TDWI recommends the second route, because it establishes a structure that leads to further progress in the long run.
From technical users to business users. Long story short, the data-quality user community gets more diverse all the time. At one extreme, technical users design large, scalable solutions and programs for matching and consolidation rules. At the other extreme, semi-technical marketers handle name-and-address cleansing and other customer data issues. With the rise of stewardship years ago and data governance recently, there’s a need to support business users who are process and domain experts, with little or no technical background. This trend affects the tools that vendors provide; most were designed for one of these user constituencies, and now must support them all. Likewise, organizations must staff their data-quality initiatives carefully to address all these user types, their needs, and their unique contributions.
From point products to tool suites. TDWI defines data quality as a collection of many practices, which explains why few vendors offer a single “data-quality tool.” Instead, most offer multiple tools, each automating a specific data-quality task like list scrubbing, fuzzy matching, geo-coding, and so on. As organizations broaden data-quality usage, they use more of these point products, then suffer the lack of interoperability, collaboration, and reuse among them. To supply this demand, vendors have worked hard to integrate their point products into cohesive suites.
From batch to real-time operation. Some data-quality tasks are still best done in batch, like list scrubbing and matching records in large datasets. However, given that data entry is the leading source of garbage data, there’s a real need for real-time verification, cleansing, and enhancement of data before it enters an application database. As with most data-management practices, data-quality software has evolved to support various speeds of “right-time” processing.
From data profiling to data monitoring. These are similar in that each results in an assessment of the quality of data. On the one hand, data profiling has the additional step of data discovery and is done deepest prior to designing a dataquality solution. Monitoring, on the other hand, is about measuring the quality of data frequently while a data-quality solution progresses, so stewards and others can make tactical adjustments to keep a quality initiative on plan. The trend is to do profiling more deeply, then embrace monitoring eventually. TDWI strongly recommends both.
From national to international data. Companies that are multi-national or have a multi-national customer base have special problems when expanding data-quality efforts across a global enterprise. Name-and-address cleansing is a straightforward task when done for U.S. and Canadian addresses and postal standards; yet it becomes quite complex as you add more languages, national postal standards, and information structures (like Unicode pages and double-byte data). Users must deal with these and other issues as they take data quality to an international enterprise.
Data Governance and Enterprise Data Quality
TDWI data shows that many organizations are practicing enterprise data quality in some sense. The catch, however, is that practices from isolated areas (like data warehousing or marketing campaigns) aren’t automatically successful on an enterprise scale. Accomplishing anything at the enterprise level requires close cooperation among IT and business professionals who understand the data and its business purpose and have a mandate for change. To achieve this, an organization can establish a datagovernance committee according to the following definition:
When an organization views data as an enterprise asset (transcending the data warehouse and spanning the whole organization), it establishes an executive-level data-governance committee that oversees data stewardship across the organization. Depending on the scope of a datagovernance initiative, it may guide related initiatives, like data quality, data architecture, data integration, data warehousing, metadata management, master data management, and so on.
Distinctions between stewardship and governance are thin in some cases. But TDWI sees data stewardship as a local task that protects and nourishes specific data collections for specific purposes (like a data warehouse for business intelligence or marketing databases for direct mail). Data governance is a larger undertaking that exerts control over multiple business initiatives and technology implementations, to unify these through consistent data definitions and gain greater reuse for IT projects and business efforts. The two can work together, in that a data-governance committee can be a management level that coordinates multiple data-stewardship teams. In a few companies, data governance is a subset of an even larger corporate governance initiative.
The most critical success factor with governance is mandate. Governance bodies and stewards must exert change on business and technical people—who own the data and its processes—when opportunities for improvement arise. The most effective mandates come from a high-level executive. Without a strong mandate for change and an attentive executive sponsor, stewardship and governance deteriorate into academic data profiling exercises with little or no practical application.
The State of Data-Governance Initiatives
TDWI’s data-quality surveys asked: “Who is responsible for data quality in your organization?” In both 2001 and 2005, the data warehouse team and IT bubbled to the top of the list (47% and 43% in Figure 5). This makes sense from a technology viewpoint, in that these are the technical people long involved in data quality. But it gives technology priority over business, whereas the two must collaborate in a stewardship or governance program. Respondents ranked business analysts and power users in third place (30%), followed by the “cross-functional team from business and IT” (28%), a description that includes both sides, as in our definition of data governance. So, respondents recognize that responsibility for the quality of data must be shared by some kind of cross-functional team. But the fact that “data-quality analysts” and “data stewards” ranked even lower than “front-line workers” (whose data entry is the leading cause of garbage data), indicates that sharing responsibility through governance and stewardship is still rare.
Who is responsible for data quality in your organization?
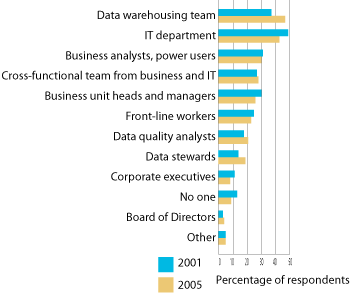
Figure 5. Based on 1701 responses from 647 respondents in 2001, 1957 responses from 750 respondents in 2005.
When asked about data-governance initiatives, a disappointing 8% reported having deployed one, while 42% have “no plans” (see Figure 6). TDWI then asked respondents (except those with “no plans”) to rank the effectiveness of the steering committees, degree of executive involvement, and usefulness of policies and processes in their data-governance initiative. The majority ranked all three areas as “moderate,” meaning there’s plenty of room for improvement.
What's the status of your organization's data-governance initiative?
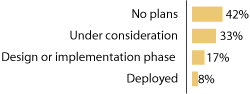
Figure 6. Based on 750 respondents in 2005.
These disappointing responses are most likely due to data governance being a relatively new approach, coupled with the fact that many organizations seem to be on the cusp—they’ve stretched data-quality practices over the enterprise in a disconnected way and now it’s time to control them to ensure consistency and efficiency, whether the control is via stewardship, governance, or centralized IT services. Various forms of data governance will, no doubt, disseminate as more organizations come off the cusp.
Anatomy of Data-Governance and Stewardship Programs
Staffing and management hierarchy for data-governance and stewardship programs will vary according to each organization’s unique structure and needs. The following description—a composite drawn from multiple interviewees—illustrates the requisite parts:
- A domain steward is assigned per business unit. Domain stewards work directly with the line-of-business manager who owns the data and the IT manager who administers it. Each steward has a mandate to change the process and structure of any business, person, or IT system, if that’s what it takes to improve data. Note that most changes proposed should be business oriented, with business value as a goal of any data-quality work that gets done. Without demonstrable value, it’s unlikely the work will get approved or done.
- A corporate steward manages a group of domain stewards. This management hierarchy helps related domain stewards collaborate. And the corporate steward provides domain stewards with additional clout to help domain stewards enforce their mandates.
- A governance committee consists of miscellaneous managers. These include corporate stewards, corporate sponsors (CxOs and SVPs), and miscellaneous IT and line-of-business managers, as needed. This committee sets top-down strategic goals, coordinates efforts, and provides common definitions, rules and standards, which apply to data structures, access, and use across the entire enterprise.
Figure 7 shows how the layers of stewardship and management may roll up into a data-governance committee. Dark arrows represent direct reports, while gray arrows represent significant interactions outside the reporting structure of the organization.
Possible Organizational and Report Structure for Data Governance
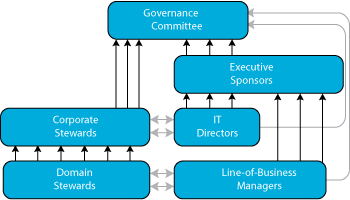
Figure 7. Stewardship and management roll up into data governance.
Conclusions and Recommendations
Data quality proved itself in its data warehouse and direct mail origins, and has now moved beyond these into enterprise data quality, where it is applied in many departments for many purposes. In fact, most trends result from or cause a broadened use of data quality tools and practices across the enterprise. Plus, data quality is now de rigueur as a component of various business initiatives and software solutions. While this broadening is good for the data, it’s challenging for the organization, which must adjust its business processes and IT org chart to adapt.
Get ready for enterprise data quality. It will improve many business and technical processes, if you’re open to its diversity and give it necessary organizational structure.
- Embrace the diversity of dataquality practices. Many organizations need to move beyond name-and-address cleansing, data warehouse enhancement, and product catalog record matching. These are useful applications, but are narrow in scope. The lessons learned and skills developed for these can be leveraged in other data-quality applications across the enterprise.
- Address enterprise data quality. The data-quality initiatives of 39% of survey respondents already address the “whole enterprise.” Follow their lead into enterprise usage, but resist the urge to deploy data quality in isolated pockets of software tools and IT personnel. Some kind of centralization can improve personnel allocation, project reuse, and data consistency.
- Give EDQ required organizational structure through data governance. EDQ's chances of large-scale, long-term success are limited without a support organization, whether its form is a data governance committee, a data stewardship program, or a data quality center of excellence. Another key requirement is a strong mandate supported by a prominent executive sponsor.
Philip Russom is TDWI's senior manager of research and services. Before joining TDWI in 2005, Russom was an industry analyst covering data warehousing, business intelligence, and integration technologies at Forrester Research, Giga Information Group, and Hurwitz Group. He also ran his own business as an independent industry analyst and consultant, and was contributing editor with Intelligent Enterprise and DM Review magazines. You can reach him at [email protected].
Excerpted from the full April 2006 report. TDWI appreciates the sponsorship of Business Objects, Collaborative Consulting, DataFlux, DataLever, Firstlogic, IBM, Informatica, Similarity Systems, and Trillium Software.
To download the full report, visit www.tdwi.org/research/reportseries.
Next
Previous
Back to Table of Contents